Fuzzy Rule-Based Models: A Design with Prototype Relocation and Granular Generalization
Information Sciences(2021)
摘要
Fuzzy rule-based models and the extension of classical fuzzy models have been widely used in many domains. From a holistic perspective, regardless of the design methods and rules adopted in a fuzzy model, the determination of fuzzy sets is a pivotal issue. In the proposed methods, instead of traditional data clustering with no directional tendency, we introduce an optimization algorithm that can adjust the position of the prototypes of zero- and first-order fuzzy models to learn internal structure information from the data in the process of parameter identification. Furthermore, to build a granular fuzzy model, the prototypes are then scaled to more robust intervals by generating information granularity with specific semantics such that they split the whole output space. Particle swarm optimization algorithm is applied to adjust both the locations of the prototypes and the allocation of information granularity to improve the performance of the data-driven models. Experimental studies on synthetic and real-world datasets are provided to demonstrate the effectiveness of these methods.
更多查看译文
关键词
Fuzzy model,Fuzzy clustering,Information granularity,Prototype,Particle swarm optimization
AI 理解论文
溯源树
样例
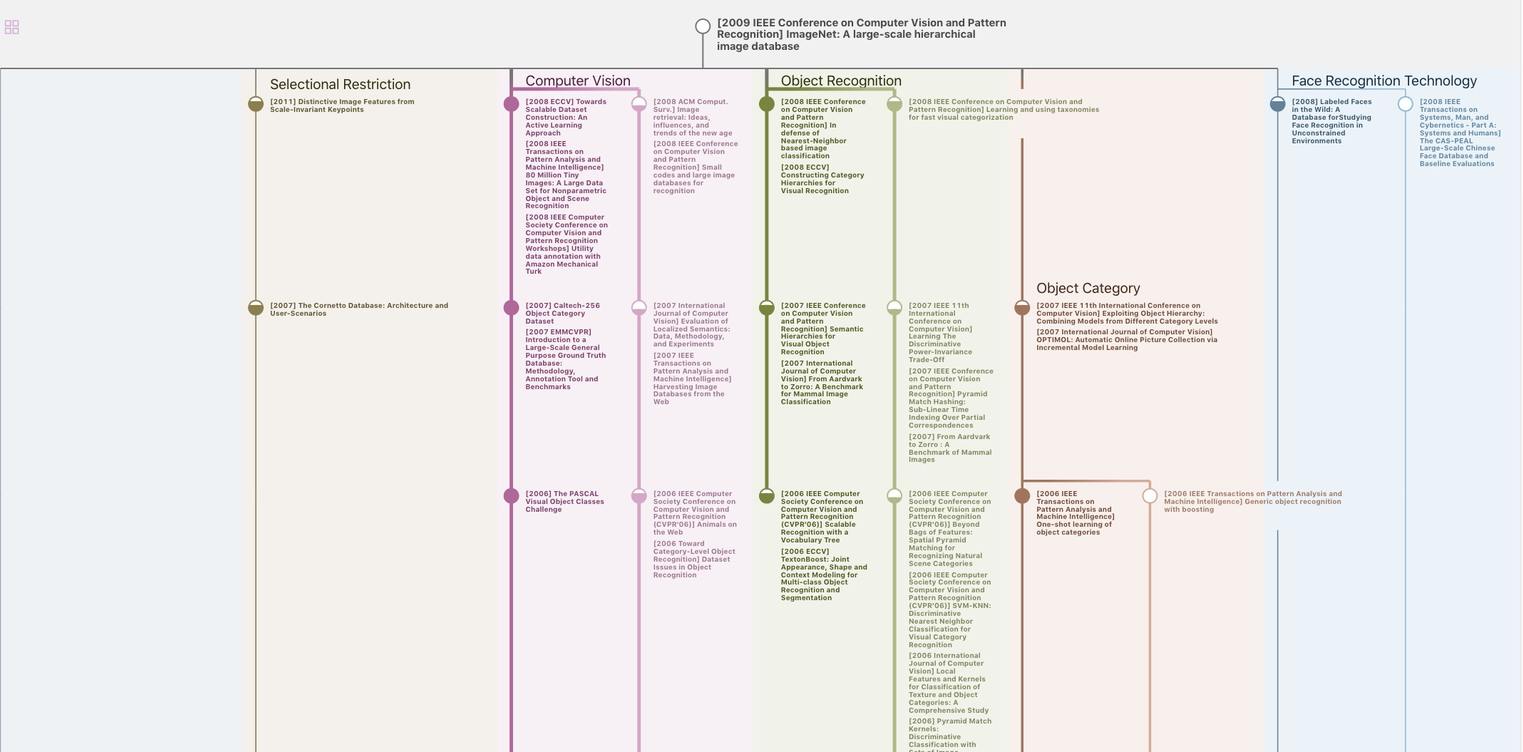
生成溯源树,研究论文发展脉络
Chat Paper
正在生成论文摘要