Interventional Video Grounding with Dual Contrastive Learning
2021 IEEE/CVF CONFERENCE ON COMPUTER VISION AND PATTERN RECOGNITION, CVPR 2021(2021)
摘要
Video grounding aims to localize a moment from an untrimmed video for a given textual query. Existing approaches focus more on the alignment of visual and language stimuli with various likelihood-based matching or regression strategies, i.e., P(Y vertical bar X). Consequently, these models may suffer from spurious correlations between the language and video features due to the selection bias of the dataset. 1) To uncover the causality behind the model and data, we first propose a novel paradigm from the perspective of the causal inference, i.e., interventional video grounding (IVG) that leverages backdoor adjustment to deconfound the selection bias based on structured causal model (SCM) and do-calculus P(Y vertical bar do(X)). Then, we present a simple yet effective method to approximate the unobserved confounder as it cannot be directly sampled from the dataset. 2) Meanwhile, we introduce a dual contrastive learning approach (DCL) to better align the text and video by maximizing the mutual information (MI) between query and video clips, and the MI between start/end frames of a target moment and the others within a video to learn more informative visual representations. Experiments on three standard benchmarks show the effectiveness of our approaches.
更多查看译文
关键词
interventional video grounding,learning,dual
AI 理解论文
溯源树
样例
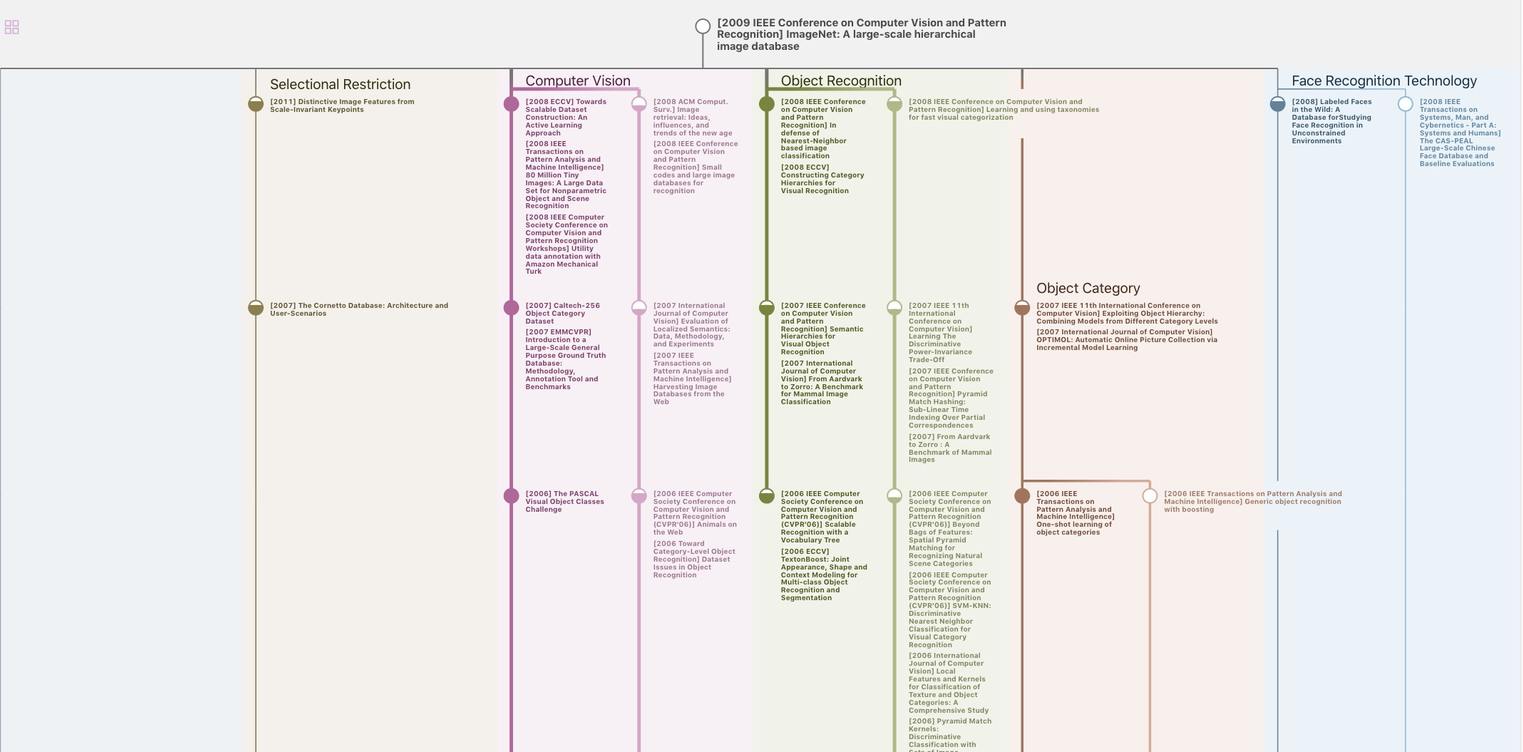
生成溯源树,研究论文发展脉络
Chat Paper
正在生成论文摘要