Anomaly Detection for Mixed Packet Sequences
2020 IEEE 45th LCN Symposium on Emerging Topics in Networking (LCN Symposium)(2020)
摘要
One-Dimensional Convolutional Neural Networks (1-DCNNs) have shown an admirable success in Natural Language Processing (NLP). Inspired by the capabilities of such approaches to overcome challenges related to sequence order, we present a 1-DCNN-based Intrusion Detection System (IDS) for attack detection in network traffic. Our proposal is capable of classifying mixed packet sequences without flow aggregation, thus reducing computational efforts. In addition, we prove that learning attack classes in an incremental manner and coping with the emergence of new patterns in a permanent implementation is feasible. We obtain comparable detection performance to other classification techniques, but with the outstanding achievement of being able to isolate malicious communications based on explainability analysis even for traffic with a comprehensive encryption.
更多查看译文
关键词
networking,encryption,convolutional neural networks,incremental learning
AI 理解论文
溯源树
样例
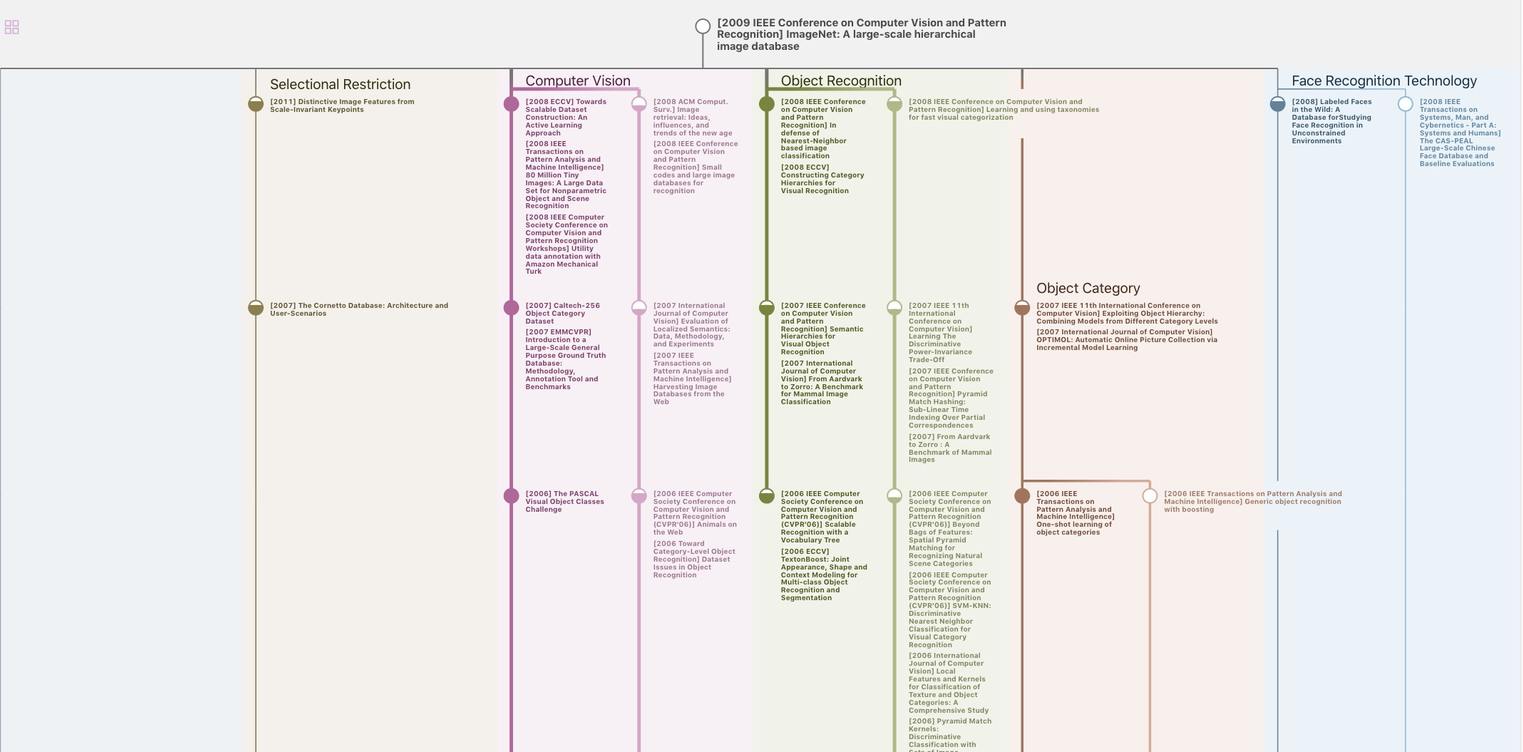
生成溯源树,研究论文发展脉络
Chat Paper
正在生成论文摘要