Fixed-Budget Best-Arm Identification in Contextual Bandits: A Static-Adaptive Algorithm
arxiv(2021)
摘要
We study the problem of best-arm identification (BAI) in contextual bandits in the fixed-budget setting. We propose a general successive elimination algorithm that proceeds in stages and eliminates a fixed fraction of suboptimal arms in each stage. This design takes advantage of the strengths of static and adaptive allocations. We analyze the algorithm in linear models and obtain a better error bound than prior work. We also apply it to generalized linear models (GLMs) and bound its error. This is the first BAI algorithm for GLMs in the fixed-budget setting. Our extensive numerical experiments show that our algorithm outperforms the state of art.
更多查看译文
关键词
contextual bandits,fixed-budget,best-arm,static-adaptive
AI 理解论文
溯源树
样例
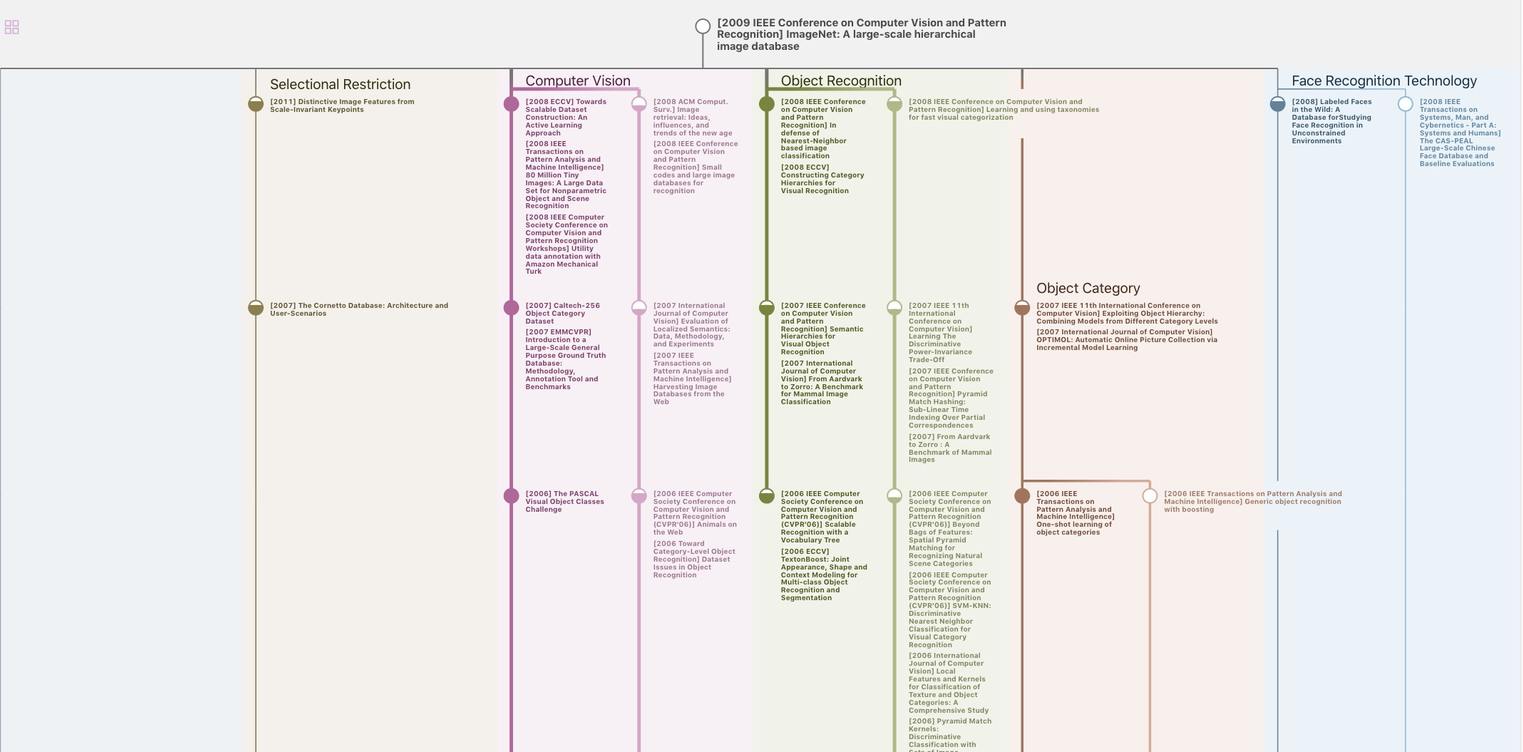
生成溯源树,研究论文发展脉络
Chat Paper
正在生成论文摘要