Analysis of Evolved Response Thresholds for Decentralized Dynamic Task Allocation
ACM Transactions on Evolutionary Learning and Optimization(2022)
摘要
In this work, we investigate the application of a multi-objective genetic algorithm to the problem of task allocation in a self-organizing, decentralized, threshold-based swarm. We use a multi-objective genetic algorithm to evolve response thresholds for a simulated swarm engaged in dynamic task allocation problems: two-dimensional and three-dimensional collective tracking. We show that evolved thresholds not only outperform uniformly distributed thresholds and dynamic thresholds but achieve nearly optimal performance on a variety of tracking problem instances (target paths). More importantly, we demonstrate that thresholds evolved for some problem instances generalize to all other problem instances, eliminating the need to evolve new thresholds for each problem instance to be solved. We analyze the properties that allow these paths to serve as universal training instances and show that they are quite natural.After a priori evolution, the response thresholds in our system are static. The problem instances solved by the swarms are highly dynamic, with schedules of task demands that change over time with significant differences in rate and magnitude of change. That the swarm is able to achieve nearly optimal results refutes the common assumption that a swarm must be dynamic to perform well in a dynamic environment.
更多查看译文
关键词
decentralized dynamic task
AI 理解论文
溯源树
样例
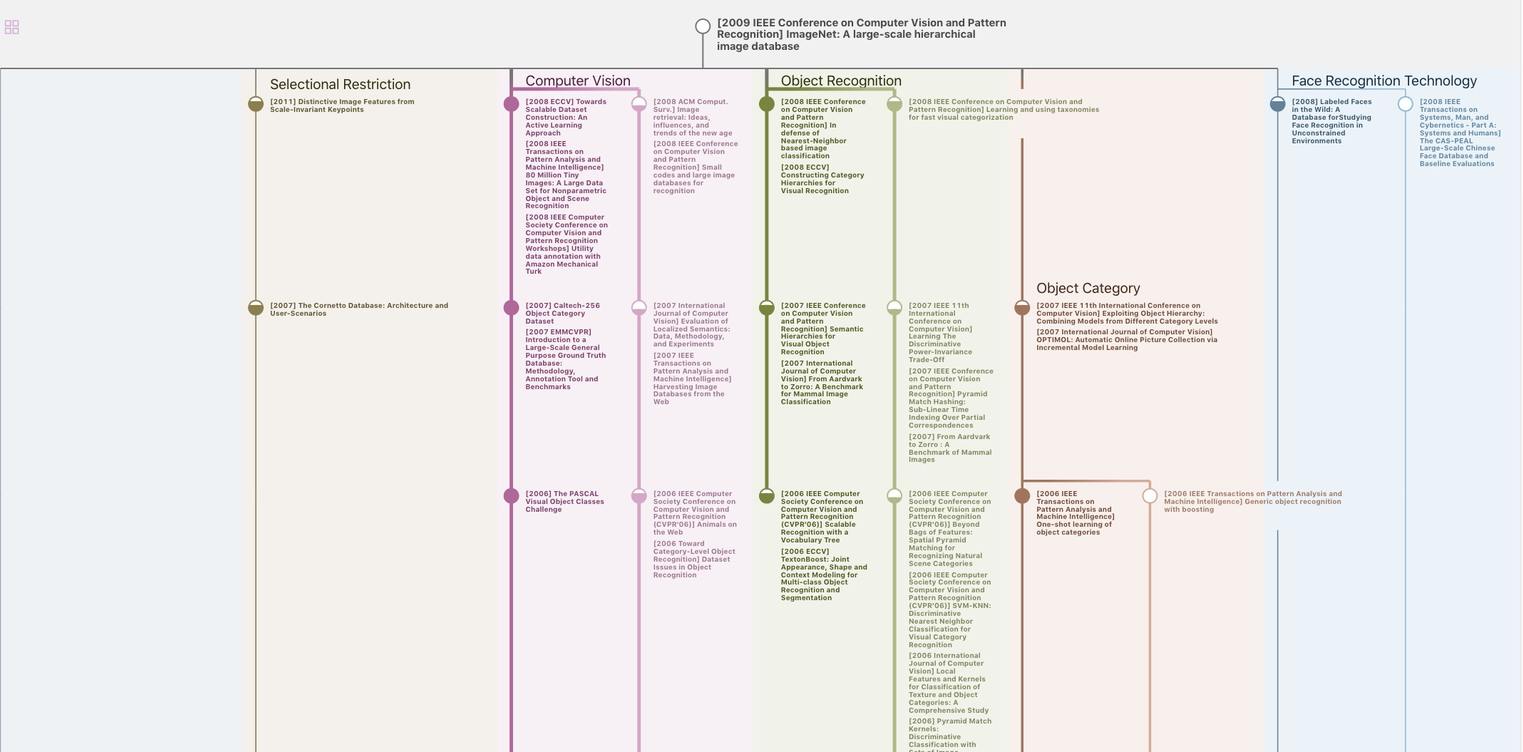
生成溯源树,研究论文发展脉络
Chat Paper
正在生成论文摘要