Temporal Averaging LSTM-based Channel Estimation Scheme for IEEE 802.11p Standard
2021 IEEE GLOBAL COMMUNICATIONS CONFERENCE (GLOBECOM)(2021)
摘要
In vehicular communications, reliable channel estimation is critical for the system performance due to the doubly-dispersive nature of vehicular channels. IEEE 802.11p standard allocates insufficient pilots for accurate channel tracking. Consequently, conventional IEEE 802.11p estimators suffer from a considerable performance degradation, especially in high mobility scenarios. Recently, deep learning (DL) techniques have been employed for IEEE 802.11p channel estimation. Nevertheless, these methods suffer either from performance degradation in very high mobility scenarios or from large computational complexity. In this paper, these limitations are solved using a long short term memory (LSTM)-based estimation. The proposed estimator employs an LSTM unit to estimate the channel, followed by temporal averaging (TA) processing as a noise alleviation technique. Moreover, the noise mitigation ratio is determined analytically, thus validating the TA processing ability in improving the overall performance. Simulation results reveal the performance superiority of the proposed schemes compared to the recently proposed DL-based estimators, while recording a significant reduction in the computational complexity.
更多查看译文
关键词
Channel estimation, deep learning, LSTM, vehicular communications, IEEE 802.11p standard
AI 理解论文
溯源树
样例
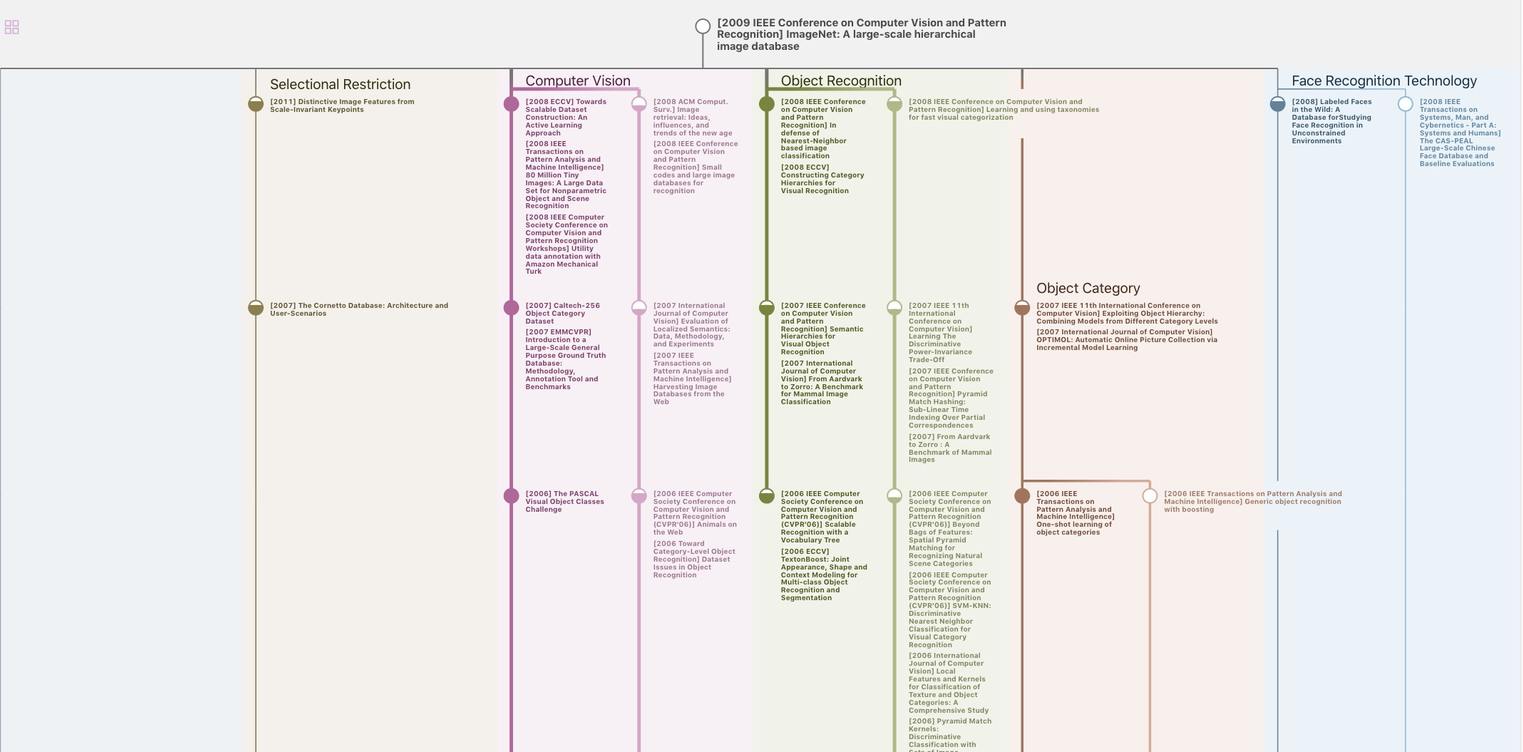
生成溯源树,研究论文发展脉络
Chat Paper
正在生成论文摘要