Deep Probabilistic Koopman: Long-term time-series forecasting under periodic uncertainties
arxiv(2021)
摘要
Probabilistic forecasting of complex phenomena is paramount to various scientific disciplines and applications. Despite the generality and importance of the problem, general mathematical techniques that allow for stable long-term forecasts with calibrated uncertainty measures are lacking. For most time series models, the difficulty of obtaining accurate probabilistic future time step predictions increases with the prediction horizon. In this paper, we introduce a surprisingly simple approach that characterizes time-varying distributions and enables reasonably accurate predictions thousands of timesteps into the future. This technique, which we call Deep Probabilistic Koopman (DPK), is based on recent advances in linear Koopman operator theory, and does not require time stepping for future time predictions. Koopman models also tend to have a small parameter footprint (often less than 10,000 parameters). We demonstrate the long-term forecasting performance of these models on a diversity of domains, including electricity demand forecasting, atmospheric chemistry, and neuroscience. For electricity demand modeling, our domain-agnostic technique outperforms all of 177 domain-specific competitors in the most recent Global Energy Forecasting Competition.
更多查看译文
关键词
deep probabilistic koopman,periodic uncertainties,time-series time-series,long-term
AI 理解论文
溯源树
样例
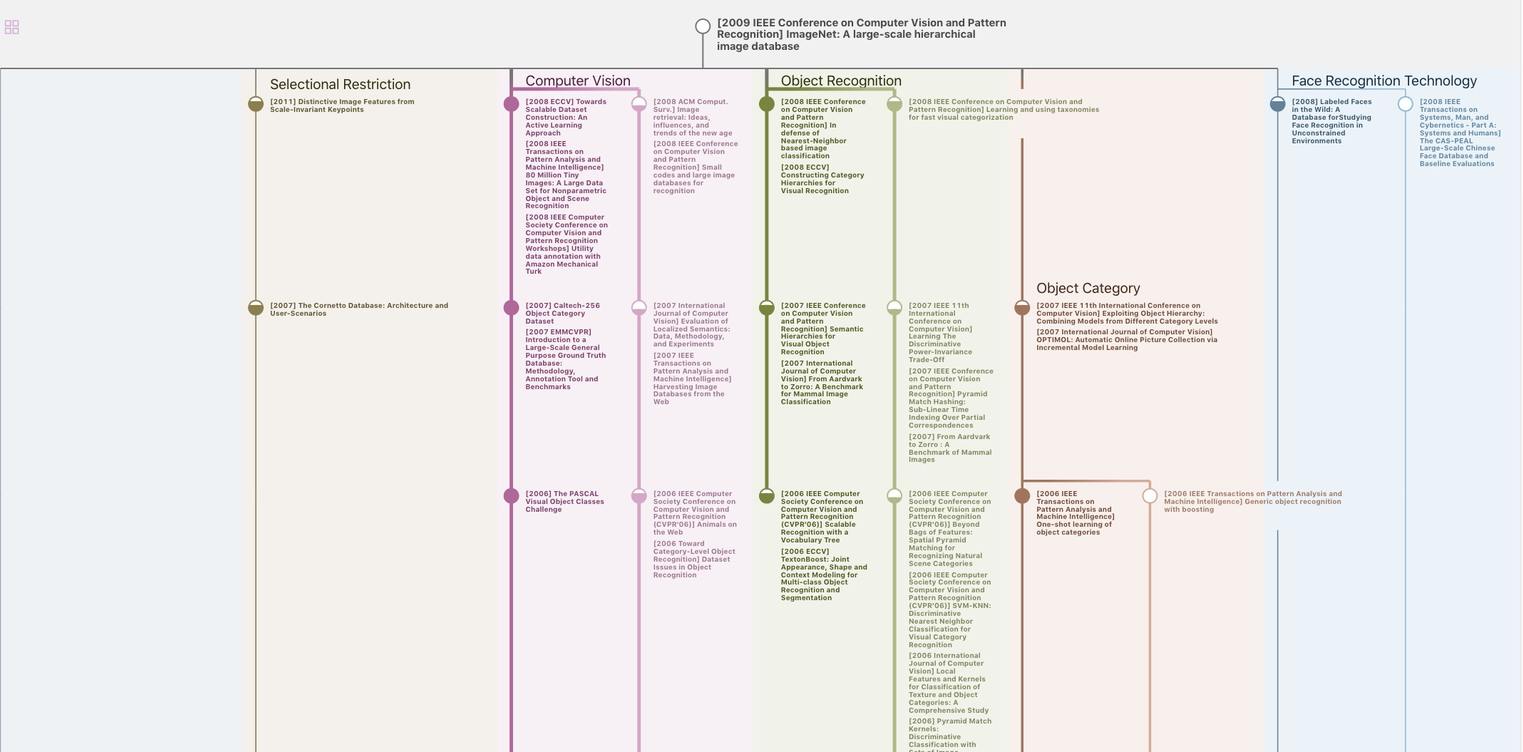
生成溯源树,研究论文发展脉络
Chat Paper
正在生成论文摘要