A structured latent space for human body motion generation
arxiv(2022)
摘要
This work investigates learning a structured latent space to represent and generate temporally and spatially dense 4D human body motion. Once trained, the proposed model generates a multi-frame sequence of dense 3D meshes based on a single point in a low-dimensional latent space. Learning a generative model of human motion with an underlying structured latent space is important for a wide set of applications in computer vision and graphics, including virtual and augmented reality, 3D telepresence, and content generation for entertainment applications. We learn this latent motion representation in a data-driven framework that builds upon two existing lines of works. The first analyzes temporally dense skeletal data to capture the global displacement, poses and temporal evolution of the motion, while the second analyzes static densely captured human scans in 3D to represent realistic 3D human body surfaces in a lowdimensional space. Building upon the respective advantages of these two concepts allows our model to simultaneously represent temporal motion information for sequences of varying duration and detailed 3D geometry at every time instant of the motion. We experimentally demonstrate that the resulting latent space is structured in the sense that similar motions form clusters in this space, and use our latent space to generate plausible interpolations between different actions. We also illustrate the benefits of the approach for 4D human motion completion, showing promising abilities of our model to learn spatio-temporal features of human motion
更多查看译文
AI 理解论文
溯源树
样例
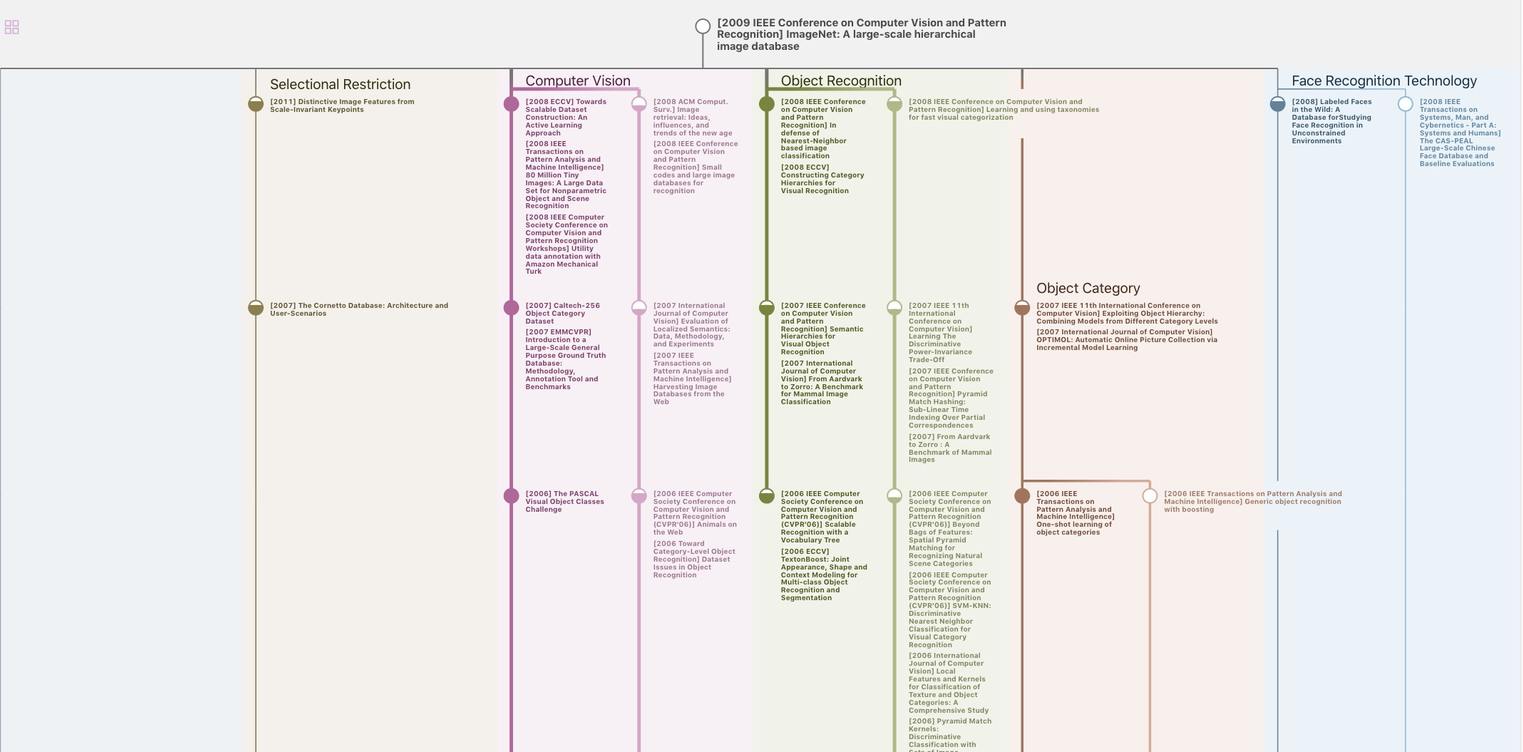
生成溯源树,研究论文发展脉络
Chat Paper
正在生成论文摘要