Domain adaptation of object detector using scissor-like networks
Neurocomputing(2021)
摘要
When the training data and the test data do not obey the same distribution, the performances of many object detection methods always decrease greatly. Naturally, domain adaptation methods at feature level are proposed. The basic idea is to adapt the feature extraction network such that the feature distributions of the source and target domains match. We propose a new method that is built directly on the Faster R-CNN model, which not only aligns the source and target data features, but also forces their generated features closer together to further align the source and target domains. Moreover, compared with previous approaches, we construct a more powerful discriminator and a simple generator to solve the domain adaptation problem. The model works like a pair of scissors, so we call it Scissors Networks (SN). We conduct extensive experiments on popular datasets, including Cityscapes, Foggy Cityscapes, SIM10k and KITTI. The experimental results demonstrate that our algorithm is superior to the state-of-the-art deep learning based domain adaptation approaches.
更多查看译文
关键词
Object detection,Domain adaptation,Feature distribution,Transfer learning
AI 理解论文
溯源树
样例
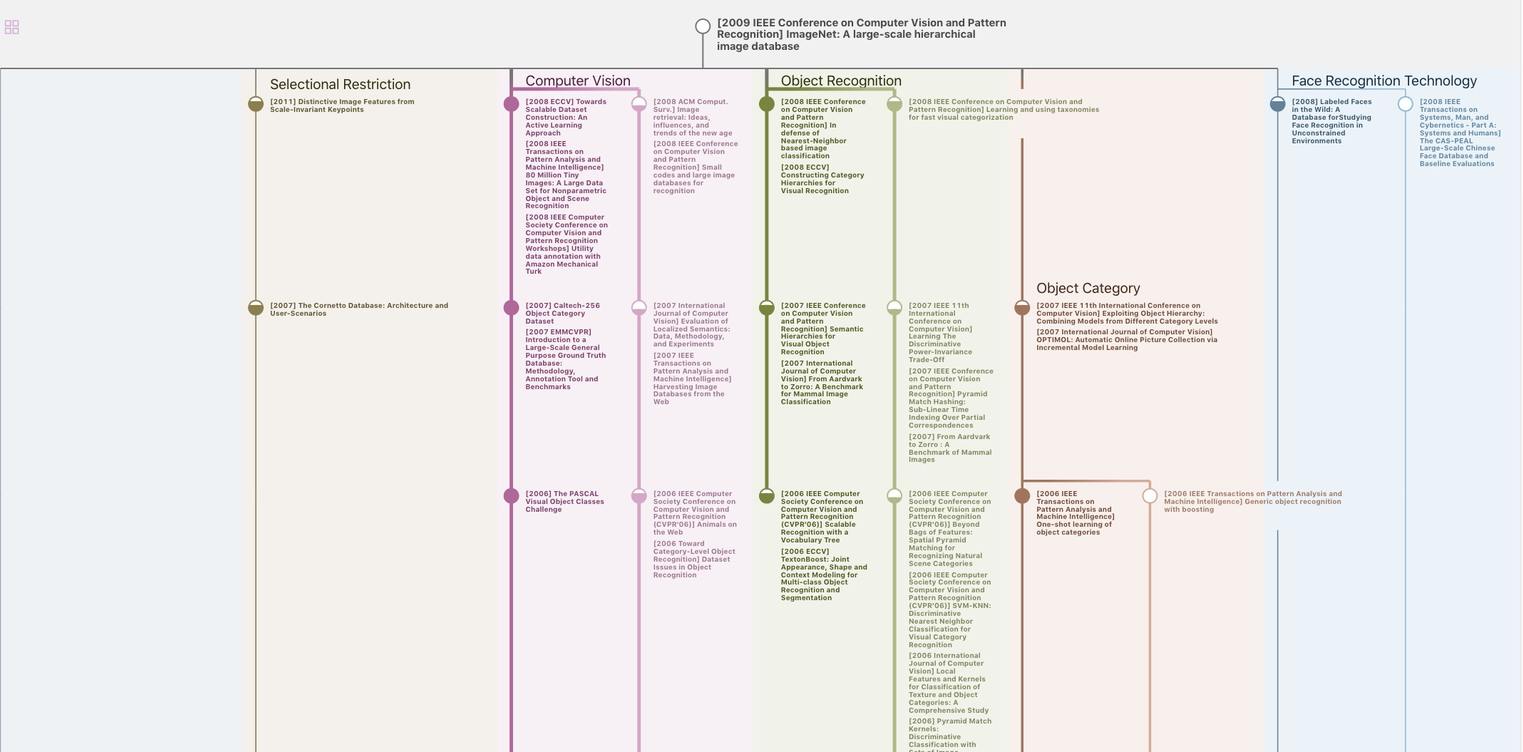
生成溯源树,研究论文发展脉络
Chat Paper
正在生成论文摘要