Efficient Sampling in POMDPs with Lipschitz Bandits for Motion Planning in Continuous Spaces
2021 32ND IEEE INTELLIGENT VEHICLES SYMPOSIUM (IV)(2021)
摘要
Decision making under uncertainty can be framed as a partially observable Markov decision process (POMDP). Finding exact solutions of POMDPs is generally computationally intractable, but the solution can be approximated by sampling-based approaches. These sampling-based POMDP solvers rely on multi-armed bandit (MAB) heuristics, which assume the outcomes of different actions to be uncorrelated. In some applications, like motion planning in continuous spaces, similar actions yield similar outcomes. In this paper, we utilize variants of MAB heuristics that make Lipschitz continuity assumptions on the outcomes of actions to improve the efficiency of sampling-based planning approaches. We demonstrate the effectiveness of this approach in the context of motion planning for automated driving.
更多查看译文
关键词
Lipschitz bandits,motion planning,continuous spaces,partially observable Markov decision process,sampling-based planning approaches,sampling-based POMDP solvers,multiarmed bandit heuristics,MAB heuristics,Lipschitz continuity assumptions,decision making
AI 理解论文
溯源树
样例
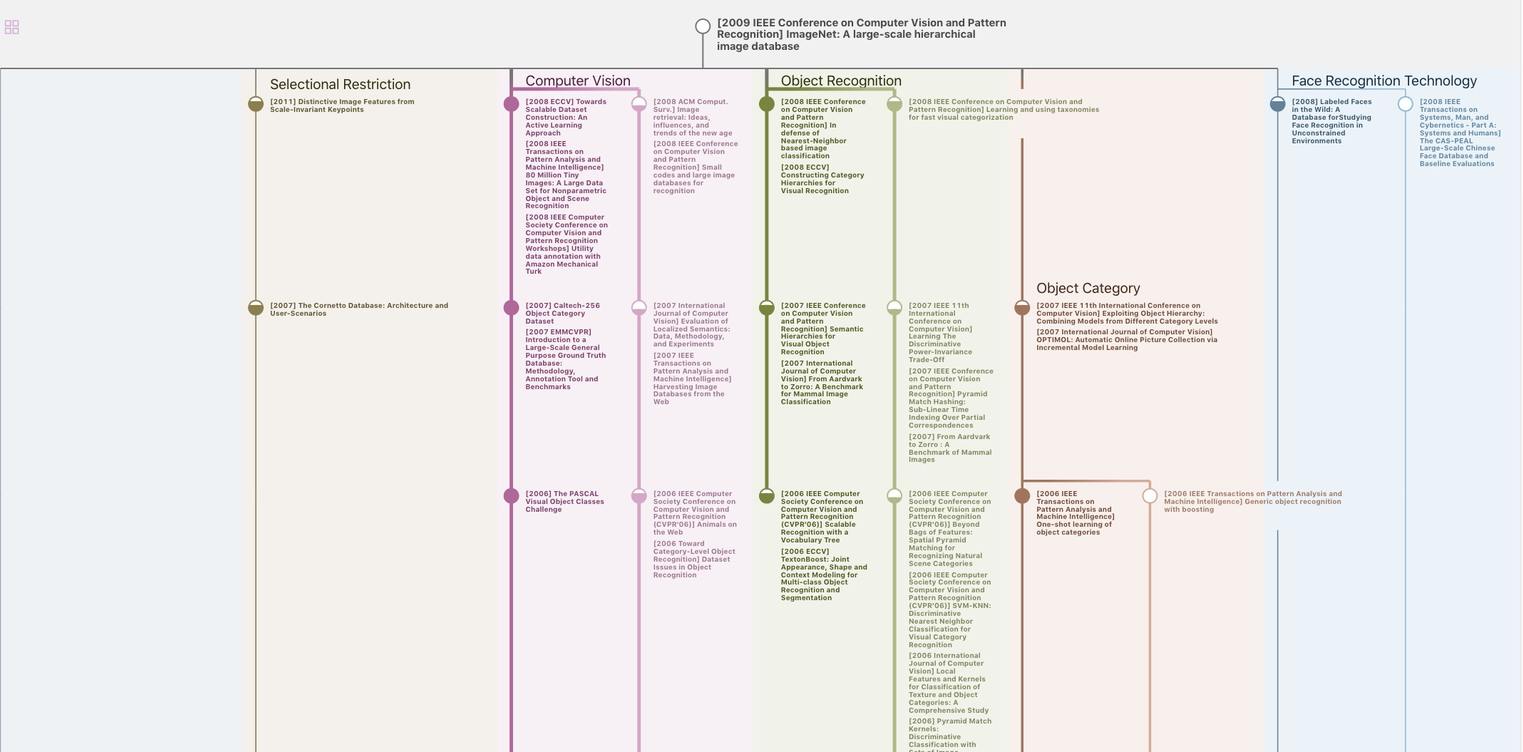
生成溯源树,研究论文发展脉络
Chat Paper
正在生成论文摘要