Can We Characterize Tasks Without Labels or Features?
2021 IEEE/CVF CONFERENCE ON COMPUTER VISION AND PATTERN RECOGNITION, CVPR 2021(2021)
摘要
The problem of expert model selection deals with choosing the appropriate pretrained network ("expert") to transfer to a target task. Methods, however, generally depend on two separate assumptions: the presence of labeled images and access to powerful "probe" networks that yield useful features. In this work, we demonstrate the current reliance on both of these aspects and develop algorithms to operate when either of these assumptions fail. In the unlabeled case, we show that pseudolabels from the probe network provide discriminative enough gradients to perform nearly-equal task selection even when the probe network is trained on imagery unrelated to the tasks. To compute the embedding with no probe network at all, we introduce the Task Tangent Kernel (TTK) which uses a kernelized distance across multiple random networks to achieve performance over double that of other methods with randomly initialized models.
更多查看译文
关键词
labeled images,probe network,nearly-equal task selection,random networks,task characterization,pretrained network,expert model selection,task tangent kernel,PseudoTask,Task2Vec algorithm,pretrained feature representation
AI 理解论文
溯源树
样例
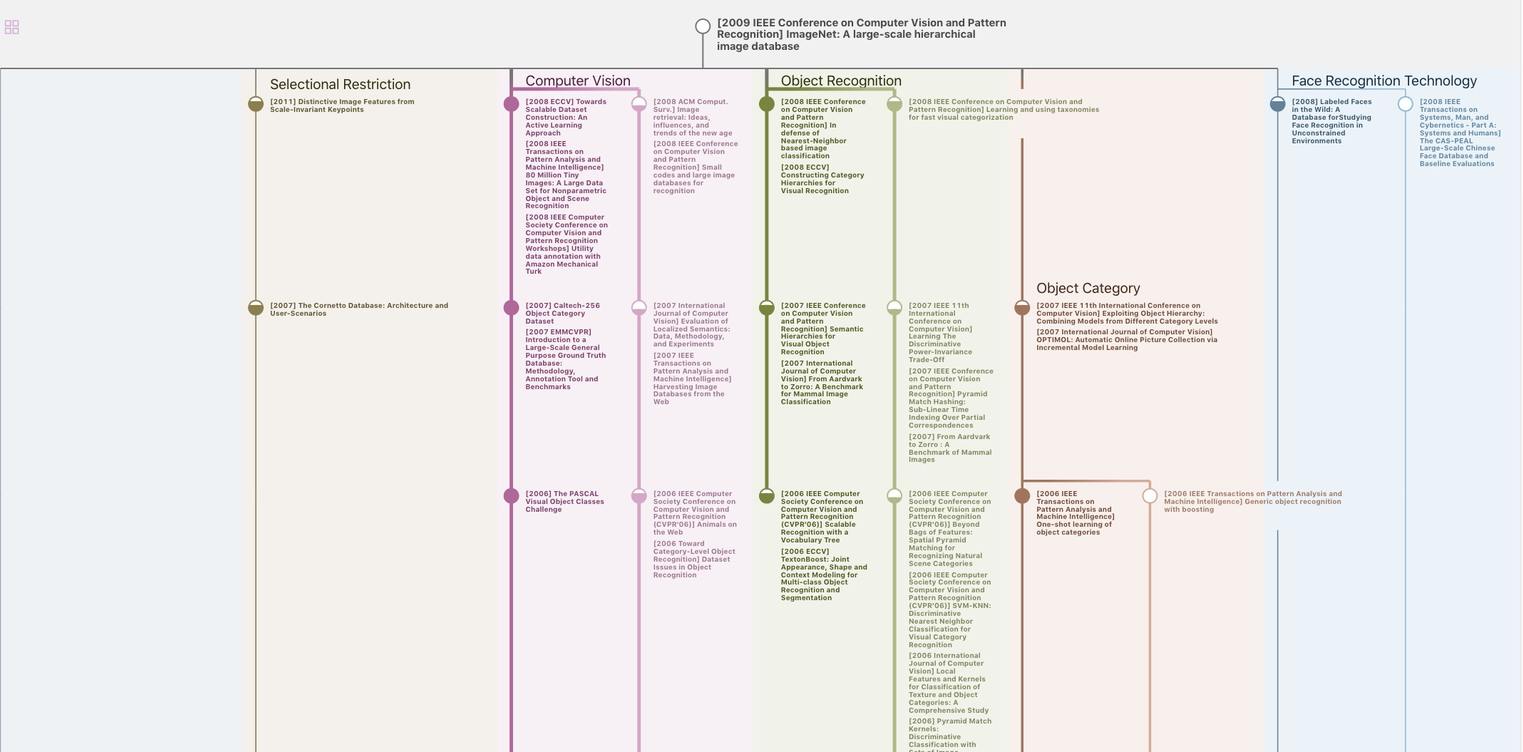
生成溯源树,研究论文发展脉络
Chat Paper
正在生成论文摘要