Scalable Quasi-Bayesian Inference for Instrumental Variable Regression.
Annual Conference on Neural Information Processing Systems(2021)
摘要
Recent years have witnessed an upsurge of interest in employing flexible machine learning models for instrumental variable (IV) regression, but the development of uncertainty quantification methodology is still lacking. In this work we present a scalable quasi-Bayesian procedure for IV regression, building upon the recently developed kernelized IV models. Contrary to Bayesian modeling for IV, our approach does not require additional assumptions on the data generating process, and leads to a scalable approximate inference algorithm with time cost comparable to the corresponding point estimation methods. Our algorithm can be further extended to work with neural network models. We analyze the theoretical properties of the proposed quasi-posterior, and demonstrate through empirical evaluation the competitive performance of our method.
更多查看译文
关键词
regression,quasi-bayesian
AI 理解论文
溯源树
样例
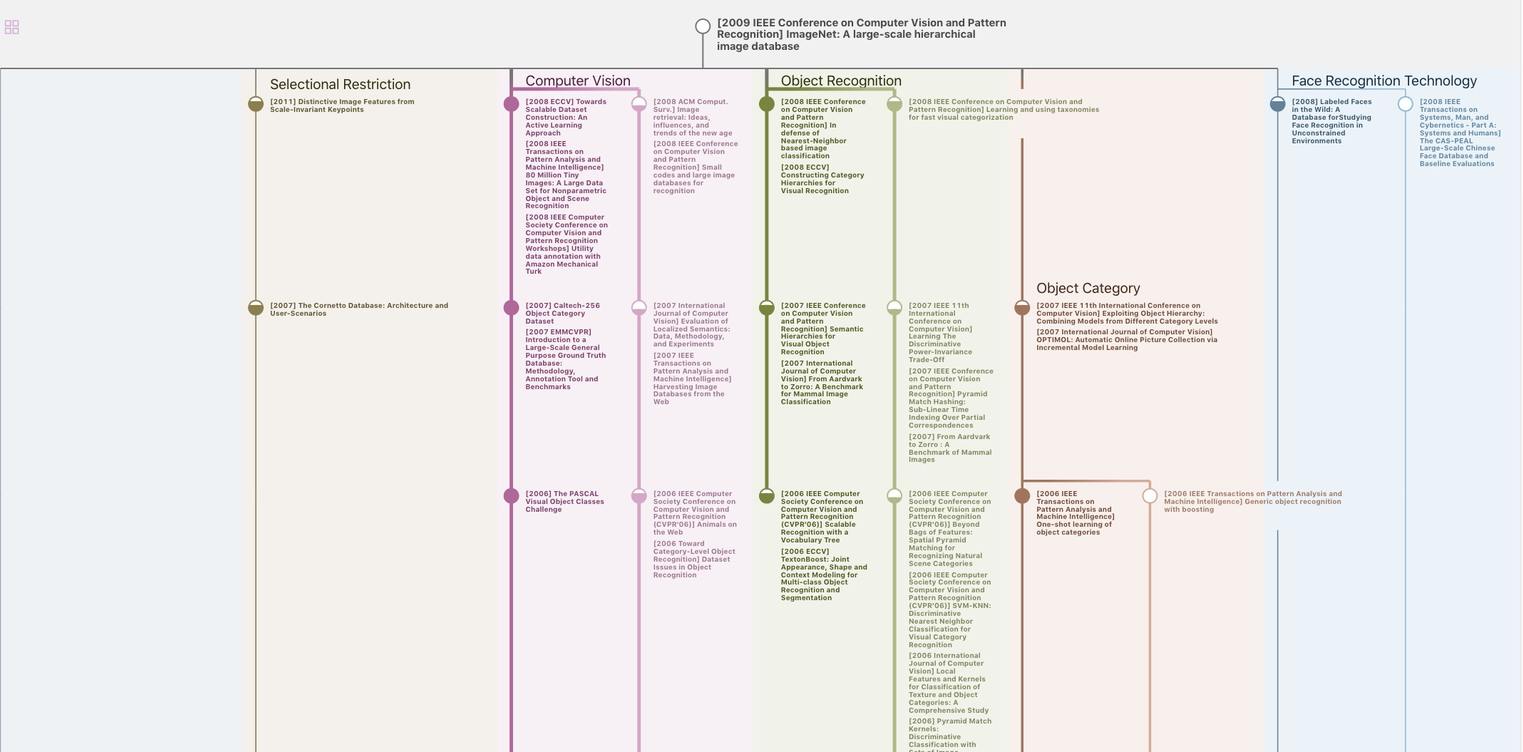
生成溯源树,研究论文发展脉络
Chat Paper
正在生成论文摘要