CSRNet: Cascaded Selective Resolution Network for real-time semantic segmentation
Expert Systems with Applications(2023)
摘要
Real-time semantic segmentation has received considerable attention due to growing demands in many practical applications, such as autonomous vehicles, robotics, etc. Existing real-time segmentation approaches often utilize feature fusion to improve segmentation accuracy. However, they fail to fully consider the feature information at different resolutions and the receptive fields of the networks are relatively limited, thereby compromising the performance. To tackle this problem, we propose a light Cascaded Selective Resolution Network (CSRNet) to improve the performance of real-time segmentation through multiple context information embedding and enhanced feature aggregation. The proposed network builds a three-stage segmentation system, which integrates feature information from low resolution to high resolution and achieves feature refinement progressively. CSRNet contains two critical modules: the Shorted Pyramid Fusion Module (SPFM) and the Selective Resolution Module (SRM). The SPFM is a computationally efficient module to incorporate the global context information and significantly enlarge the receptive field at each stage. The SRM is designed to fuse multi-resolution feature maps with various receptive fields, which assigns soft channel attentions across the feature maps and helps to remedy the problem caused by multi-scale objects. Comprehensive experiments on well-known road scene datasets demonstrate that the proposed CSRNet outperforms the main-stream efficient semantic segmentation approaches by accuracy and can be performed at a fast inference speed.
更多查看译文
关键词
Semantic segmentation,Attention mechanism,Real-time inference,Deep neural networks
AI 理解论文
溯源树
样例
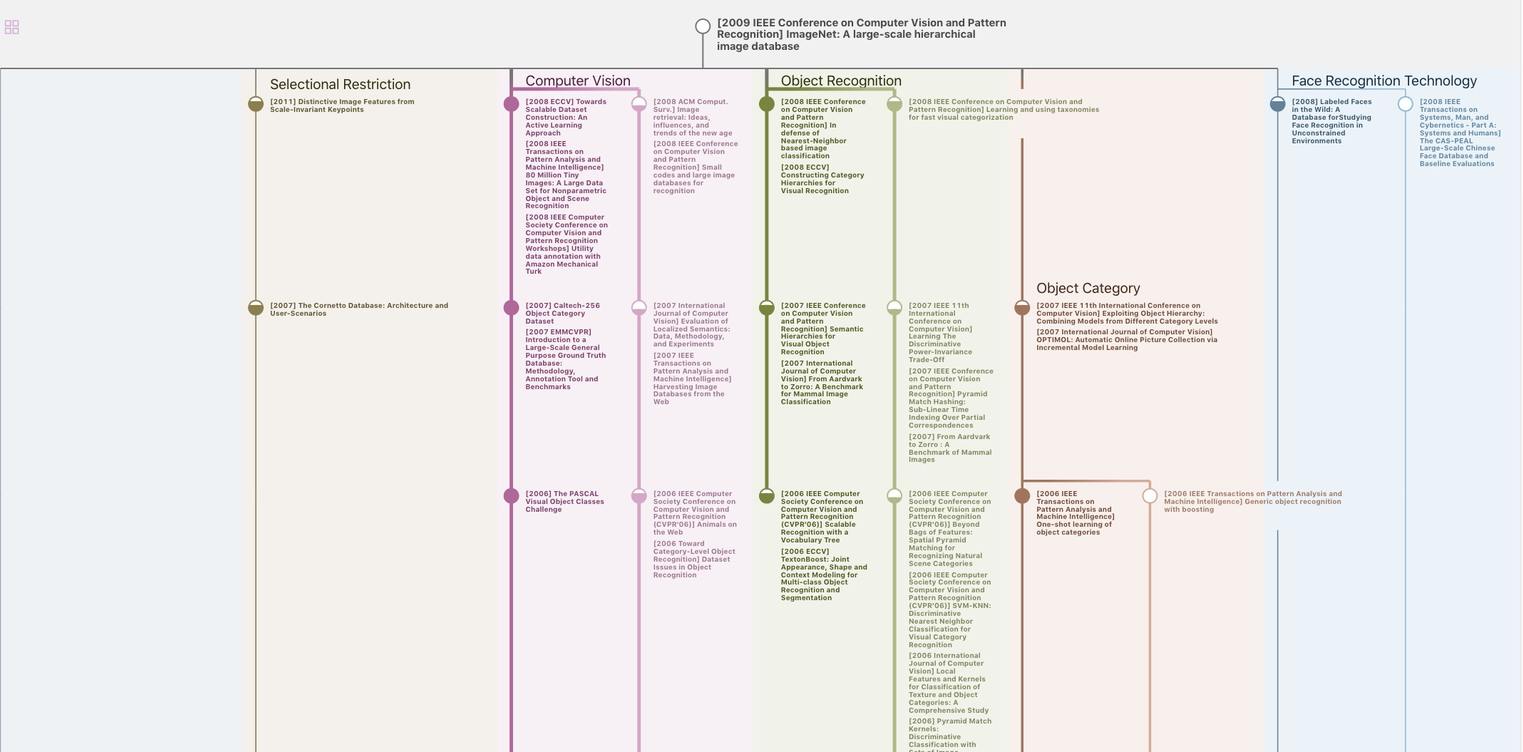
生成溯源树,研究论文发展脉络
Chat Paper
正在生成论文摘要