Learning Correlated Communication Topology in Multi-Agent Reinforcement learning.
AAMAS(2021)
摘要
Communication improves the efficiency and convergence of multi-agent learning. Existing study of agent communication has been limited on predefined fixed connections. While an attention mechanism exists and is useful for scheduling the communication between agents, it, however, largely ignores the dynamical nature of communication and thus the correlation between agents' connections. In this work, we adopt a normalizing flow to encode correlation between agents interactions. The dynamical communication topology is directly learned by maximizing the agent rewards. In our end-to-end formulation, the communication structure is learned by considering it as a hidden dynamical variable. We realize centralized training of critics and graph reasoning policy, and decentralized execution from local observation and message that are received through the learned dynamical communication topology. Experiments on cooperative navigation in the particle world and adaptive traffic control tasks demonstrate the effectiveness of our method.
更多查看译文
关键词
correlated communication topology,reinforcement,learning,multi-agent
AI 理解论文
溯源树
样例
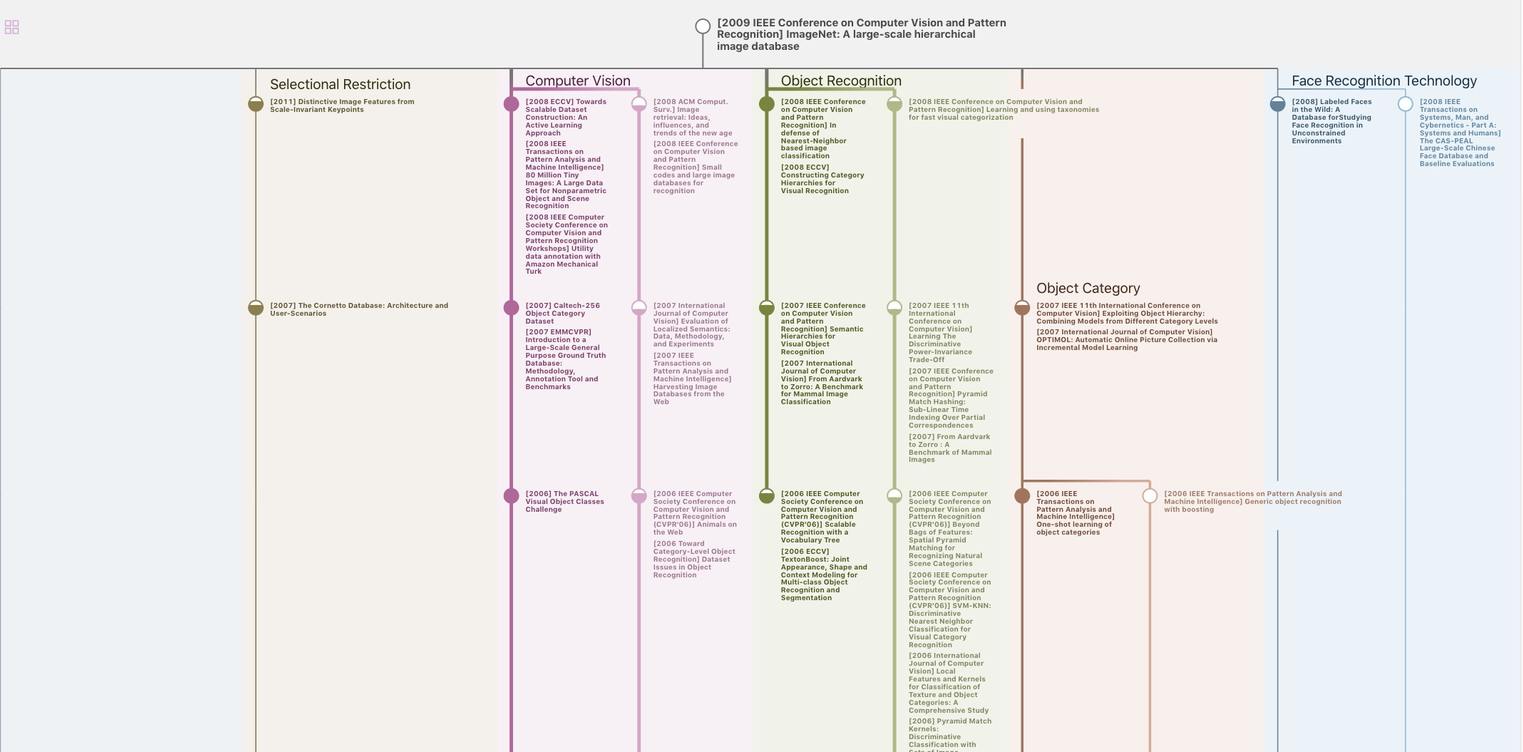
生成溯源树,研究论文发展脉络
Chat Paper
正在生成论文摘要