Generative Adversarial Network-Based Synthetic Seizure Dataset Augmentation
2021 10TH INTERNATIONAL IEEE/EMBS CONFERENCE ON NEURAL ENGINEERING (NER)(2021)
Abstract
Electroencephalography (EEG) datasets can be used to train machine learning classifiers for personalized implantable seizure prevention devices. These devices apply electrical stimuli to prevent seizures from propagating in the brain, but excessive stimulation caused by a high false positive rate (FPR) can cause side effects and require more frequent battery replacement surgeries. Reducing the FPR requires vast and balanced training sets, but seizures only comprise <1% of clinically recorded EEG data. In this work, we present a method of generating multi-channel seizure data with a Wasserstein Generative Adversarial Network. This approach is demonstrated to reduce the false positive rate by 72.72%, significantly reducing the frequency of erroneously applied electrical stimuli that could degrade patients' quality of life. This is achieved without changing the algorithm implemented on neurostimulators and only slightly increasing detection latency while maintaining 100% seizure sensitivity.
MoreTranslated text
Key words
balanced training sets,clinically recorded EEG data,multichannel seizure data,Wasserstein generative adversarial network,electroencephalography datasets,personalized implantable seizure prevention devices,false positive rate,FPR,applied electrical stimuli,seizure sensitivity,generative adversarial network-based synthetic seizure dataset augmentation,quality-of-life,neurostimulators,machine learning classifiers
AI Read Science
Must-Reading Tree
Example
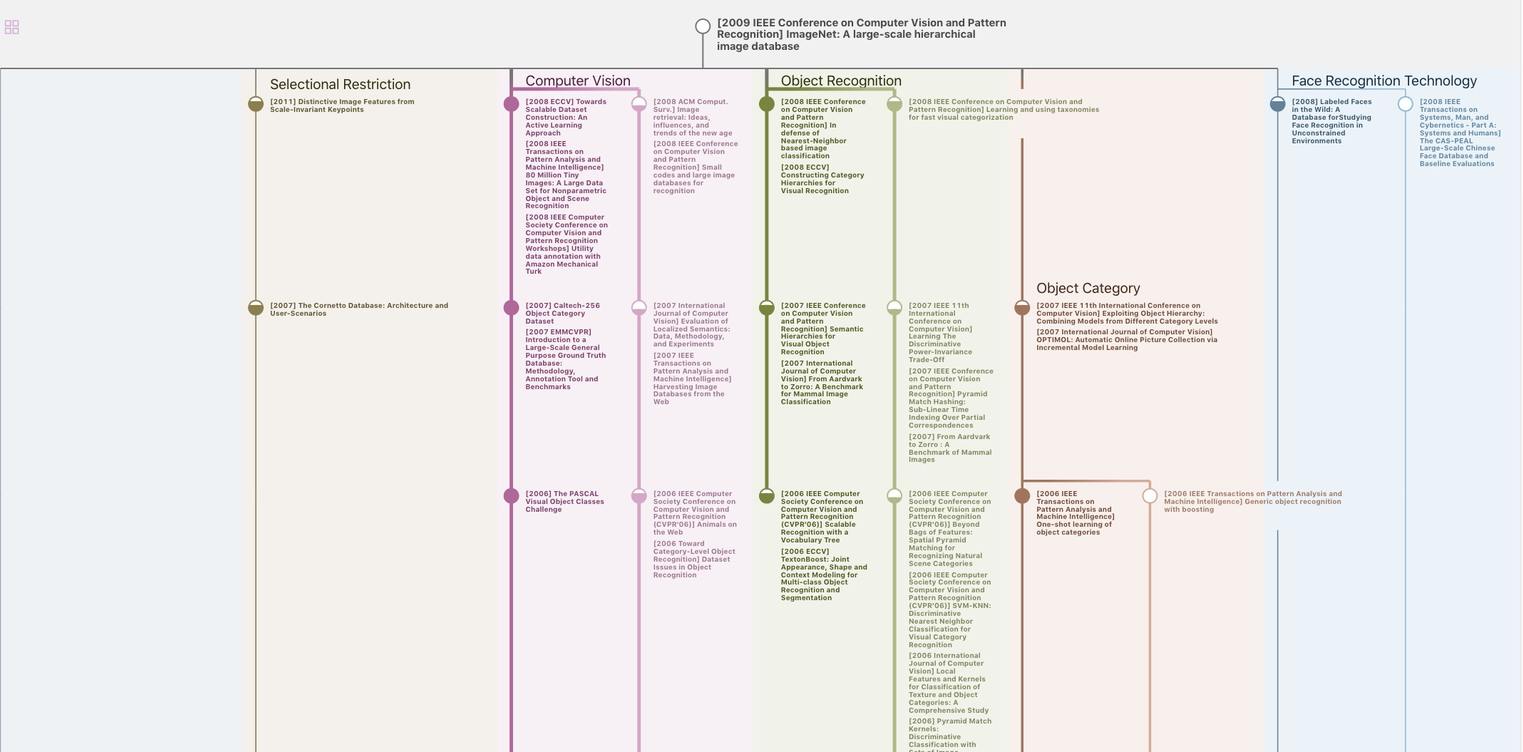
Generate MRT to find the research sequence of this paper
Chat Paper
Summary is being generated by the instructions you defined