Hermite Polynomial Features for Private Data Generation.
International Conference on Machine Learning(2022)
Abstract
Kernel mean embedding is a useful tool to compare probability measures. Despite its usefulness, kernel mean embedding considers infinite-dimensional features, which are challenging to handle in the context of differentially private data generation. A recent work, DP-MERF (Harder et al., 2021), proposes to approximate the kernel mean embedding of data distribution using finite-dimensional random features, which yields an analytically tractable sensitivity of approximate kernel mean embedding. However, the required number of random features in DP-MERF is excessively high, often ten thousand to a hundred thousand, which worsens the sensitivity of the approximate kernel mean embedding. To improve the sensitivity, we propose to replace random features with Hermite polynomial features. Unlike the random features, the Hermite polynomial features are ordered, where the features at the low orders contain more information on the distribution than those at the high orders. Hence, a relatively low order of Hermite polynomial features can more accurately approximate the mean embedding of the data distribution compared to a significantly higher number of random features. As a result, the Hermite polynomial features help us to improve the privacy-accuracy trade-off compared to DP-MERF, as demonstrated on several heterogeneous tabular datasets, as well as several image benchmark datasets.
MoreTranslated text
AI Read Science
Must-Reading Tree
Example
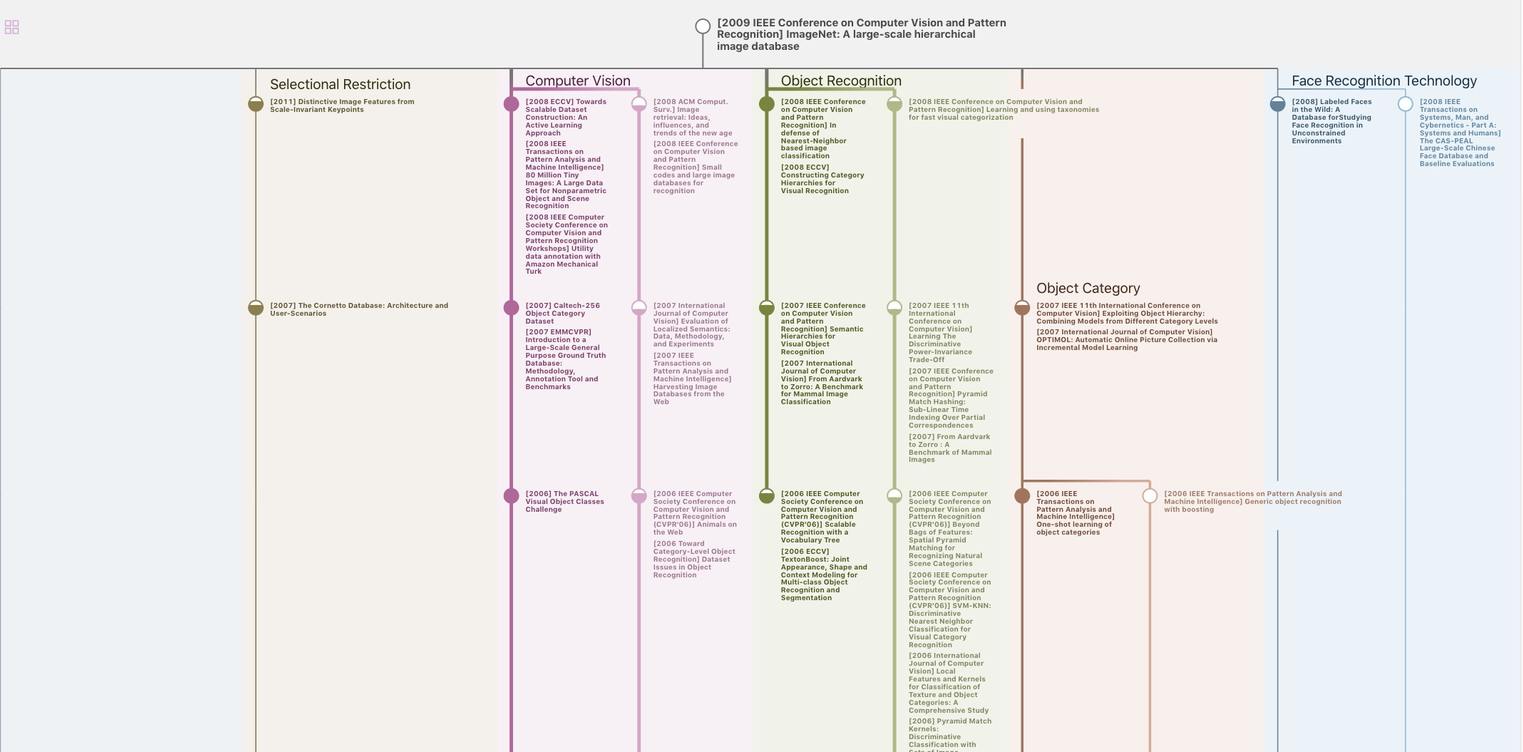
Generate MRT to find the research sequence of this paper
Chat Paper
Summary is being generated by the instructions you defined