On the Design of Strategic Task Recommendations for Sustainable Crowdsourcing-Based Content Moderation
arxiv(2021)
摘要
Crowdsourcing-based content moderation is a platform that hosts content moderation tasks for crowd workers to review user submissions (e.g. text, images and videos) and make decisions regarding the admissibility of the posted content, along with a gamut of other tasks such as image labeling and speech-to-text conversion. In an attempt to reduce cognitive overload at the workers and improve system efficiency, these platforms offer personalized task recommendations according to the worker's preferences. However, the current state-of-the-art recommendation systems disregard the effects on worker's mental health, especially when they are repeatedly exposed to content moderation tasks with extreme content (e.g. violent images, hate-speech). In this paper, we propose a novel, strategic recommendation system for the crowdsourcing platform that recommends jobs based on worker's mental status. Specifically, this paper models interaction between the crowdsourcing platform's recommendation system (leader) and the worker (follower) as a Bayesian Stackelberg game where the type of the follower corresponds to the worker's cognitive atrophy rate and task preferences. We discuss how rewards and costs should be designed to steer the game towards desired outcomes in terms of maximizing the platform's productivity, while simultaneously improving the working conditions of crowd workers.
更多查看译文
关键词
strategic task recommendations,content,crowdsourcing-based
AI 理解论文
溯源树
样例
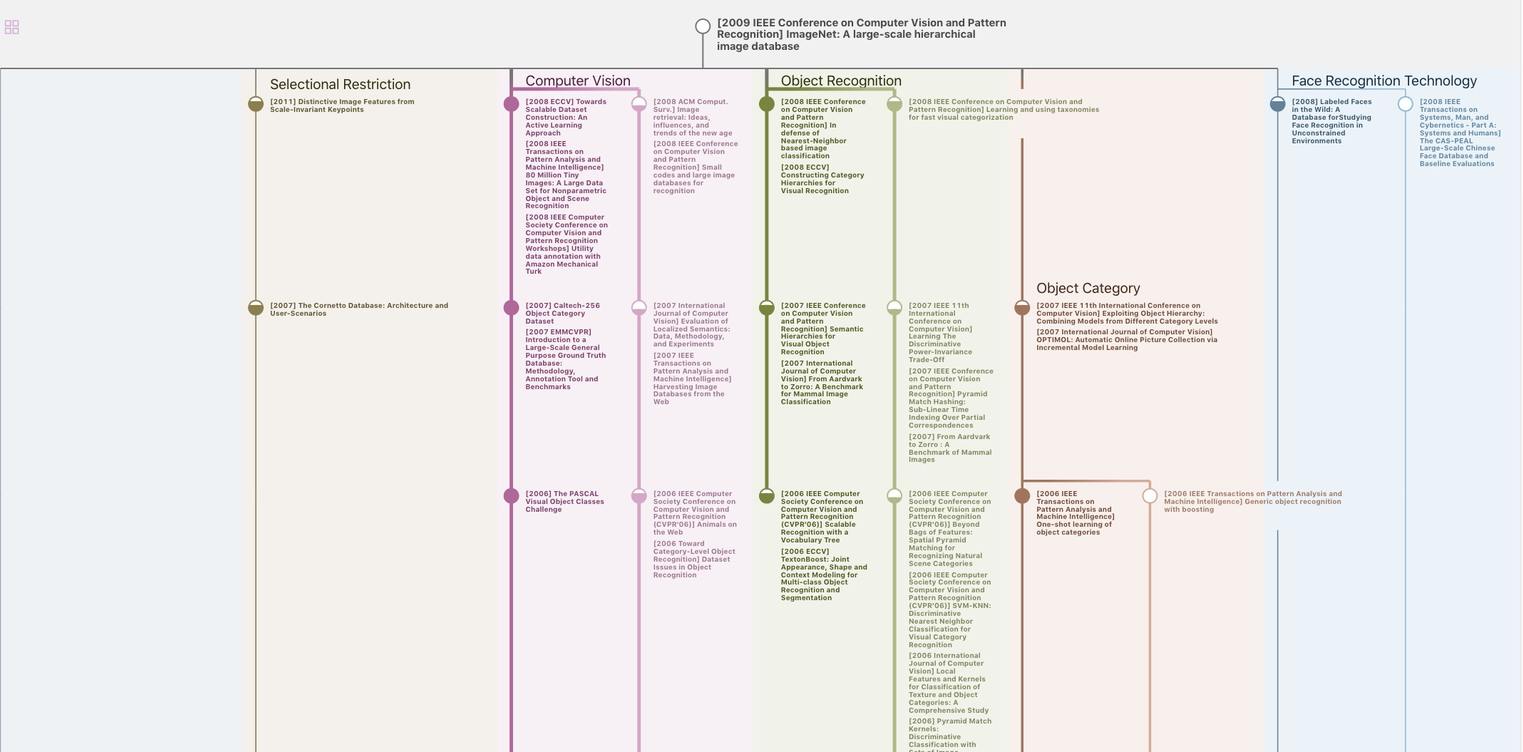
生成溯源树,研究论文发展脉络
Chat Paper
正在生成论文摘要