Data-Driven Battery Operation For Energy Arbitrage Using Rainbow Deep Reinforcement Learning
ENERGY(2022)
摘要
As the world seeks to become more sustainable, intelligent solutions are needed to increase the penetration of renewable energy. In this paper, the model-free deep reinforcement learning algorithm Rainbow Deep Q-Networks is used to control a battery in a microgrid to perform energy arbitrage and more efficiently utilise solar and wind energy sources. The grid operates with its own demand and renewable generation, as well as dynamic energy pricing from a real wholesale energy market. Four scenarios are tested including using demand and price forecasting produced with local weather data. The algorithm and its subcomponents are evaluated against an actor-critic method and a linear programming model with Rainbow able to outperform all other methods. This research shows the importance of using the distributional approach for reinforcement learning for complex environments, as well as how it can visualise and contextualise the agents' behaviour for real-world applications. (c) 2021 Elsevier Ltd. All rights reserved.
更多查看译文
关键词
Actor-critic methods, Deep Q-Networks, Demand response, Microgrids, Renewable energy
AI 理解论文
溯源树
样例
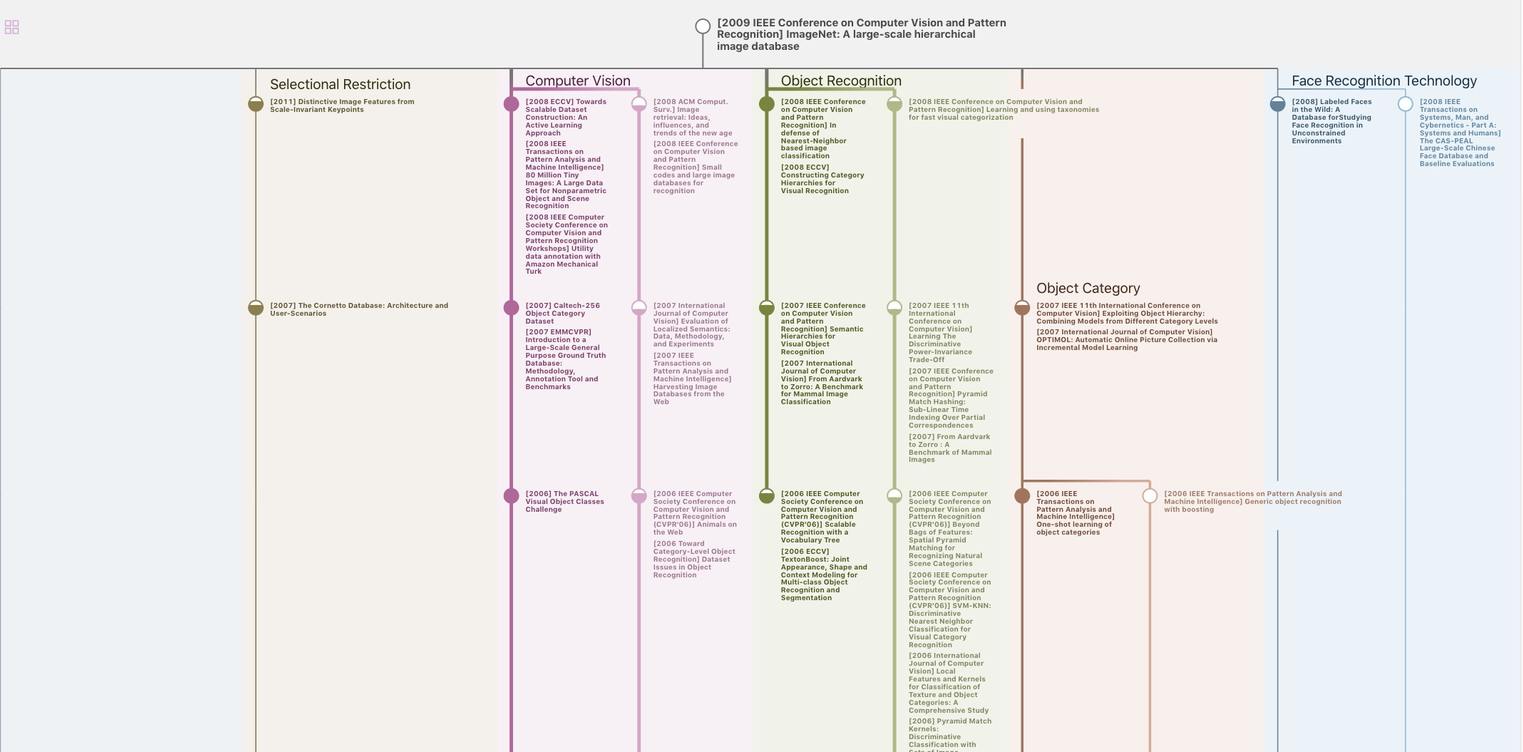
生成溯源树,研究论文发展脉络
Chat Paper
正在生成论文摘要