Learning to Warp for Style Transfer
2021 IEEE/CVF CONFERENCE ON COMPUTER VISION AND PATTERN RECOGNITION, CVPR 2021(2021)
摘要
Since its inception in 2015, Style Transfer has focused on texturing a content image using an art exemplar. Recently, the geometric changes that artists make have been acknowledged as an important component of style [42, 55, 62, 63]. Our contribution is to propose a neural network that, uniquely, learns a mapping from a 4D array of inter-feature distances to a non parametric 2D warp field. The system is generic in not being limited by semantic class, a single learned model will suffice; all examples in this paper are output from one model. Our approach combines the benefits of the high speed of Liu et al. [42] with the non-parametric warping of Kim et al. [55]. Furthermore, our system extends the normal NST paradigm: although it can be used with a single exemplar, we also allow two style exemplars: one for texture and another for geometry. This supports far greater flexibility in use cases than single exemplars can provide.
更多查看译文
关键词
geometric changes,neural network,inter-feature distances,semantic class,single learned model,nonparametric warping,single exemplar,style exemplars,Style Transfer,texturing,content image,nonparametric 2D warp field
AI 理解论文
溯源树
样例
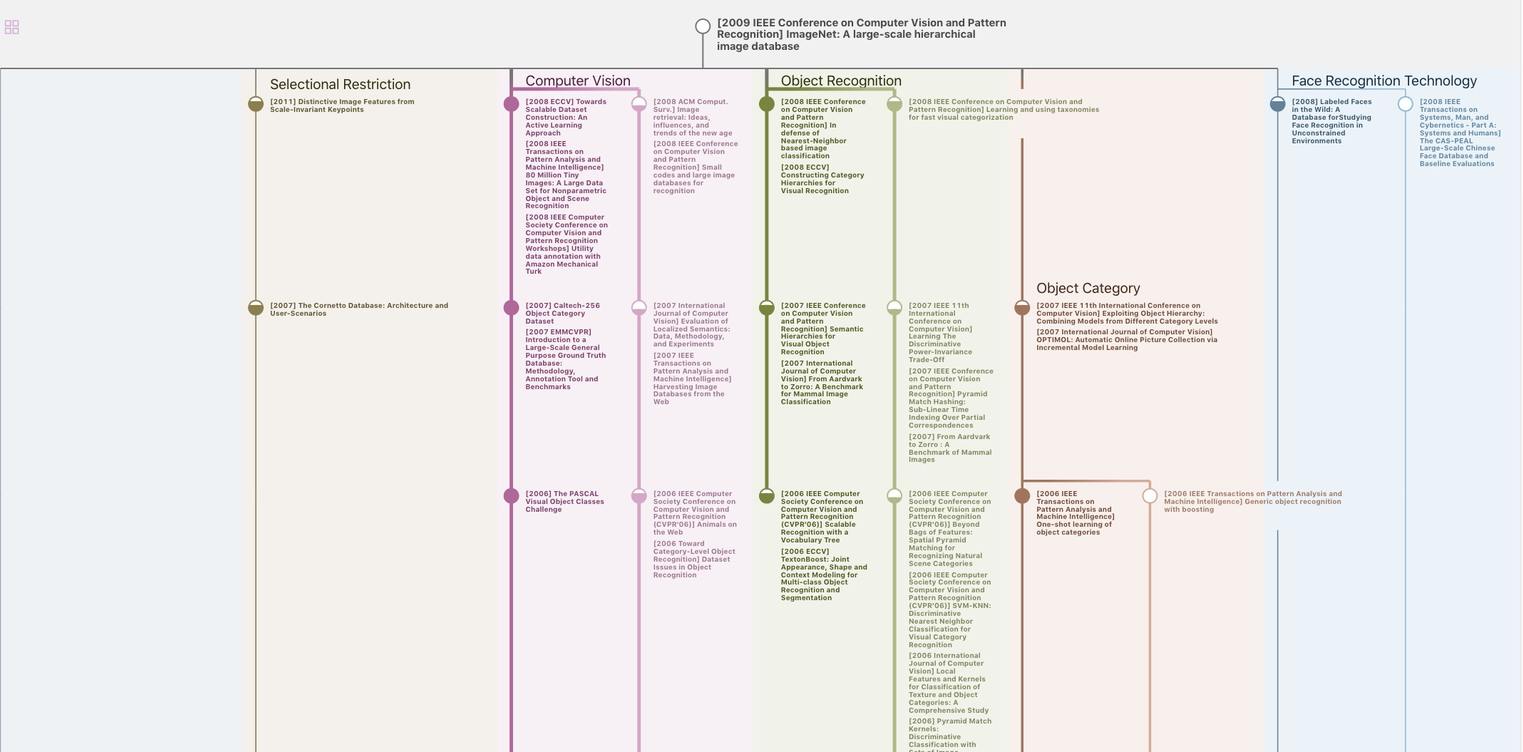
生成溯源树,研究论文发展脉络
Chat Paper
正在生成论文摘要