Inter-domain Multi-relational Link Prediction
MACHINE LEARNING AND KNOWLEDGE DISCOVERY IN DATABASES, ECML PKDD 2021: RESEARCH TRACK, PT II(2021)
摘要
Multi-relational graph is a ubiquitous and important data structure, allowing flexible representation of multiple types of interactions and relations between entities. Similar to other graph-structured data, link prediction is one of the most important tasks on multi-relational graphs and is often used for knowledge completion. When related graphs coexist, it is of great benefit to build a larger graph via integrating the smaller ones. The integration requires predicting hidden relational connections between entities belonged to different graphs (inter-domain link prediction). However, this poses a real challenge to existing methods that are exclusively designed for link prediction between entities of the same graph only (intra-domain link prediction). In this study, we propose a new approach to tackle the inter-domain link prediction problem by softly aligning the entity distributions between different domains with optimal transport and maximum mean discrepancy regularizers. Experiments on real-world datasets show that optimal transport regularizer is beneficial and considerably improves the performance of baseline methods.
更多查看译文
关键词
Inter-domain link prediction,Multi-relational data,Optimal transport
AI 理解论文
溯源树
样例
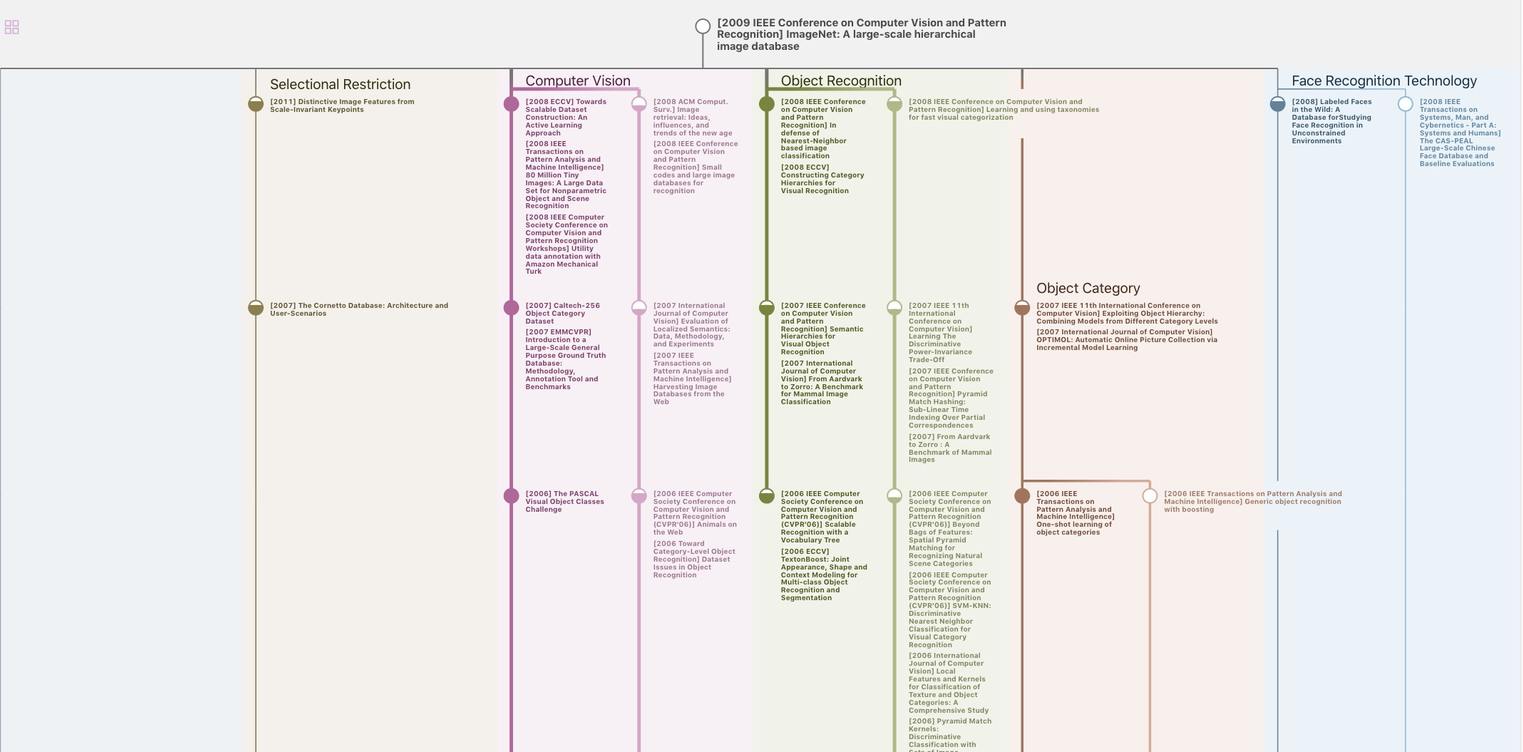
生成溯源树,研究论文发展脉络
Chat Paper
正在生成论文摘要