SAU-Net: A Unified Network for Cell Counting in 2D and 3D Microscopy Images
IEEE/ACM Transactions on Computational Biology and Bioinformatics(2022)
摘要
Image-based cell counting is a fundamental yet challenging task with wide applications in biological research. In this paper, we propose a novel unified deep network framework designed to solve this problem for various cell types in both 2D and 3D images. Specifically, we first propose SAU-Net for cell counting by extending the segmentation network U-Net with a Self-Attention module. Second, we design an extension of Batch Normalization (BN) to facilitate the training process for small datasets. In addition, a new 3D benchmark dataset based on the existing mouse blastocyst (MBC) dataset is developed and released to the community. Our SAU-Net achieves state-of-the-art results on four benchmark 2D datasets - synthetic fluorescence microscopy (VGG) dataset, Modified Bone Marrow (MBM) dataset, human subcutaneous adipose tissue (ADI) dataset, and Dublin Cell Counting (DCC) dataset, and the new 3D dataset, MBC. The BN extension is validated using extensive experiments on the 2D datasets, since GPU memory constraints preclude use of 3D datasets. The source code is available at
https://github.com/mzlr/sau-net
.
更多查看译文
关键词
Animals,Attention,Humans,Image Processing, Computer-Assisted,Imaging, Three-Dimensional,Mice,Microscopy
AI 理解论文
溯源树
样例
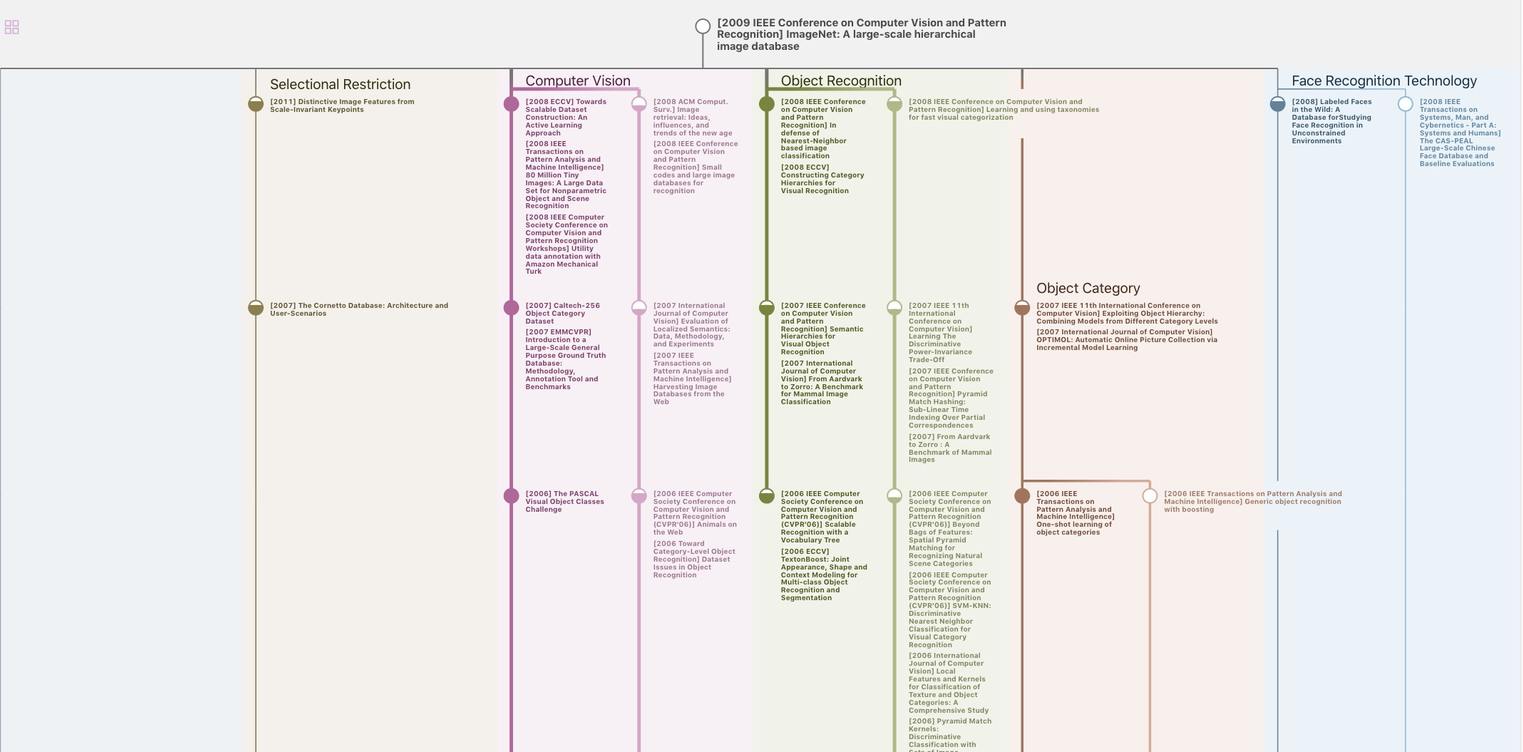
生成溯源树,研究论文发展脉络
Chat Paper
正在生成论文摘要