Collaborative Training of Acoustic Encoders for Speech Recognition.
Interspeech(2021)
摘要
On-device speech recognition requires training models of different sizes for deploying on devices with various computational budgets. When building such different models, we can benefit from training them jointly to take advantage of the knowledge shared between them. Joint training is also efficient since it reduces the redundancy in the training procedure's data handling operations. We propose a method for collaboratively training acoustic encoders of different sizes for speech recognition. We use a sequence transducer setup where different acoustic encoders share a common predictor and joiner modules. The acoustic encoders are also trained using co-distillation through an auxiliary task for frame level chenone prediction, along with the transducer loss. We perform experiments using the LibriSpeech corpus and demonstrate that the collaboratively trained acoustic encoders can provide up to a 11% relative improvement in the word error rate on both the test partitions.
更多查看译文
关键词
speech recognition,knowledge distillation,co-distillation,collaborative training,transformer
AI 理解论文
溯源树
样例
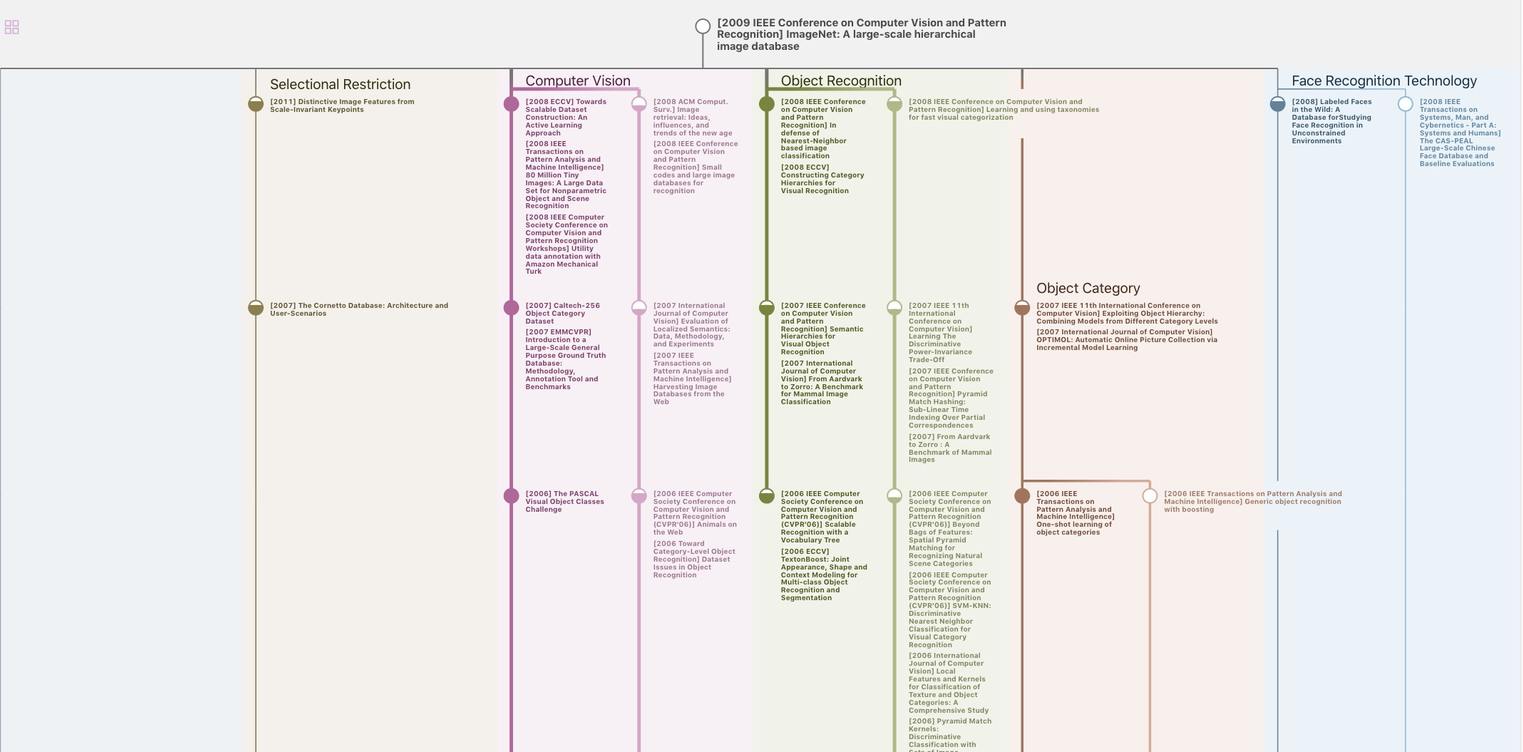
生成溯源树,研究论文发展脉络
Chat Paper
正在生成论文摘要