Deep Learning for Predictive Analytics in Reversible Steganography
arxiv(2023)
摘要
Deep learning is regarded as a promising solution for reversible steganography. There is an accelerating trend of representing a reversible steo-system by monolithic neural networks, which bypass intermediate operations in traditional pipelines of reversible steganography. This end-to-end paradigm, however, suffers from imperfect reversibility. By contrast, the modular paradigm that incorporates neural networks into modules of traditional pipelines can stably guarantee reversibility with mathematical explainability. Prediction-error modulation is a well-established reversible steganography pipeline for digital images. It consists of a predictive analytics module and a reversible coding module. Given that reversibility is governed independently by the coding module, we narrow our focus to the incorporation of neural networks into the analytics module, which serves the purpose of predicting pixel intensities and a pivotal role in determining capacity and imperceptibility. The objective of this study is to evaluate the impacts of different training configurations upon predictive accuracy of neural networks and provide practical insights. In particular, we investigate how different initialisation strategies for input images may affect the learning process and how different training strategies for dual-layer prediction respond to the problem of distributional shift. Furthermore, we compare steganographic performance of various model architectures with different loss functions.
更多查看译文
关键词
Neural networks,Deep learning,Steganography,Training data,Distortion,Encoding,Watermarking,Predictive models,modularity,predictive analytics,reversible steganography
AI 理解论文
溯源树
样例
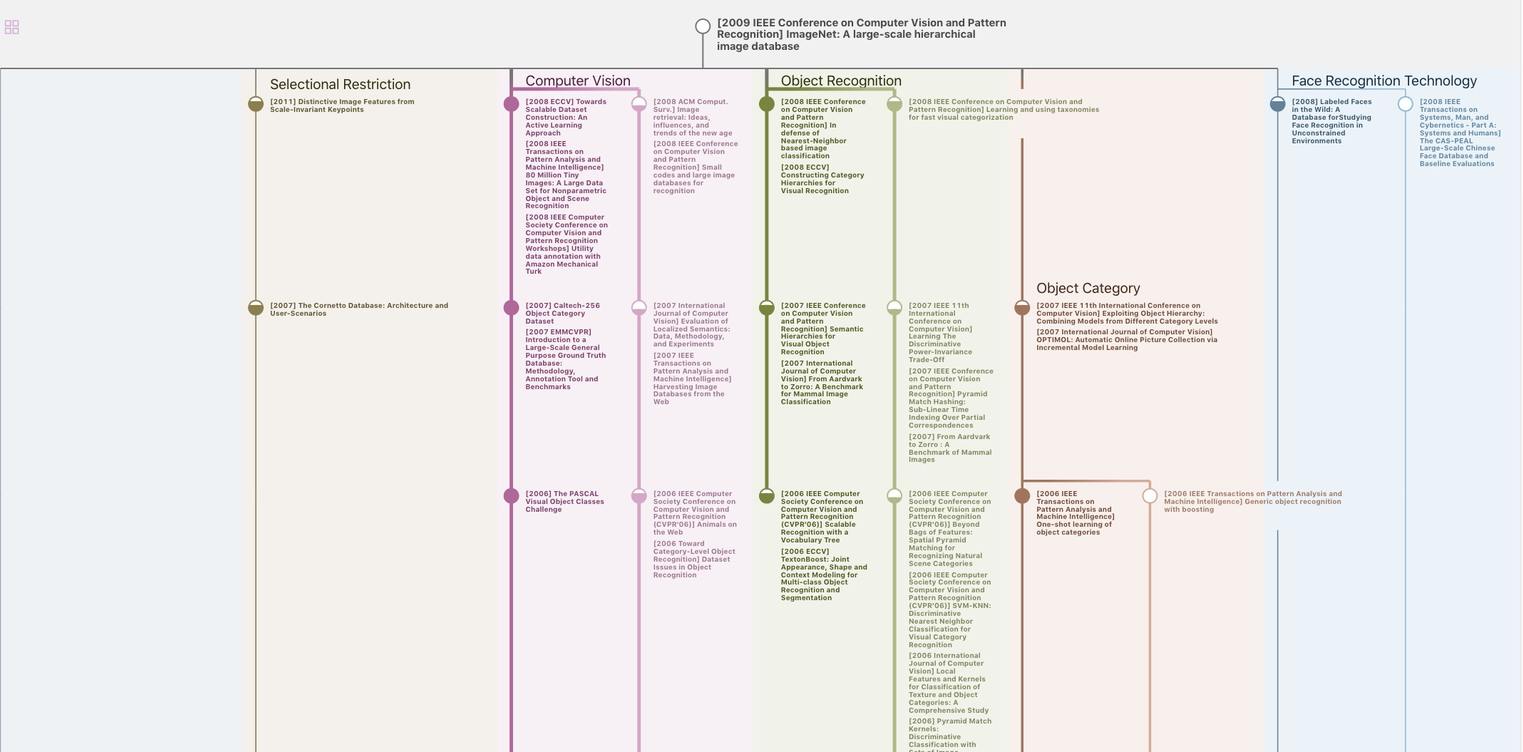
生成溯源树,研究论文发展脉络
Chat Paper
正在生成论文摘要