Attentional Meta-learners for Few-shot Polythetic Classification
International Conference on Machine Learning(2022)
摘要
Polythetic classifications, based on shared patterns of features that need neither be universal nor constant among members of a class, are common in the natural world and greatly outnumber monothetic classifications over a set of features. We show that threshold meta-learners, such as Prototypical Networks, require an embedding dimension that is exponential in the number of task-relevant features to emulate these functions. In contrast, attentional classifiers, such as Matching Networks, are polythetic by default and able to solve these problems with a linear embedding dimension. However, we find that in the presence of task-irrelevant features, inherent to meta-learning problems, attentional models are susceptible to misclassification. To address this challenge, we propose a self-attention feature-selection mechanism that adaptively dilutes non-discriminative features. We demonstrate the effectiveness of our approach in meta-learning Boolean functions, and synthetic and real-world few-shot learning tasks.
更多查看译文
关键词
polythetic classification,meta-learners,few-shot
AI 理解论文
溯源树
样例
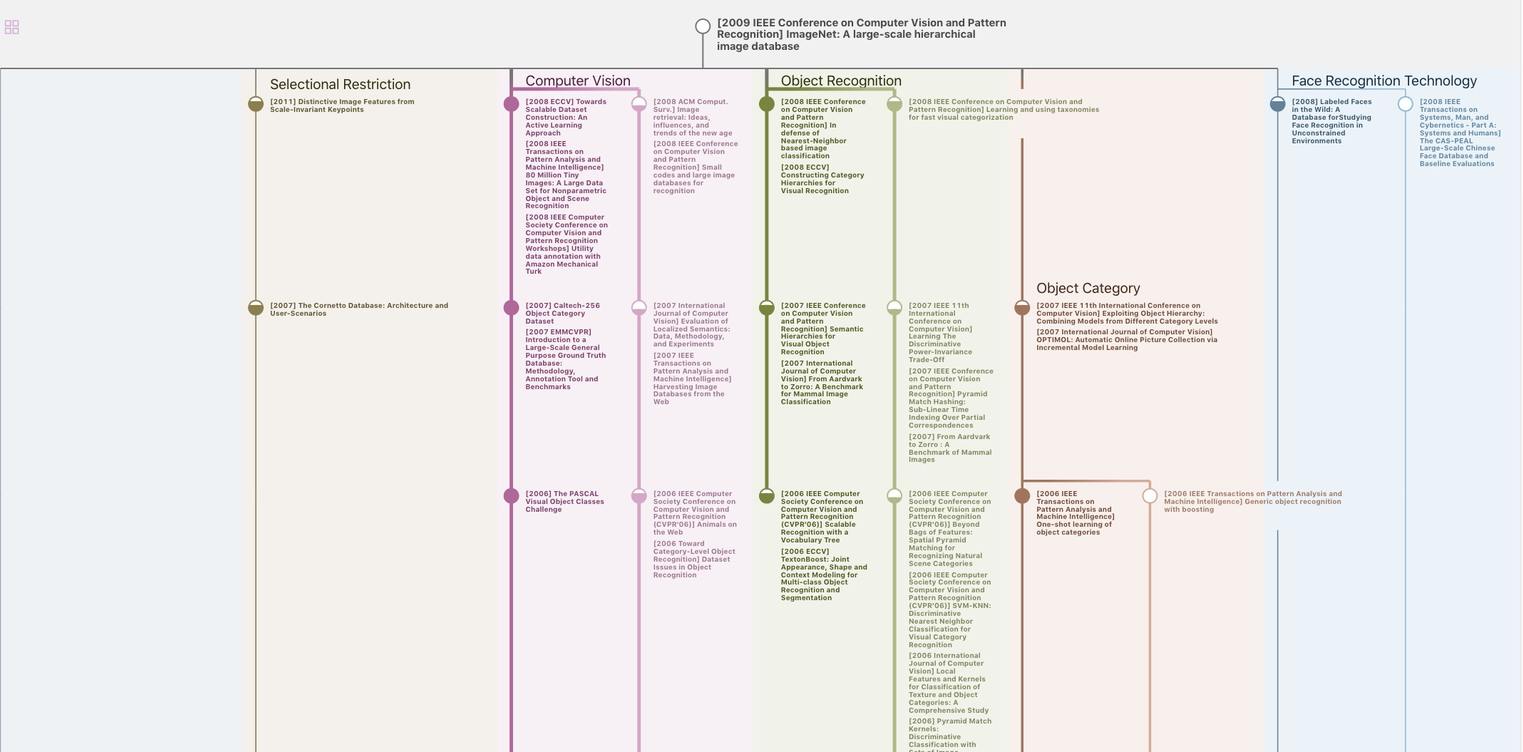
生成溯源树,研究论文发展脉络
Chat Paper
正在生成论文摘要