PointNetLK Revisited
Proceedings of the IEEE/CVF Conference on Computer Vision and Pattern Recognition (CVPR)(2021)
摘要
We address the generalization ability of recent learning-based point cloud registration methods. Despite their success, these approaches tend to have poor performance when applied to mismatched conditions that are not well-represented in the training set, such as unseen object categories, different complex scenes, or unknown depth sensors. In these circumstances, it has often been better to rely on classical non-learning methods (e.g., Iterative Closest Point), which have better generalization ability. Hybrid learning methods, that use learning for predicting point correspondences and then a deterministic step for alignment, have offered some respite, but are still limited in their generalization abilities. We revisit a recent innovation— PointNetLK [1]—and show that the inclusion of an analytical Jacobian can exhibit remarkable generalization properties while reaping the inherent fidelity benefits of a learning framework. Our approach not only outperforms the state-of-the-art in mismatched conditions but also produces results competitive with current learning methods when operating on real-world test data close to the training set.
更多查看译文
关键词
PointNetLK revisited,learning-based point cloud registration methods,mismatched conditions,training set,unseen object categories,complex scenes,unknown depth sensors,Iterative Closest Point,hybrid learning methods,point correspondences,generalization properties,learning framework,classical nonlearning methods,analytical Jacobian inclusion,real-world test data
AI 理解论文
溯源树
样例
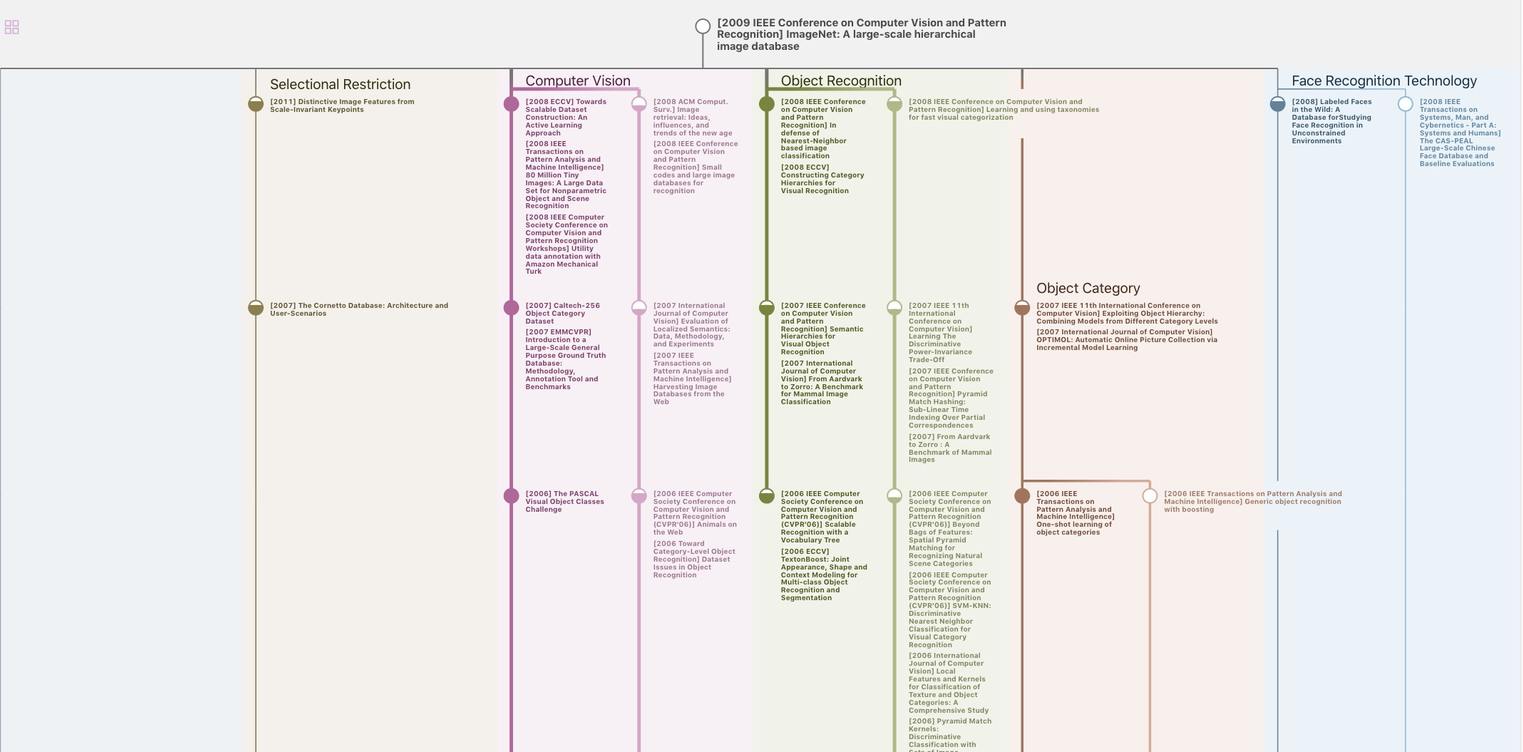
生成溯源树,研究论文发展脉络
Chat Paper
正在生成论文摘要