Revisiting Superpixels for Active Learning in Semantic Segmentation with Realistic Annotation Costs
2021 IEEE/CVF CONFERENCE ON COMPUTER VISION AND PATTERN RECOGNITION, CVPR 2021(2021)
摘要
State-of-the-art methods for semantic segmentation are based on deep neural networks that are known to be data-hungry. Region-based active learning has shown to be a promising method for reducing data annotation costs. A key design choice for region-based AL is whether to use regularly-shaped regions (e.g., rectangles) or irregularly-shaped region (e.g., superpixels). In this work, we address this question under realistic, click-based measurement of annotation costs. In particular, we revisit the use of super-pixels and demonstrate that the inappropriate choice of cost measure (e.g., the percentage of labeled pixels), may cause the effectiveness of the superpixel-based approach to be under-estimated. We benchmark the superpixel-based approach against the traditional "rectangle+polygon"-based approach with annotation cost measured in clicks, and show that the former outperforms on both Cityscapes and PASCAL VOC. We further propose a class-balanced acquisition function to boost the performance of the superpixel-based approach and demonstrate its effectiveness on the evaluation datasets. Our results strongly argue for the use of superpixel-based AL for semantic segmentation and highlight the importance of using realistic annotation costs in evaluating such methods.
更多查看译文
关键词
semantic segmentation,realistic annotation costs,deep neural networks,region-based active learning,data annotation costs,region-based AL,irregularly-shaped region,click-based measurement,cost measure,superpixel-based AL,Cityscapes,PASCAL VOC,rectangle+polygon-based approach
AI 理解论文
溯源树
样例
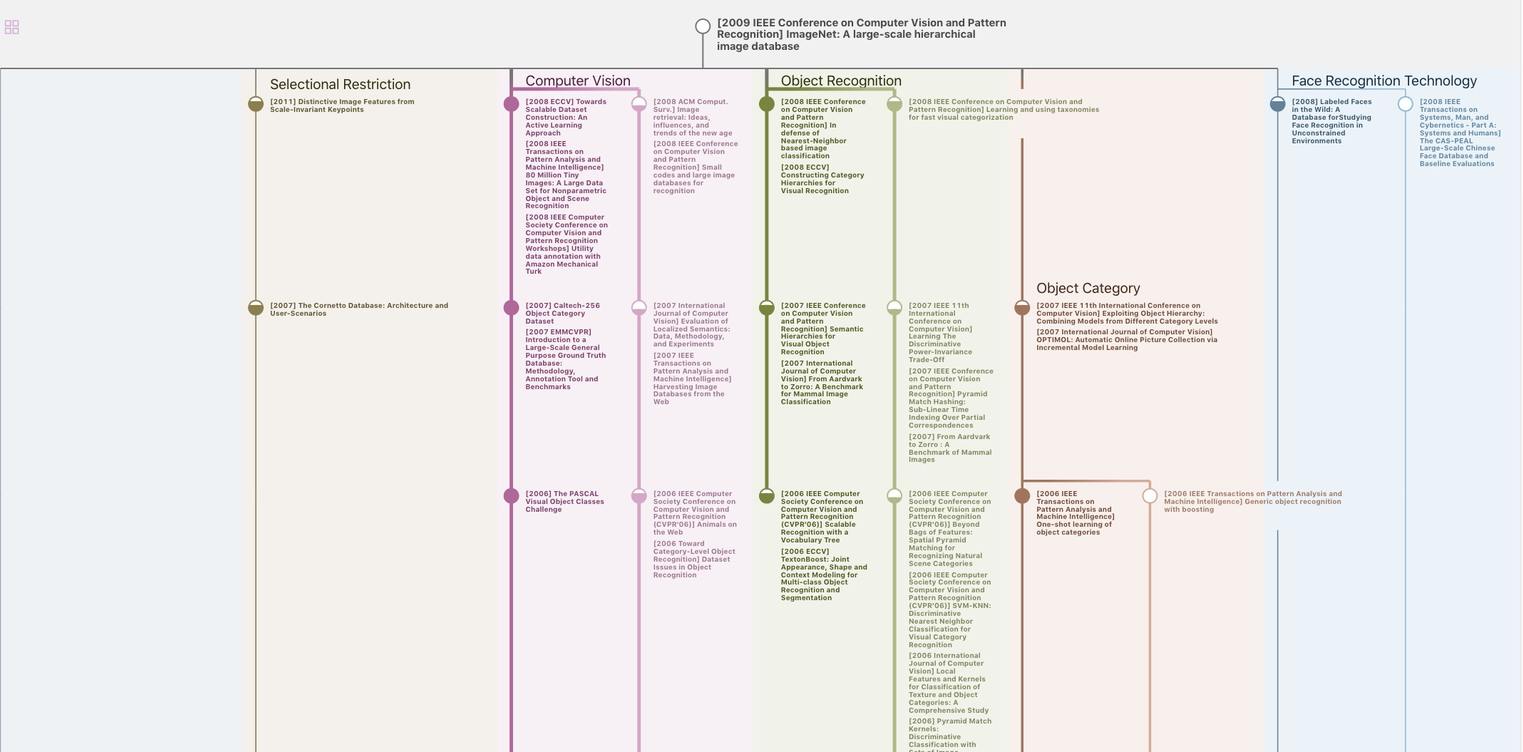
生成溯源树,研究论文发展脉络
Chat Paper
正在生成论文摘要