Predicting Human Scanpaths in Visual Question Answering
2021 IEEE/CVF CONFERENCE ON COMPUTER VISION AND PATTERN RECOGNITION, CVPR 2021(2021)
摘要
Attention has been an important mechanism for both humans and computer vision systems. While state-of-the-art models to predict attention focus on estimating a static probabilistic saliency map with free-viewing behavior, real-life scenarios are filled with tasks of varying types and complexities, and visual exploration is a temporal process that contributes to task performance. To bridge the gap, we conduct a first study to understand and predict the temporal sequences of eye fixations (a.k.a. scanpaths) during performing general tasks, and examine how scanpaths affect task performance. We present a new deep reinforcement learning method to predict scanpaths leading to different performances in visual question answering. Conditioned on a task guidance map, the proposed model learns question-specific attention patterns to generate scanpaths. It addresses the exposure bias in scanpath prediction with self-critical sequence training and designs a Consistency-Divergence loss to generate distinguishable scanpaths between correct and incorrect answers. The proposed model not only accurately predicts the spatio-temporal patterns of human behavior in visual question answering, such as fixation position, duration, and order, but also generalizes to free-viewing and visual search tasks, achieving human-level performance in all tasks and significantly outperforming the state of the art.
更多查看译文
关键词
predicting human scanpaths,visual question answering,computer vision systems,attention focus,static probabilistic saliency map,free-viewing behavior,visual exploration,temporal process,task performance,temporal sequences,general tasks,deep reinforcement learning method,task guidance map,question-specific attention patterns,scanpath prediction,self-critical sequence training,distinguishable scanpaths,correct answers,incorrect answers,spatio-temporal patterns,human behavior,visual search tasks,human-level performance
AI 理解论文
溯源树
样例
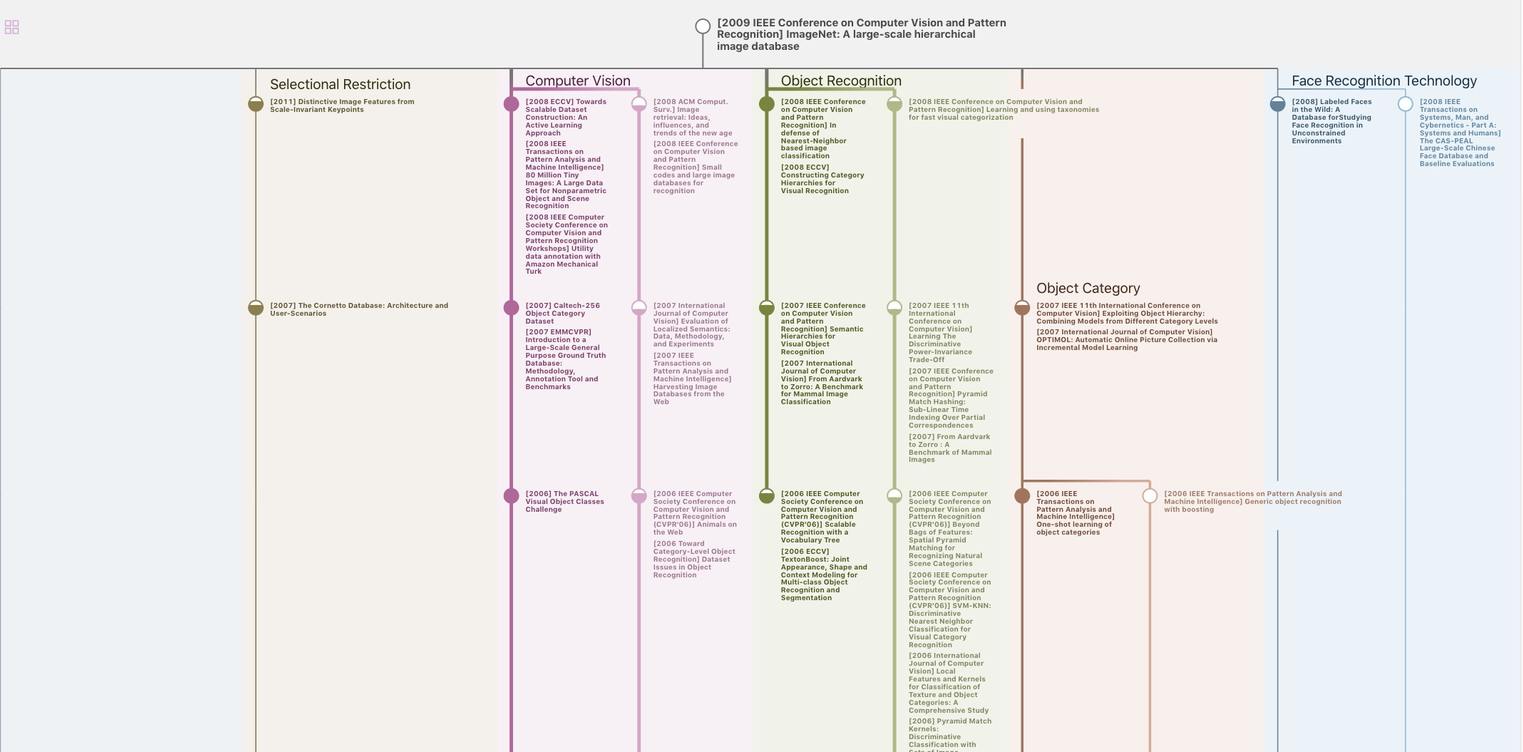
生成溯源树,研究论文发展脉络
Chat Paper
正在生成论文摘要