LipSync3D: Data-Efficient Learning of Personalized 3D Talking Faces from Video using Pose and Lighting Normalization
2021 IEEE/CVF CONFERENCE ON COMPUTER VISION AND PATTERN RECOGNITION, CVPR 2021(2021)
Abstract
In this paper, we present a video-based learning framework for animating personalized 3D talking faces from audio. We introduce two training-time data normalizations that significantly improve data sample efficiency. First, we isolate and represent faces in a normalized space that decouples 3D geometry, head pose, and texture. This decomposes the prediction problem into regressions over the 3D face shape and the corresponding 2D texture atlas. Second, we leverage facial symmetry and approximate albedo constancy of skin to isolate and remove spatio-temporal lighting variations. Together, these normalizations allow simple networks to generate high fidelity lip-sync videos under novel ambient illumination while training with just a single speaker-specific video. Further, to stabilize temporal dynamics, we introduce an auto-regressive approach that conditions the model on its previous visual state. Human ratings and objective metrics demonstrate that our method outperforms contemporary state-of-the-art audio-driven video reenactment benchmarks in terms of realism, lip-sync and visual quality scores. We illustrate several applications enabled by our framework.
MoreTranslated text
Key words
LipSync3D,data-efficient learning,personalized 3D talking faces,video using pose,lighting normalization,video-based,training-time data normalizations,data sample efficiency,normalized space,prediction problem,3D face shape,leverage facial symmetry,spatio-temporal lighting variations,high fidelity lip-sync videos,single speaker-specific video,auto-regressive approach,contemporary state-of-the-art audio-driven video reenactment benchmarks
AI Read Science
Must-Reading Tree
Example
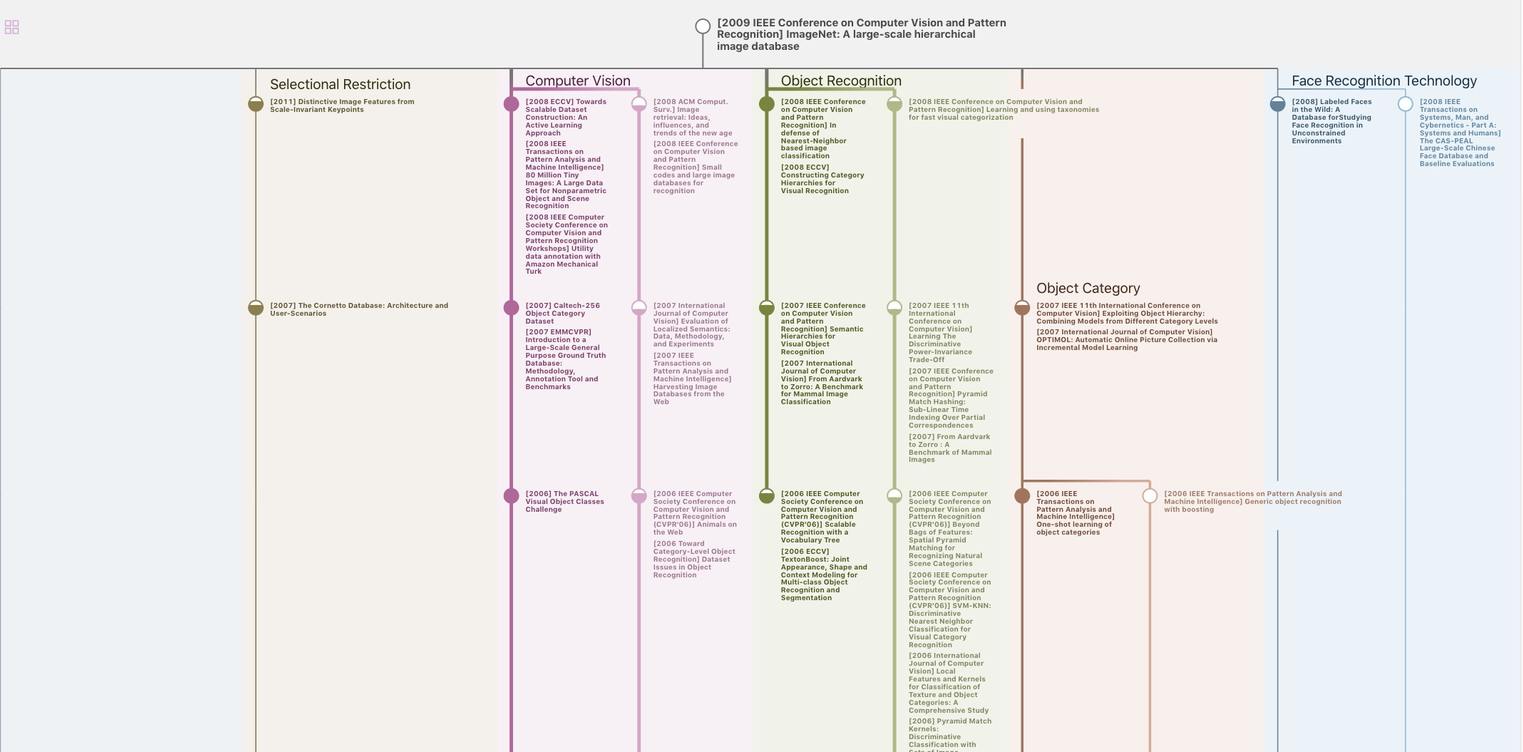
Generate MRT to find the research sequence of this paper
Chat Paper
Summary is being generated by the instructions you defined