Machine learning of superconducting critical temperature from Eliashberg theory
NPJ COMPUTATIONAL MATERIALS(2022)
摘要
The Eliashberg theory of superconductivity accounts for the fundamental physics of conventional superconductors, including the retardation of the interaction and the Coulomb pseudopotential, to predict the critical temperature T c . McMillan, Allen, and Dynes derived approximate closed-form expressions for the critical temperature within this theory, which depends on the electron–phonon spectral function α 2 F ( ω ). Here we show that modern machine-learning techniques can substantially improve these formulae, accounting for more general shapes of the α 2 F function. Using symbolic regression and the SISSO framework, together with a database of artificially generated α 2 F functions and numerical solutions of the Eliashberg equations, we derive a formula for T c that performs as well as Allen–Dynes for low- T c superconductors and substantially better for higher- T c ones. This corrects the systematic underestimation of T c while reproducing the physical constraints originally outlined by Allen and Dynes. This equation should replace the Allen–Dynes formula for the prediction of higher-temperature superconductors.
更多查看译文
关键词
critical temperature,eliashberg
AI 理解论文
溯源树
样例
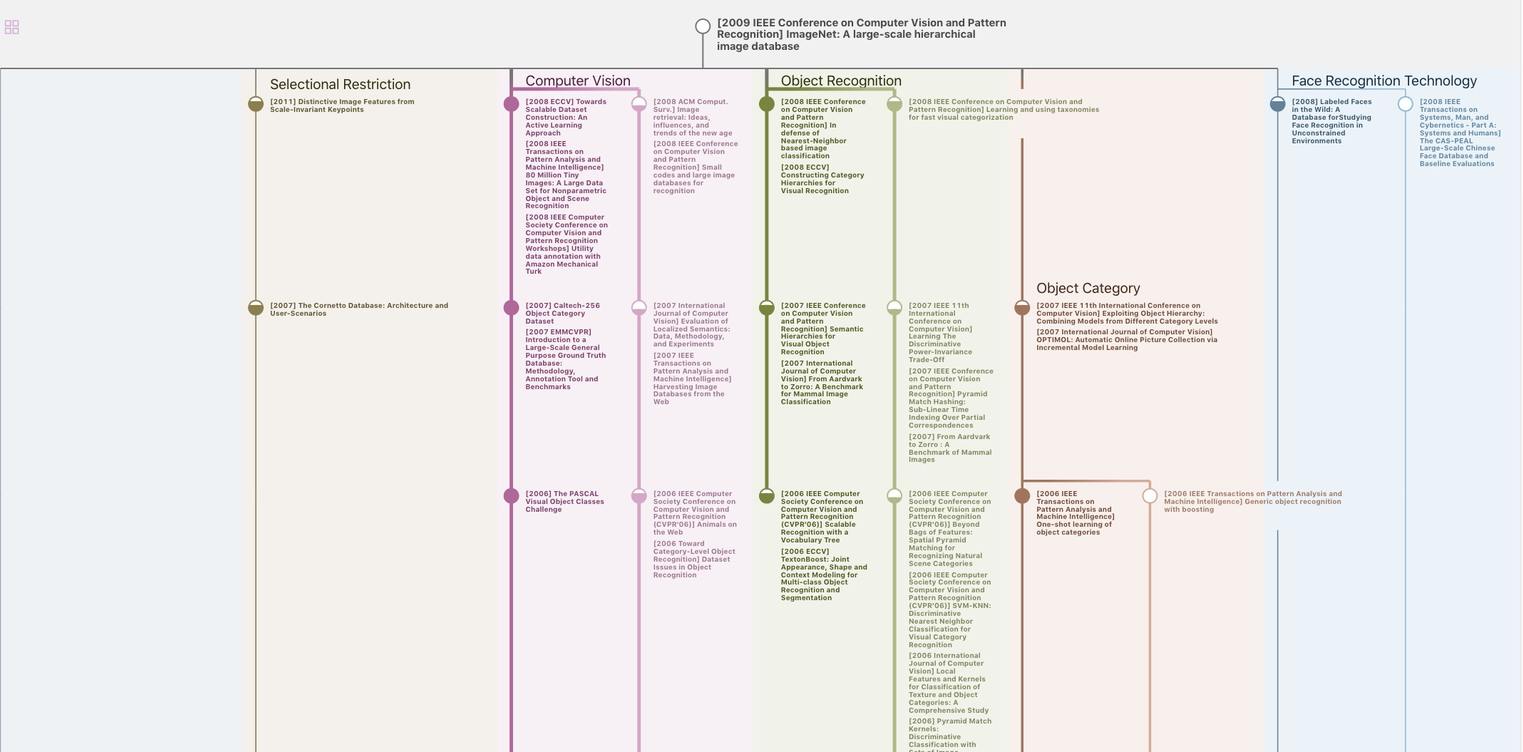
生成溯源树,研究论文发展脉络
Chat Paper
正在生成论文摘要