SignalNet: A Low Resolution Sinusoid Decomposition and Estimation Network
IEEE TRANSACTIONS ON SIGNAL PROCESSING(2022)
摘要
The detection and estimation of sinusoids is a fundamental signal processing task for many applications related to sensing and communications. While algorithms have been proposed for this setting, quantization is a critical, but often ignored modeling effect. In wireless communications, estimation with low resolution data converters is relevant for reduced power consumption in wideband receivers. Similarly, low resolution sampling in imaging and spectrum sensing allows for efficient data collection. In this work, we propose SignalNet, a neural network architecture that detects the number of sinusoids and estimates their parameters from quantized in-phase and quadrature samples. We incorporate signal reconstruction internally as domain knowledge within the network to enhance learning and surpass traditional algorithms in mean squared error and Chamfer error. We introduce a worst-case learning threshold for comparing the results of our network relative to the underlying data distributions. This threshold provides insight into why neural networks tend to outperform traditional methods and into the learned relationships between the input and output distributions. In simulation, we find that our algorithm is always able to surpass the threshold for three-bit data but often cannot exceed the threshold for one-bit data. We use the learning threshold to explain, in the one-bit case, how our estimators learn to minimize the distributional loss, rather than learn features from the data.
更多查看译文
关键词
Signal processing algorithms, Estimation, Quantization (signal), Signal resolution, Task analysis, Neural networks, Data models, Sinusoid decomposition, estimation, deep-learning, low-resolution, detection
AI 理解论文
溯源树
样例
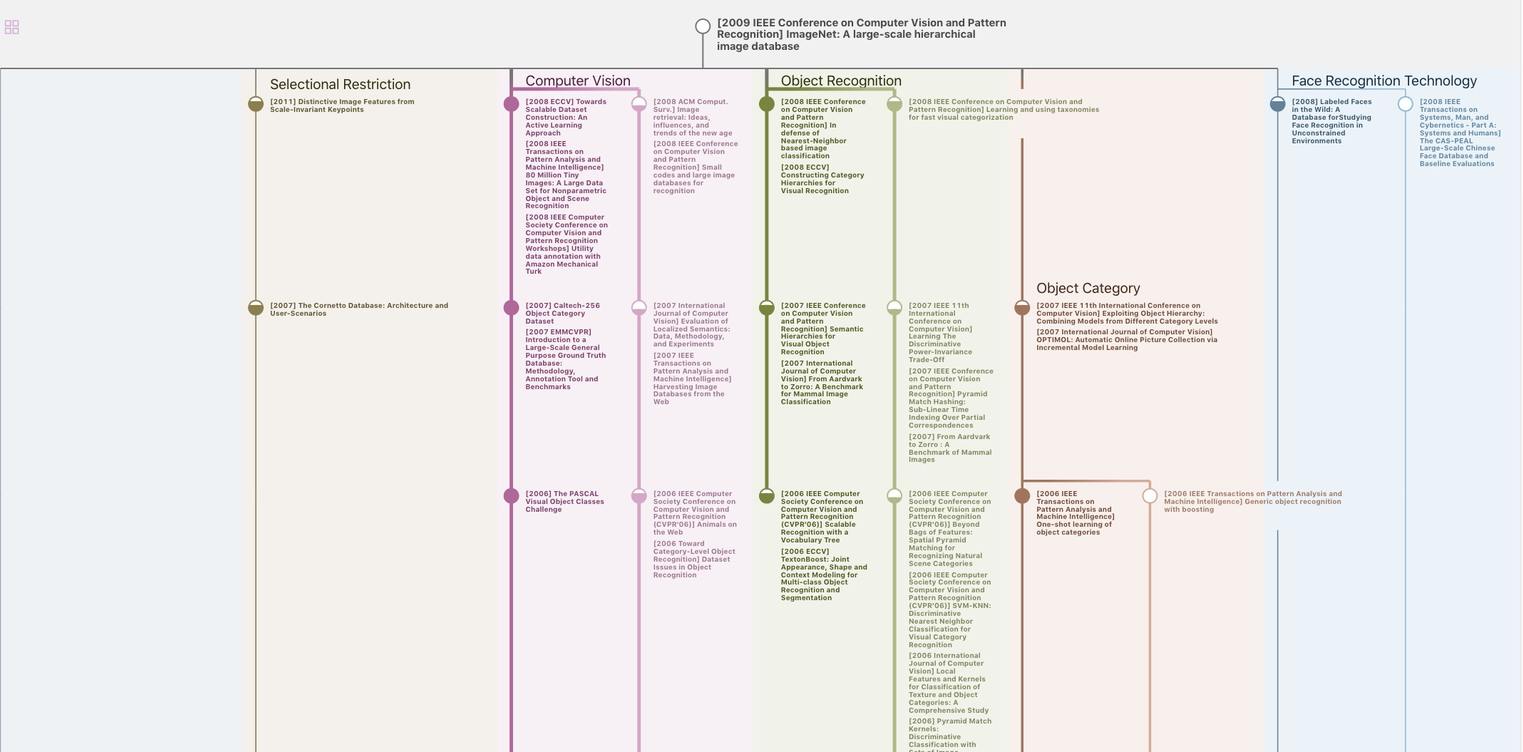
生成溯源树,研究论文发展脉络
Chat Paper
正在生成论文摘要