Influential Rank: A New Perspective of Post-training for Robust Model against Noisy Labels
ArXiv(2023)
Abstract
Deep neural network can easily overfit to even noisy labels due to its high capacity, which degrades the generalization performance of a model. To overcome this issue, we propose a new approach for learning from noisy labels (LNL) via post-training, which can significantly improve the generalization performance of any pre-trained model on noisy label data. To this end, we rather exploit the overfitting property of a trained model to identify mislabeled samples. Specifically, our post-training approach gradually removes samples with high influence on the decision boundary and refines the decision boundary to improve generalization performance. Our post-training approach creates great synergies when combined with the existing LNL methods. Experimental results on various real-world and synthetic benchmark datasets demonstrate the validity of our approach in diverse realistic scenarios.
MoreTranslated text
Key words
overfitted model property,detection,over-fit,noisy-label
AI Read Science
Must-Reading Tree
Example
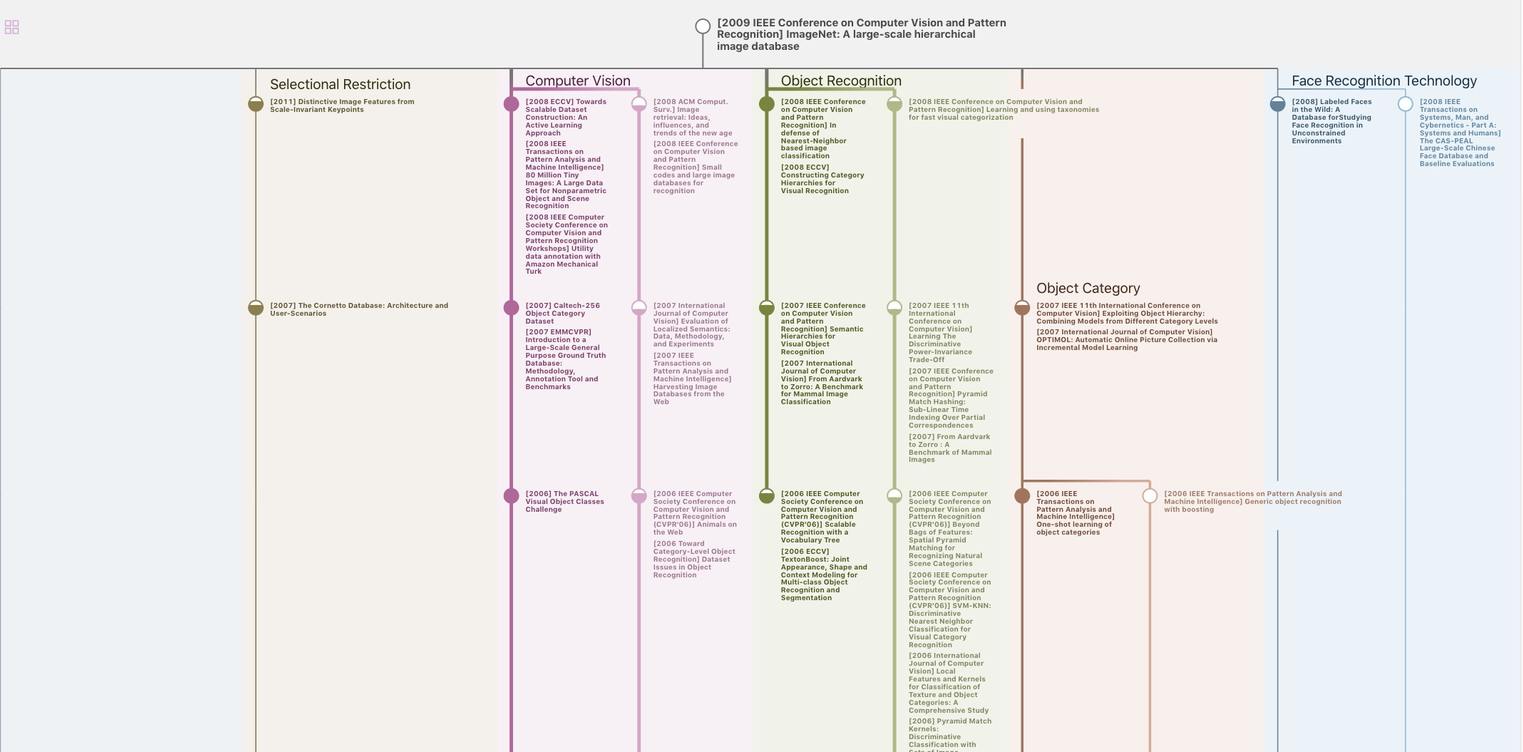
Generate MRT to find the research sequence of this paper
Chat Paper
Summary is being generated by the instructions you defined