Retraction-based first-order feasible methods for difference-of-convex programs with smooth inequality and simple geometric constraints
arxiv(2023)
摘要
In this paper, we propose first-order feasible methods for difference-of-convex (DC) programs with smooth inequality and simple geometric constraints. Our strategy for maintaining feasibility of the iterates is based on a “retraction” idea adapted from the literature of manifold optimization. When the constraints are convex, we establish the global subsequential convergence of the sequence generated by our algorithm under strict feasibility condition, and analyze its convergence rate when the objective is in addition convex according to the Kurdyka-Łojasiewicz (KL) exponent of the extended objective (i.e., sum of the objective and the indicator function of the constraint set). We also show that the extended objective of a large class of Euclidean norm (and more generally, group LASSO penalty) regularized convex optimization problems is a KL function with exponent 1/2 ; consequently, our algorithm is locally linearly convergent when applied to these problems. We then extend our method to solve DC programs with a single specially structured nonconvex constraint. Finally, we discuss how our algorithms can be applied to solve two concrete optimization problems, namely, group-structured compressed sensing problems with Gaussian measurement noise and compressed sensing problems with Cauchy measurement noise, and illustrate the empirical performance of our algorithms.
更多查看译文
关键词
First-order feasible methods,Retraction,Difference-of-convex optimization,Kurdyka-Lojasiewicz exponents
AI 理解论文
溯源树
样例
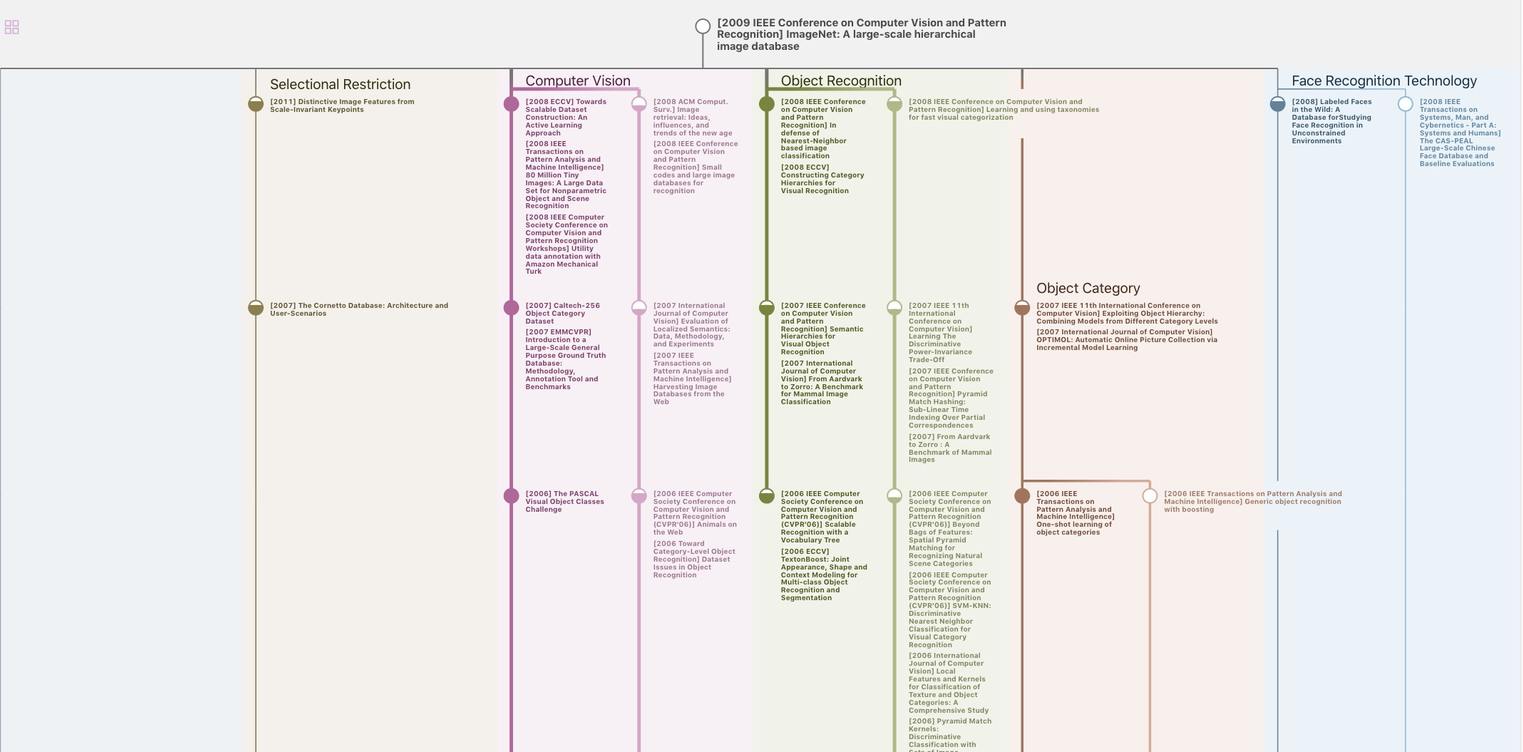
生成溯源树,研究论文发展脉络
Chat Paper
正在生成论文摘要