One-shot learning of paired association navigation with biologically plausible schemas
arxiv(2023)
摘要
Schemas are knowledge structures that can enable one-shot learning. Rodent one-shot learning in a multiple paired association navigation task has been postulated to be schema-dependent. However, the correspondence between schemas and neural implementations remains poorly understood, and a biologically plausible computational model of the rodents learning has not been demonstrated. Here, we compose such an agent from schemas with biologically plausible neural implementations. The agent contains an associative memory that can form one-shot associations between sensory cues and goal coordinates, implemented using a network with either a feedforward layer or a reservoir of recurrently connected neurons whose plastic output weights are governed by a novel 4-factor reward modulated Exploratory Hebbian (EH) rule. Adding an actor-critic allows the agent to succeed even if obstacles prevent direct heading. With the addition of working memory, the rodent behavior is replicated. Temporal-difference learning of a working memory gate enables one-shot learning despite distractors.
更多查看译文
关键词
association navigation,learning,one-shot
AI 理解论文
溯源树
样例
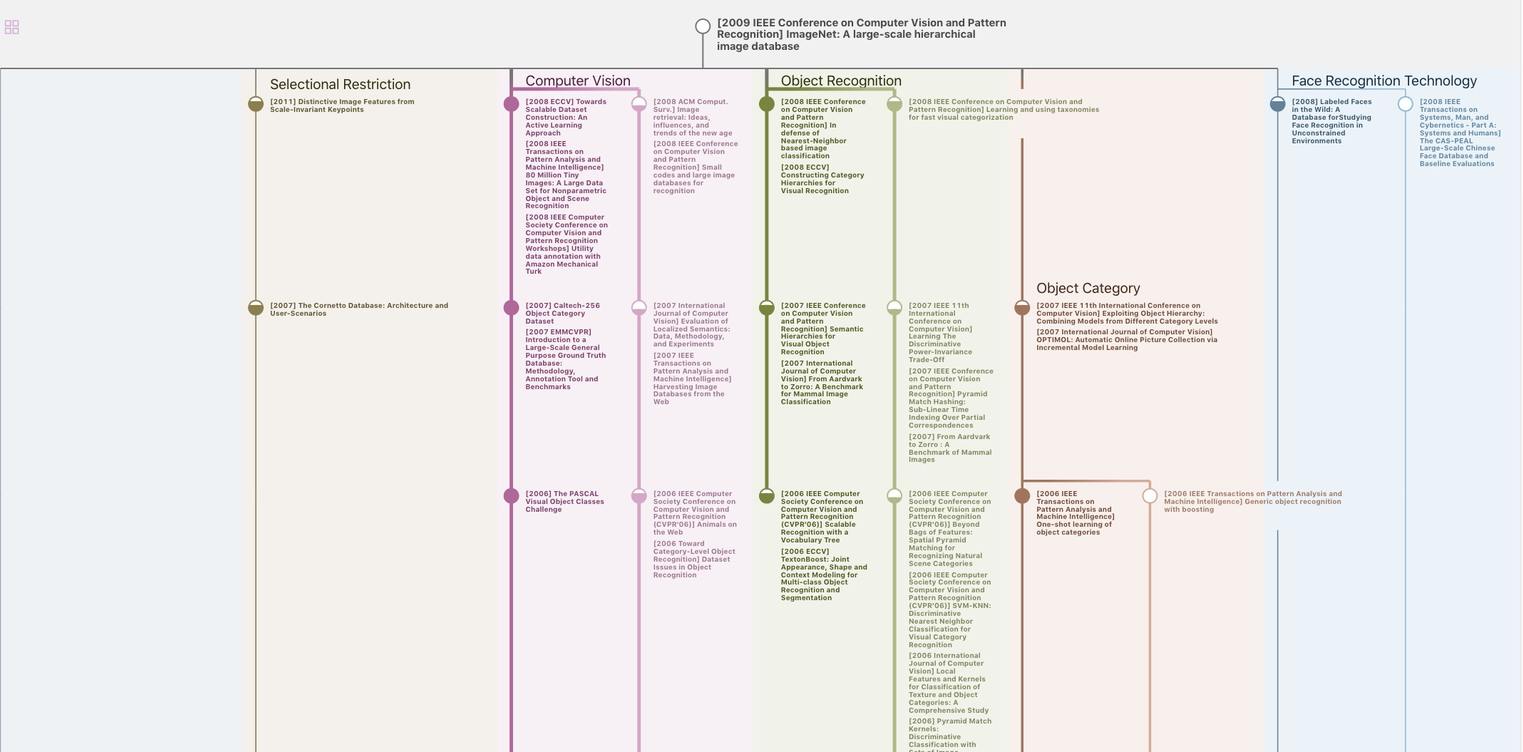
生成溯源树,研究论文发展脉络
Chat Paper
正在生成论文摘要