RSN: Range Sparse Net for Efficient, Accurate LiDAR 3D Object Detection
2021 IEEE/CVF CONFERENCE ON COMPUTER VISION AND PATTERN RECOGNITION, CVPR 2021(2021)
Abstract
The detection of 3D objects from LiDAR data is a critical component in most autonomous driving systems. Safe, high speed driving needs larger detection ranges, which are enabled by new LiDARs. These larger detection ranges require more efficient and accurate detection models. Towards this goal, we propose Range Sparse Net (RSN) - a simple, efficient, and accurate 3D object detector - in order to tackle real time 3D object detection in this extended detection regime. RSN predicts foreground points from range images and applies sparse convolutions on the selected foreground points to detect objects. The lightweight 2D convolutions on dense range images results in significantly fewer selected foreground points, thus enabling the later sparse convolutions in RSN to efficiently operate. Combining features from the range image further enhance detection accuracy. RSN runs at more than 60 frames per second on a 150m x 150m detection region on Waymo Open Dataset (WOD) while being more accurate than previously published detectors. As of 11/2020, RSN is ranked first in the WOD leaderboard based on the APH/LEVEL_1 metrics for LiDAR-based pedestrian and vehicle detection, while being several times faster than alternatives.
MoreTranslated text
Key words
RSN,Range Sparse Net,LiDAR 3D object detection,LiDAR data,autonomous driving systems,3D object detector,extended detection regime,sparse convolutions,lightweight 2D convolutions,dense range images results,vehicle detection,real time 3D object detection,Waymo Open Dataset,WOD
AI Read Science
Must-Reading Tree
Example
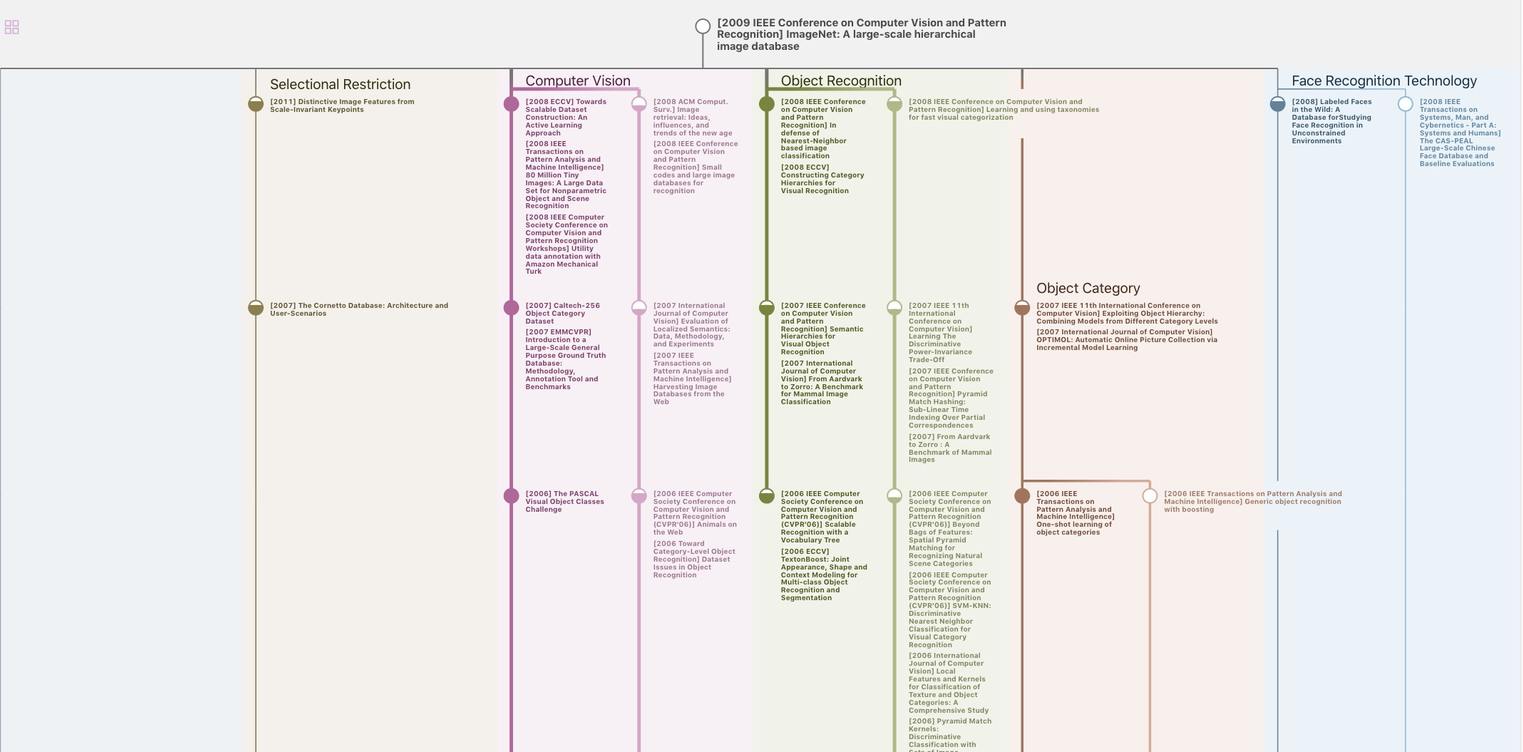
Generate MRT to find the research sequence of this paper
Chat Paper
Summary is being generated by the instructions you defined