Mussels as Aquatic Pollution Biosensors using Neural Networks and Control Charts.
INDIN(2020)
Abstract
Even though the oil industry importance is notable, oil exploitation may cause impairment of aquatic organisms due to the risk of oil spills. In this context, the development of low-cost and effective aquatic pollution sensors has paramount importance. The present study proposes the association of Nonlinear Autoregressive (NAR) neural network and Exponentially Weighted Moving Average (EWMA) control chart in the behavioral analysis of Perna perna mussels. Bivalve mollusks were instrumented with hall effect sensors and magnets, maintained under controlled environmental conditions and exposed to different concentrations of diesel S-500 Water-Accommodated Fraction. Behavioral data were acquired before (3 days) and along (44 hours) toxicological exposure. NAR neural network was used to forecast the Average Opening Amplitude (AOA) of Perna perna mussels under a non-toxicological environment. It was effective in predicting non-exposed behavior of mussels and allowed to consider individual's adaptive nature. EWMA control chart was employed to evaluate the residues among neural network forecast and experimental AOA. The exposure of bivalves to diesel WAF provided a discrepancy among the predicted and experimental AOA. Hence, EWMA control chart has provided out of control (unpredictable) values throughout the toxicological exposure period. The association of NAR neural networks and EWMA control charts is a potential tool in online monitoring of aquatic environments and considers individual peculiarities of each bivalve which leads to the development of more accurate aquatic pollution biosensors.
MoreTranslated text
Key words
biosensors,NAR neural network,EWMA control chart,aquatic pollution
AI Read Science
Must-Reading Tree
Example
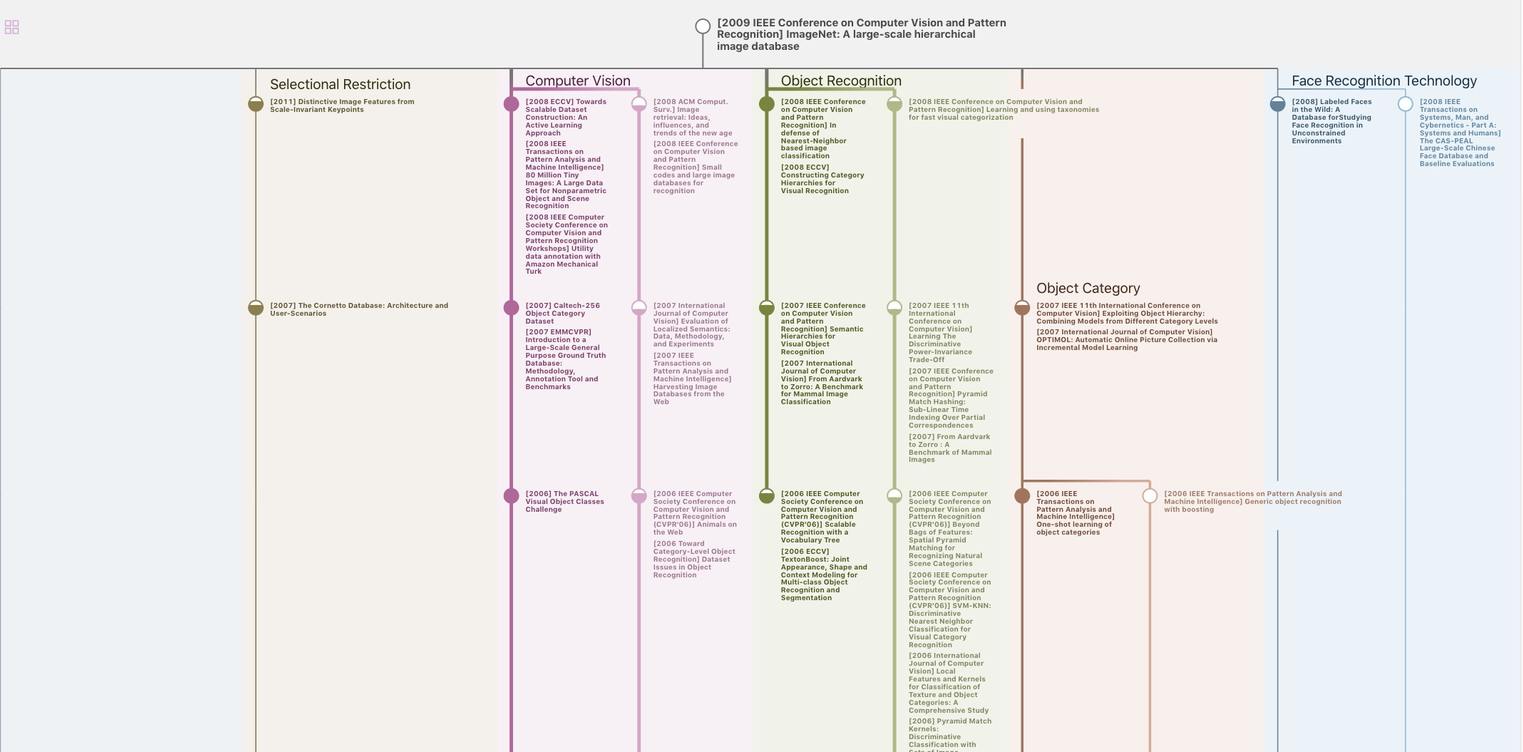
Generate MRT to find the research sequence of this paper
Chat Paper
Summary is being generated by the instructions you defined