LPSNet: A lightweight solution for fast panoptic segmentation
2021 IEEE/CVF CONFERENCE ON COMPUTER VISION AND PATTERN RECOGNITION, CVPR 2021(2021)
摘要
Panoptic segmentation is a challenging task aiming to simultaneously segment objects (things) at instance level and background contents (stuff) at semantic level. Existing methods mostly utilize a two-stage detection network to attain instance segmentation results, and a fully convolutional network to produce a semantic segmentation prediction. Post-processing or additional modules are required to handle the conflicts between the outputs from these two nets, which makes such methods suffer from low efficiency, heavy memory consumption and complicated implementation. To simplify the pipeline and decrease computation/memory cost, we propose an one-stage approach called Lightweight Panoptic Segmentation Network (LPSNet), which does not involve a proposal, anchor or mask head. Instead, we predict a bounding box and semantic category at each pixel upon the feature map produced by an augmented feature pyramid, and design a parameter-free head to merge the per-pixel bounding box and semantic prediction into panoptic segmentation output. Our LPSNet is not only efficient in computation and memory, but also accurate in panoptic segmentation. Comprehensive experiments on COCO, Cityscapes and Mapillary Vistas datasets demonstrate the promising effectiveness and efficiency of the proposed LP-SNet.
更多查看译文
关键词
per-pixel bounding box prediction,COCO datasets,Cityscape datasets,Mapillary Vistas datasets,augmented feature pyramid,parameter-free head design,feature map,background contents,instance segmentation,panoptic segmentation output,semantic prediction,semantic category,Lightweight Panoptic Segmentation Network,one-stage approach,heavy memory consumption,semantic segmentation prediction,fully convolutional network,two-stage detection network,semantic level,instance level,fast panoptic segmentation,LPSNet
AI 理解论文
溯源树
样例
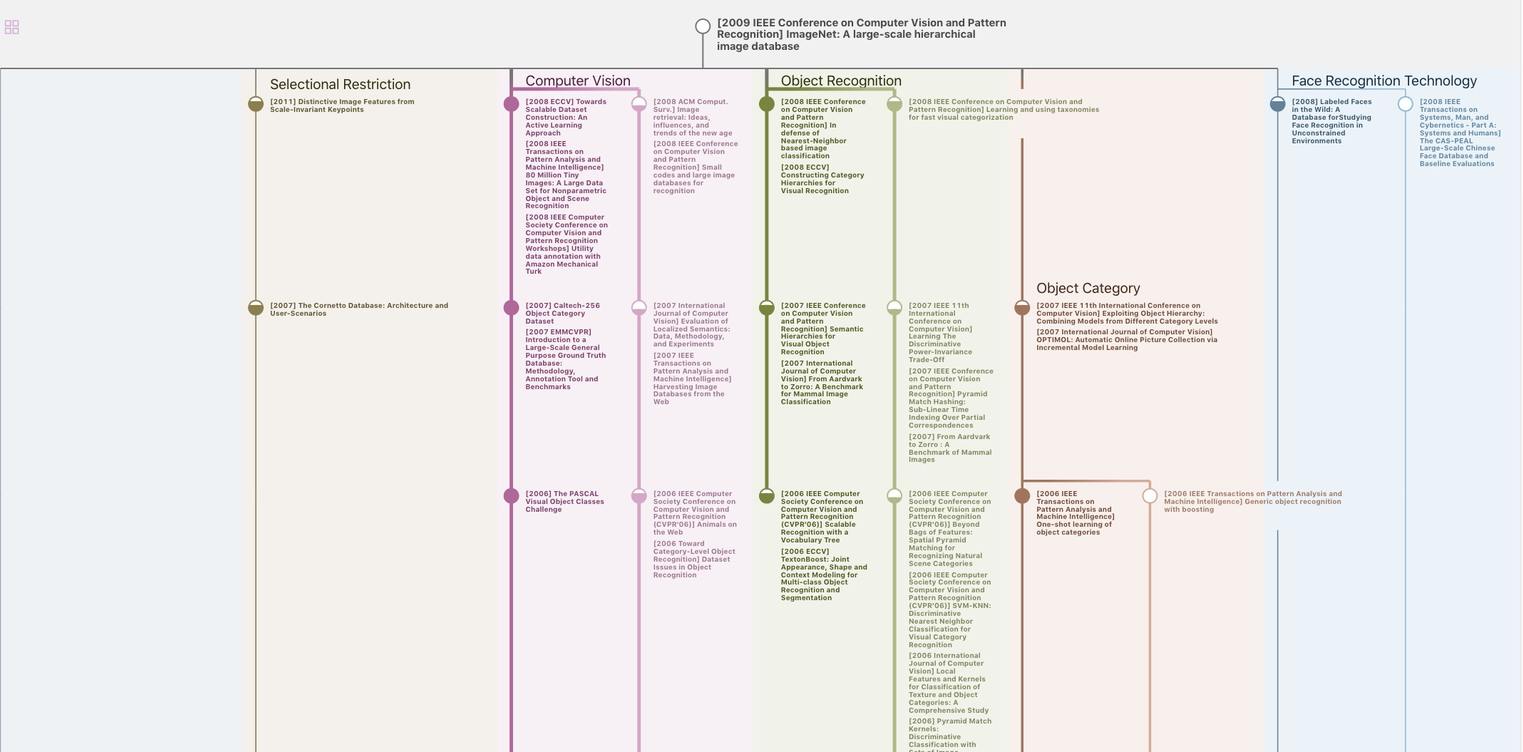
生成溯源树,研究论文发展脉络
Chat Paper
正在生成论文摘要