Back2Future: Leveraging Backfill Dynamics for Improving Real-time Predictions in Future
International Conference on Learning Representations (ICLR)(2022)
摘要
In real-time forecasting in public health, data collection is a non-trivial and demanding task. Often after initially released, it undergoes several revisions later (maybe due to human or technical constraints) - as a result, it may take weeks until the data reaches to a stable value. This so-called 'backfill' phenomenon and its effect on model performance has been barely studied in the prior literature. In this paper, we introduce the multi-variate backfill problem using COVID-19 as the motivating example. We construct a detailed dataset composed of relevant signals over the past year of the pandemic. We then systematically characterize several patterns in backfill dynamics and leverage our observations for formulating a novel problem and neural framework Back2Future that aims to refines a given model's predictions in real-time. Our extensive experiments demonstrate that our method refines the performance of top models for COVID-19 forecasting, in contrast to non-trivial baselines, yielding 18% improvement over baselines, enabling us obtain a new SOTA performance. In addition, we show that our model improves model evaluation too; hence policy-makers can better understand the true accuracy of forecasting models in real-time.
更多查看译文
关键词
Epidemic Forecasting,Data revisions,Graph Representation learning,Time Series Forecasting
AI 理解论文
溯源树
样例
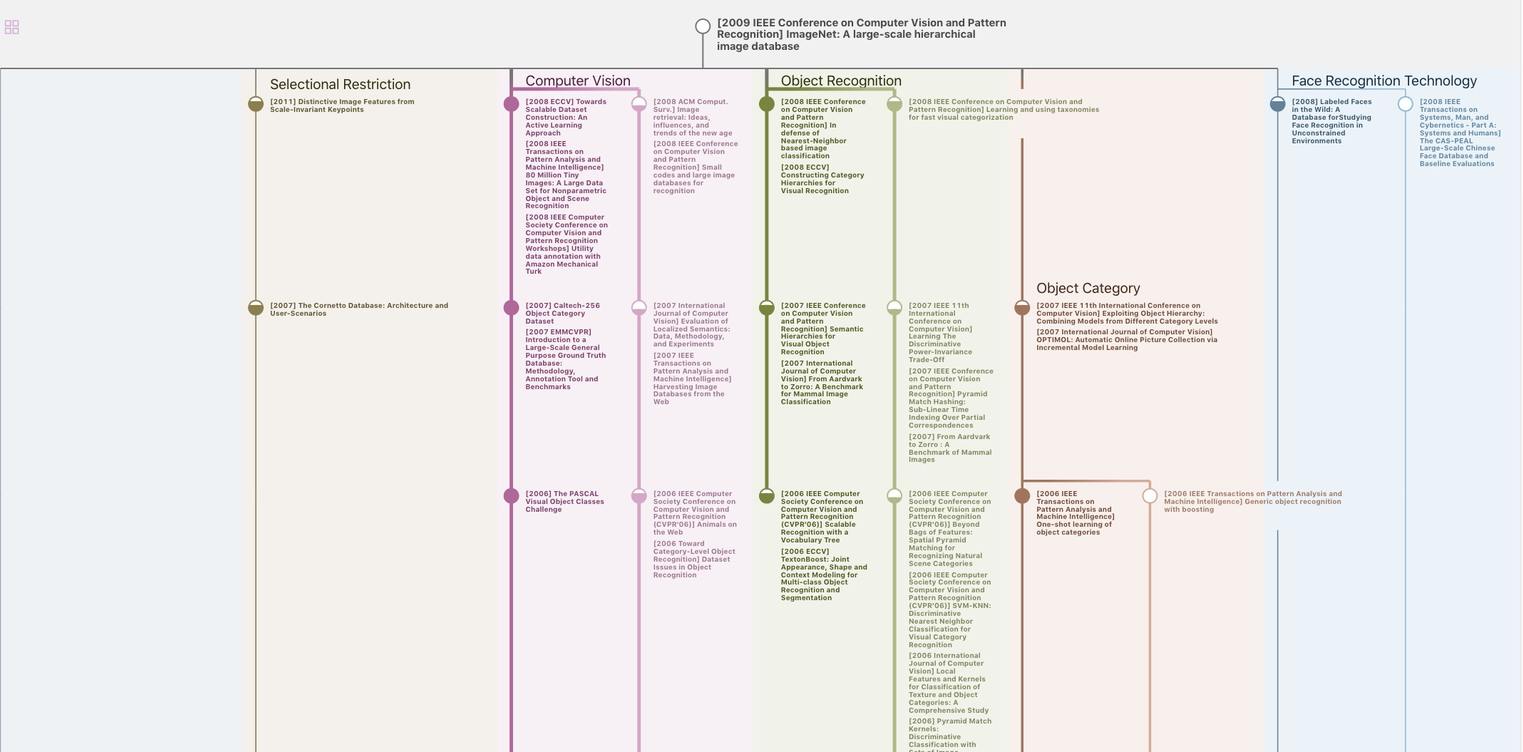
生成溯源树,研究论文发展脉络
Chat Paper
正在生成论文摘要