Multimodal Humor Dataset: Predicting Laughter Tracks for Sitcoms
IEEE Workshop/Winter Conference on Applications of Computer Vision(2021)
摘要
A great number of situational comedies (sitcoms) are being regularly made and the task of adding laughter tracks to these is a critical task. Providing an ability to be able to predict whether something will be humorous to the audience is also crucial. In this project, we aim to automate this task. Towards doing so, we annotate an existing sitcom (‘Big Bang Theory’) and use the laughter cues present to obtain a manual annotation for this show. We provide detailed analysis for the dataset design and further evaluate various state of the art baselines for solving this task. We observe that existing LSTM and BERT based networks on the text alone do not perform as well as joint text and video or only video-based networks. Moreover, it is challenging to ascertain that the words attended to while predicting laughter are indeed humorous. Our dataset and analysis provided through this paper is a valuable resource towards solving this interesting semantic and practical task. As an additional contribution, we have developed a novel model for solving this task that is a multi-modal self-attention based model that outperforms currently prevalent models for solving this task. The project page for our paper is https://delta-lab-iitk.github.io/Multimodal-Humor-Dataset/.
更多查看译文
关键词
Computer vision,Annotations,Conferences,Semantics,Bit error rate,Manuals,Task analysis
AI 理解论文
溯源树
样例
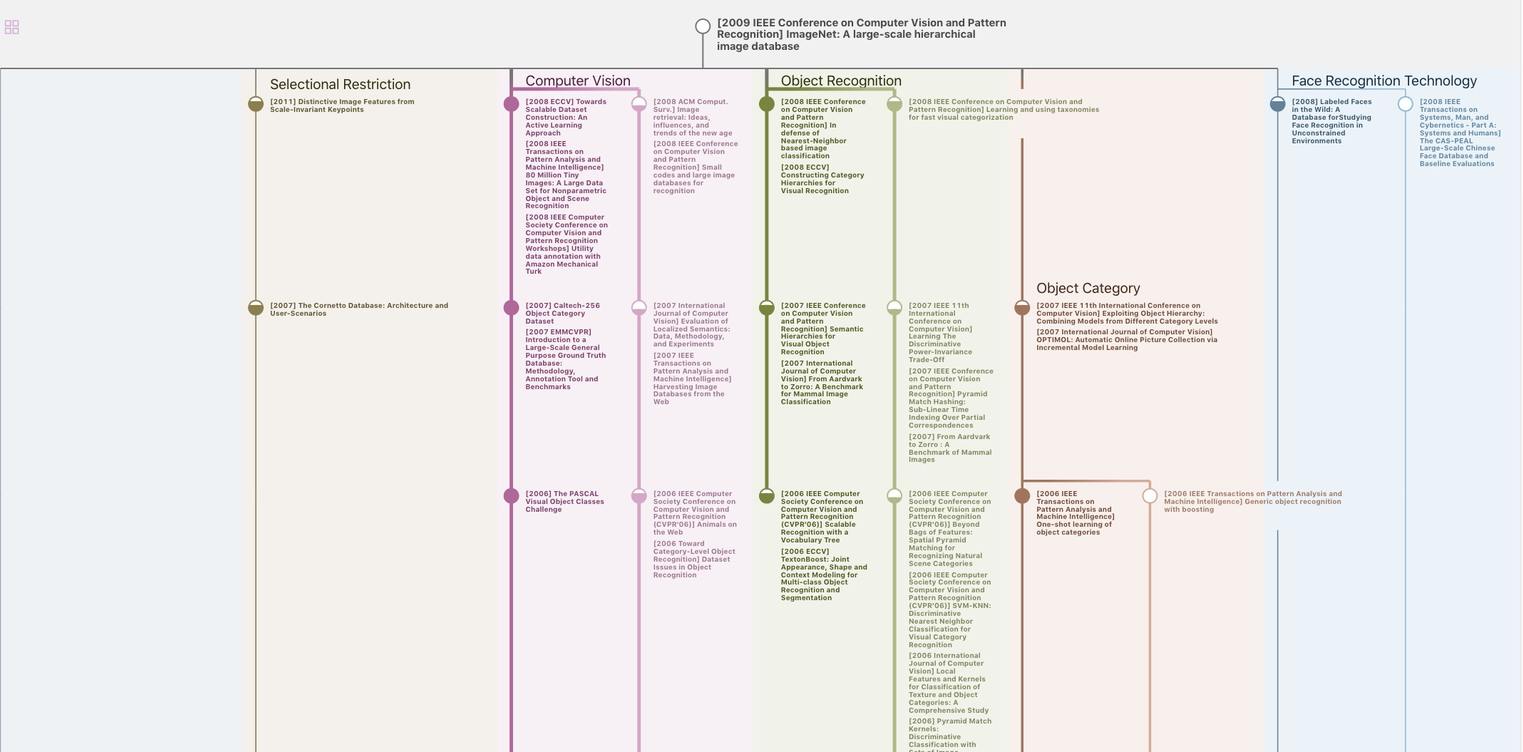
生成溯源树,研究论文发展脉络
Chat Paper
正在生成论文摘要