Dilated Convolution Based CSI Feedback Compression for Massive MIMO Systems
IEEE Transactions on Vehicular Technology(2022)
摘要
Although the frequency-division duplex (FDD) massive multiple-input multiple-output (MIMO) system can offer high spectral and energy efficiency, it requires to feedback the downlink channel state information (CSI) from users to the base station (BS), in order to fulfill the precoding design at the BS. However, the large dimension of CSI matrices in the massive MIMO system makes the CSI feedback very challenging, and it is urgent to compress the feedback CSI. To this end, this paper proposes a novel dilated convolution based CSI feedback network, namely
D
ilated
C
hannel
R
econstruction
Net
work (DCRNet). Specifically, the dilated convolutions are used to enhance the receptive field (RecF) of the proposed DCRNet without increasing the convolution size. Moreover, advanced encoder and decoder blocks are designed to improve the reconstruction performance and reduce computational complexity as well. Numerical results are presented to show the superiority of the proposed DCRNet over the conventional networks. In particular, compared to the state-of-the-arts (SOTA) networks, the proposed DCRNet can achieve almost the same performance while reduce floating point operations (FLOPs) by about 30%.
更多查看译文
关键词
CSI feedback,deep learning,dilated convolutions,massive MIMO
AI 理解论文
溯源树
样例
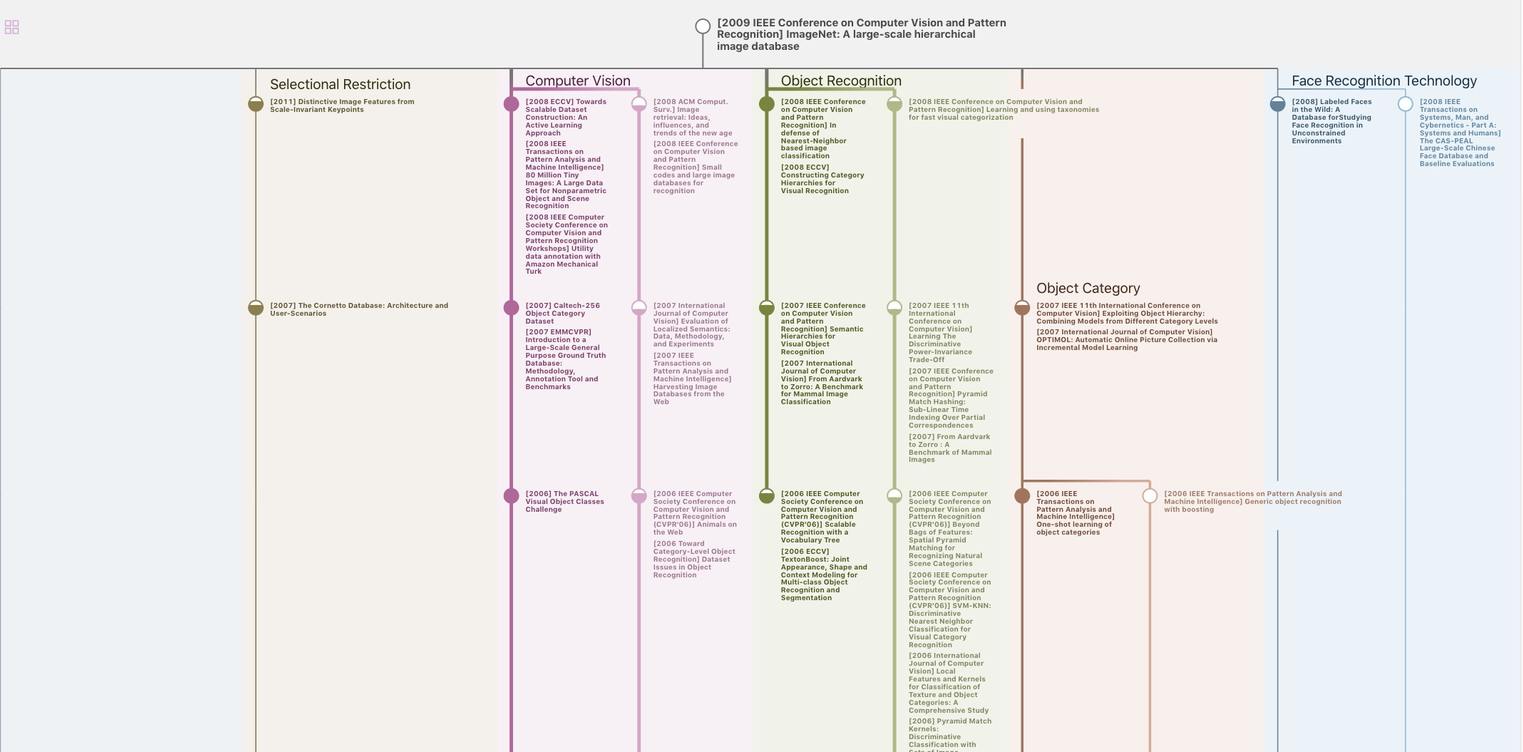
生成溯源树,研究论文发展脉络
Chat Paper
正在生成论文摘要