RefBERT: Compressing BERT by Referencing to Pre-computed Representations
2021 INTERNATIONAL JOINT CONFERENCE ON NEURAL NETWORKS (IJCNN)(2021)
Abstract
Recently developed large pre-trained language models, e.g., BERT, have achieved remarkable performance in many downstream natural language processing applications. These pretrained language models often contain hundreds of millions of parameters and suffer from high computation and latency in real-world applications. It is desirable to reduce the computation overhead of the models for fast training and inference while keeping the model performance in downstream applications. Several lines of work utilize knowledge distillation to compress the teacher model to a smaller student model. However, they usually discard the teacher's knowledge when in inference. Differently, in this paper, we propose RefBERT to leverage the knowledge learned from the teacher, i.e., facilitating the pre-computed BERT representation on the reference sample and compressing BERT into a smaller student model. To guarantee our proposal, we provide theoretical justification on the loss function and the usage of reference samples. Significantly, the theoretical result shows that including the pre-computed teacher's representations on the reference samples indeed increases the mutual information in learning the student model. Finally, we conduct the empirical evaluation and show that our RefBERT can beat the vanilla TinyBERT over 8.1% and achieves more than 94% of the performance of BERTBASE on the GLUE benchmark. Meanwhile, RefBERT is 7.4x smaller and 9.5x faster on inference than BERTBASE.
MoreTranslated text
Key words
BERT, knowledge distillation, reference, mutual information
AI Read Science
Must-Reading Tree
Example
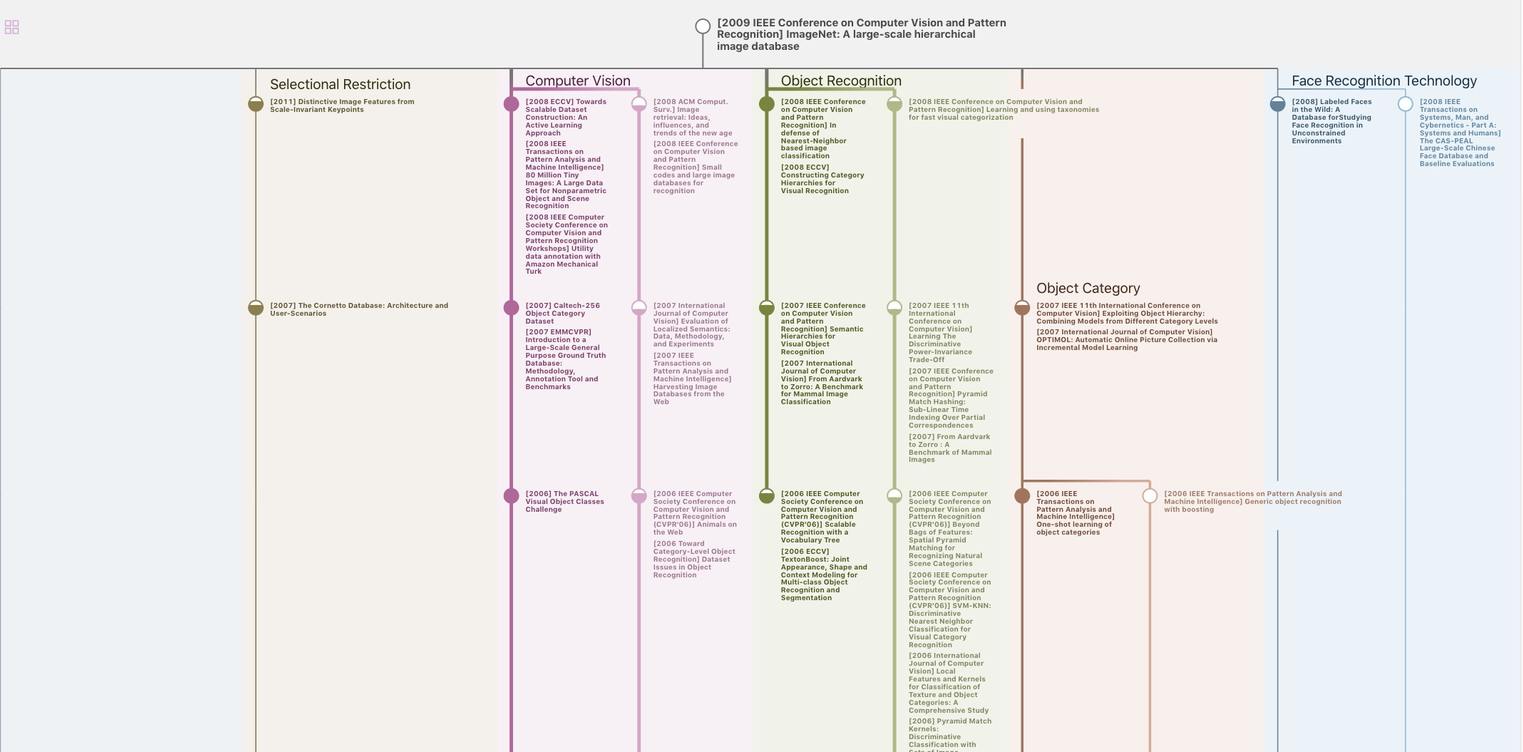
Generate MRT to find the research sequence of this paper
Chat Paper
Summary is being generated by the instructions you defined