VirFace: Enhancing Face Recognition via Unlabeled Shallow Data
CVPR(2021)
摘要
Recently, how to exploit unlabeled data for training face recognition models has been attracting increasing attention. However, few works consider the unlabeled shallow data(1) in real-world scenarios. The existing semi-supervised face recognition methods that focus on generating pseudo labels or minimizing softmax classification probabilities of the unlabeled data do not work very well on the unlabeled shallow data. It is still a challenge on how to effectively utilize the unlabeled shallow face data to improve the performance of face recognition. In this paper, we propose a novel face recognition method, named VirFace, to effectively exploit the unlabeled shallow data for face recognition. VirFace consists of VirClass and VirInstance. Specifically, VirClass enlarges the inter-class distance by injecting the unlabeled data as new identities, while VirInstance produces virtual instances sampled from the learned distribution of each identity to further enlarge the inter-class distance. To the best of our knowledge, we are the first to tackle the problem of unlabeled shallow face data. Extensive experiments have been conducted on both the small- and large-scale datasets, e.g. LFW and IJB-C, etc, demonstrating the superiority of the proposed method.
更多查看译文
AI 理解论文
溯源树
样例
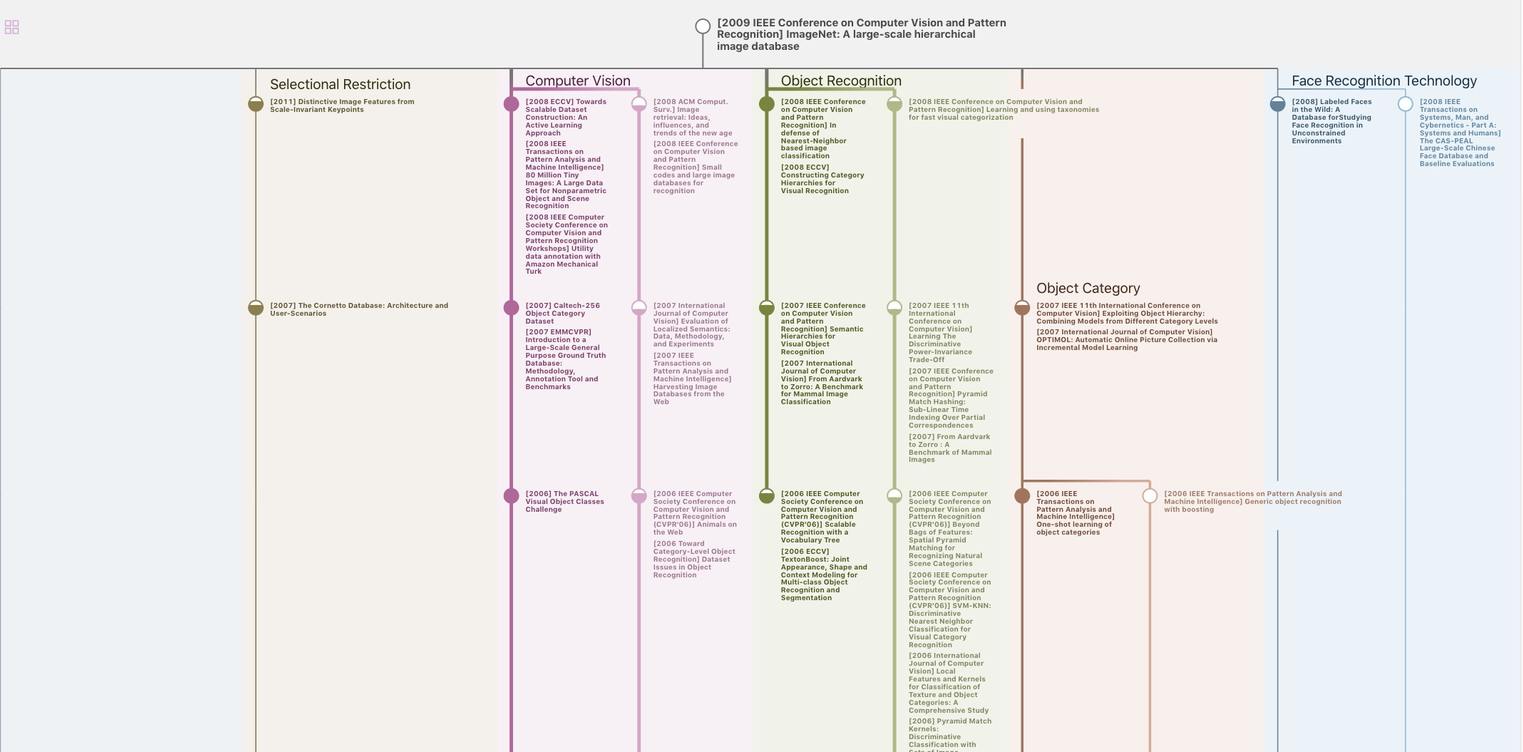
生成溯源树,研究论文发展脉络
Chat Paper
正在生成论文摘要