Multivariate Bayesian clustering using covariate-informed components with application to boreal vegetation sensitivity.
Biometrics(2022)
摘要
Climate change is impacting both the distribution and abundance of vegetation, especially in far northern latitudes. The effects of climate change are different for every plant assemblage and vary heterogeneously in both space and time. Small changes in climate could result in large vegetation responses in sensitive assemblages but weak responses in robust assemblages. But, patterns and mechanisms of sensitivity and robustness are not yet well understood, largely due to a lack of long-term measurements of climate and vegetation. Fortunately, observations are sometimes available across a broad spatial extent. We develop a novel statistical model for a multivariate response based on unknown cluster-specific effects and covariances, where cluster labels correspond to sensitivity and robustness. Our approach utilizes a prototype model for cluster membership that offers flexibility while enforcing smoothness in cluster probabilities across sites with similar characteristics. We demonstrate our approach with an application to vegetation abundance in Alaska, USA, in which we leverage the broad spatial extent of the study area as a proxy for unrecorded historical observations. In the context of the application, our approach yields interpretable site-level cluster labels associated with assemblage-level sensitivity and robustness without requiring strong a priori assumptions about the drivers of climate sensitivity.
更多查看译文
关键词
Alaska national parks,climate change,clustering regression coefficients,community ecology,joint distribution models
AI 理解论文
溯源树
样例
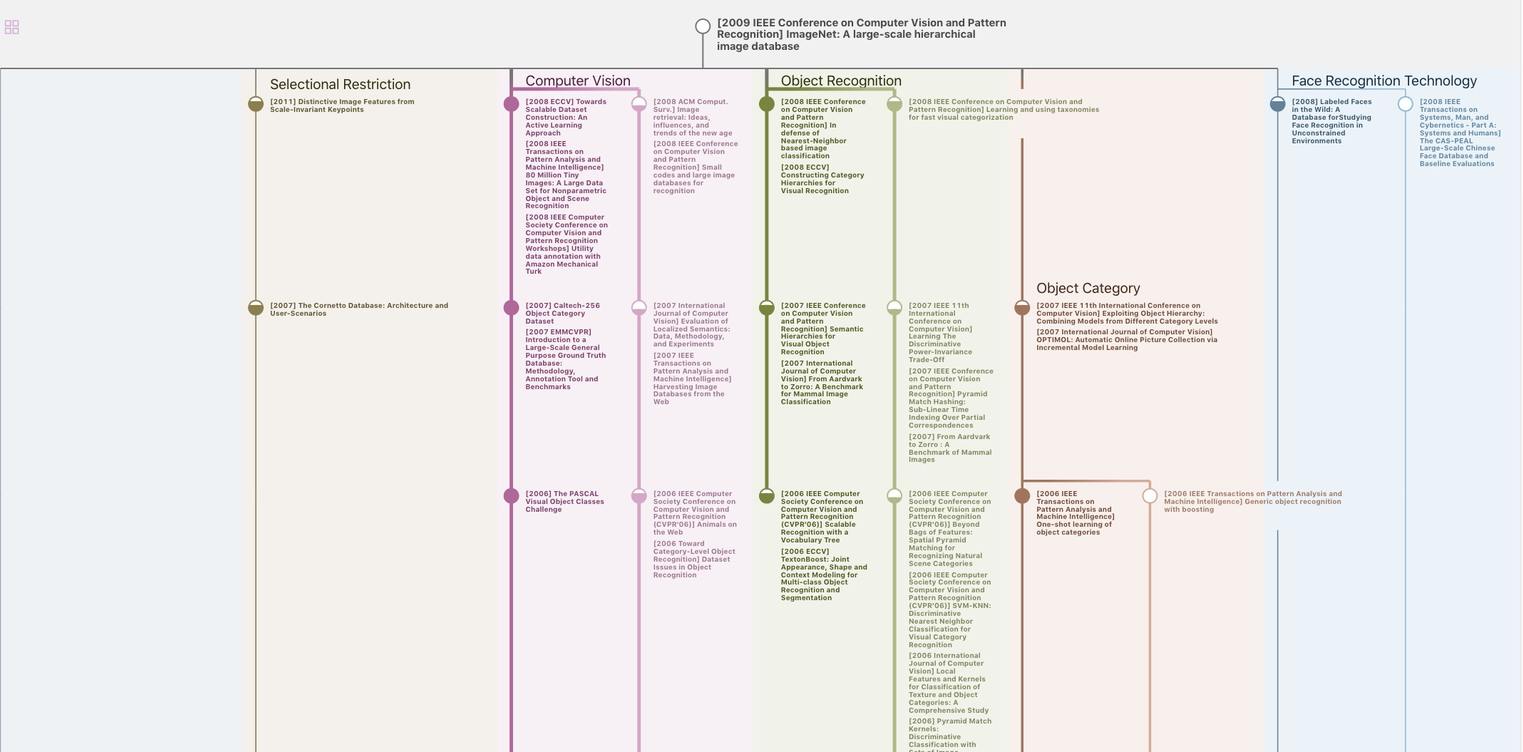
生成溯源树,研究论文发展脉络
Chat Paper
正在生成论文摘要