Graph Attention Autoencoder for Collaborative Pair-wise Ranking
PROCEEDINGS OF THE 2021 IEEE 24TH INTERNATIONAL CONFERENCE ON COMPUTER SUPPORTED COOPERATIVE WORK IN DESIGN (CSCWD)(2021)
Abstract
Recently, top-k recommendation system is getting more and more attention from researchers and unlike the rating prediction task, the purpose of top-k recommendation is to present the user with a list of items they are most interested in. Many rating prediction models do not produce good ranking results, so the top-k recommendation faces two challenges: the first is how to accurately obtain user and item latent vector from the user-item interaction rating matrix, and the second challenge is how to combine the ranking strategy with the recommendation model deeply. The booming deep learning technology, such as autoencoder and graph neural networks, brings us new solutions. So in this paper, we propose a novel model: Graph Attention Autoencoder for Collaborative Pair-wise Ranking. The autoencoder consists of graph attention encoder and collaborative neural decoder, which is used to generate user and item latent vector accurately. And then we use the pairwise ranking learning process to ensure the rating value accuracy and rating value pairwise ranking consistency. Finally, experimental results on three real-world datasets demonstrate the superiority of our model.
MoreTranslated text
Key words
Graph Attention Autoencoder, Pair-wise Ranking, Multi-head Attention, Top-K Recommendation
AI Read Science
Must-Reading Tree
Example
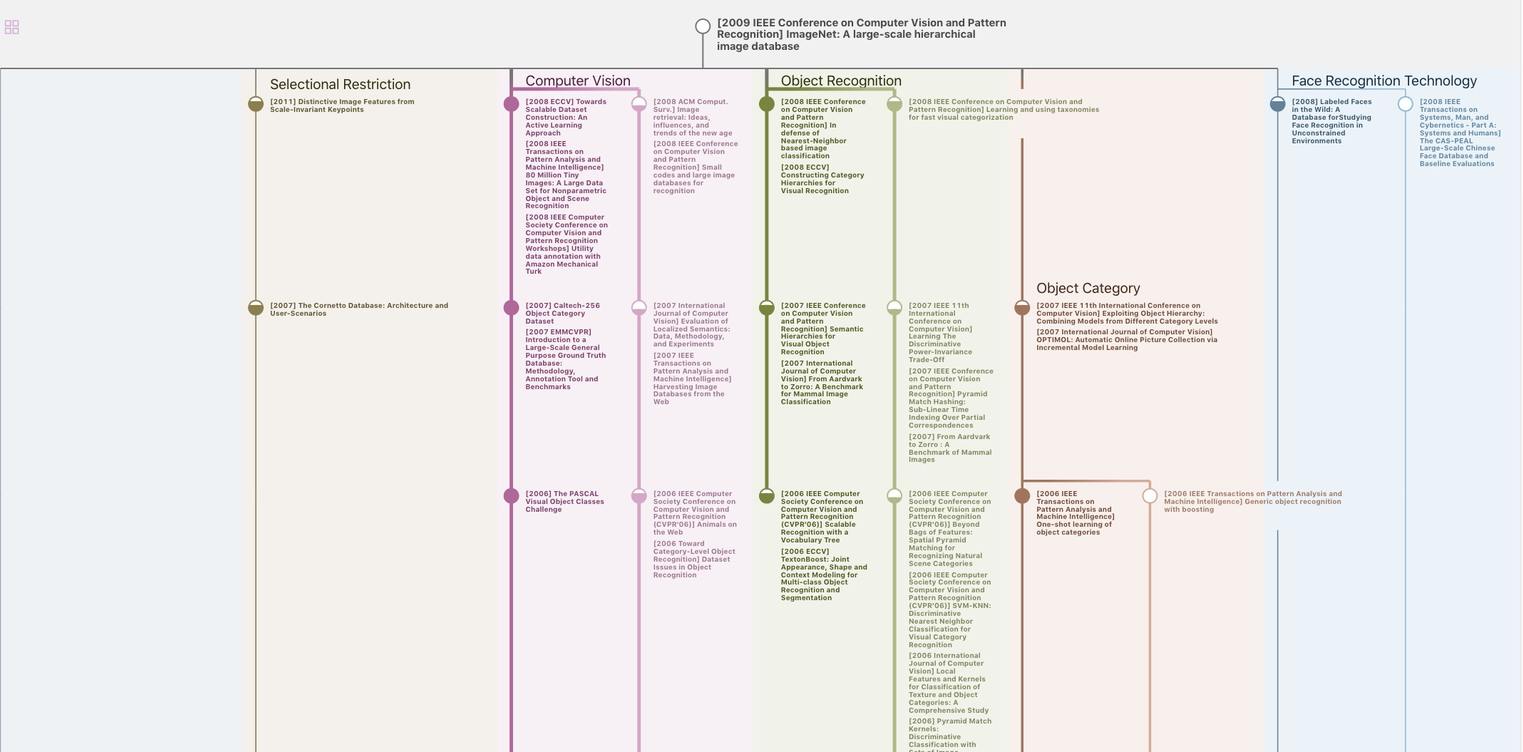
Generate MRT to find the research sequence of this paper
Chat Paper
Summary is being generated by the instructions you defined