Understanding and Improving Fairness-Accuracy Trade-offs in Multi-Task Learning
Knowledge Discovery and Data Mining(2021)
摘要
ABSTRACTAs multi-task models gain popularity in a wider range of machine learning applications, it is becoming increasingly important for practitioners to understand the fairness implications associated with those models. Most existing fairness literature focuses on learning a single task more fairly, while how ML fairness interacts with multiple tasks in the joint learning setting is largely under-explored. In this paper, we are concerned with how group fairness (e.g., equal opportunity, equalized odds) as an ML fairness concept plays out in the multi-task scenario. In multi-task learning, several tasks are learned jointly to exploit task correlations for a more efficient inductive transfer. This presents a multi-dimensional Pareto frontier on (1) the trade-off between group fairness and accuracy with respect to each task, as well as (2) the trade-offs across multiple tasks. We aim to provide a deeper understanding on how group fairness interacts with accuracy in multi-task learning, and we show that traditional approaches that mainly focus on optimizing the Pareto frontier of multi-task accuracy might not perform well on fairness goals. We propose a new set of metrics to better capture the multi-dimensional Pareto frontier of fairness-accuracy trade-offs uniquely presented in a multi-task learning setting. We further propose a Multi-Task-Aware Fairness (MTA-F) approach to improve fairness in multi-task learning. Experiments on several real-world datasets demonstrate the effectiveness of our proposed approach.
更多查看译文
关键词
fairness, multi-task learning, Pareto frontier, multi-task-aware fairness treatment
AI 理解论文
溯源树
样例
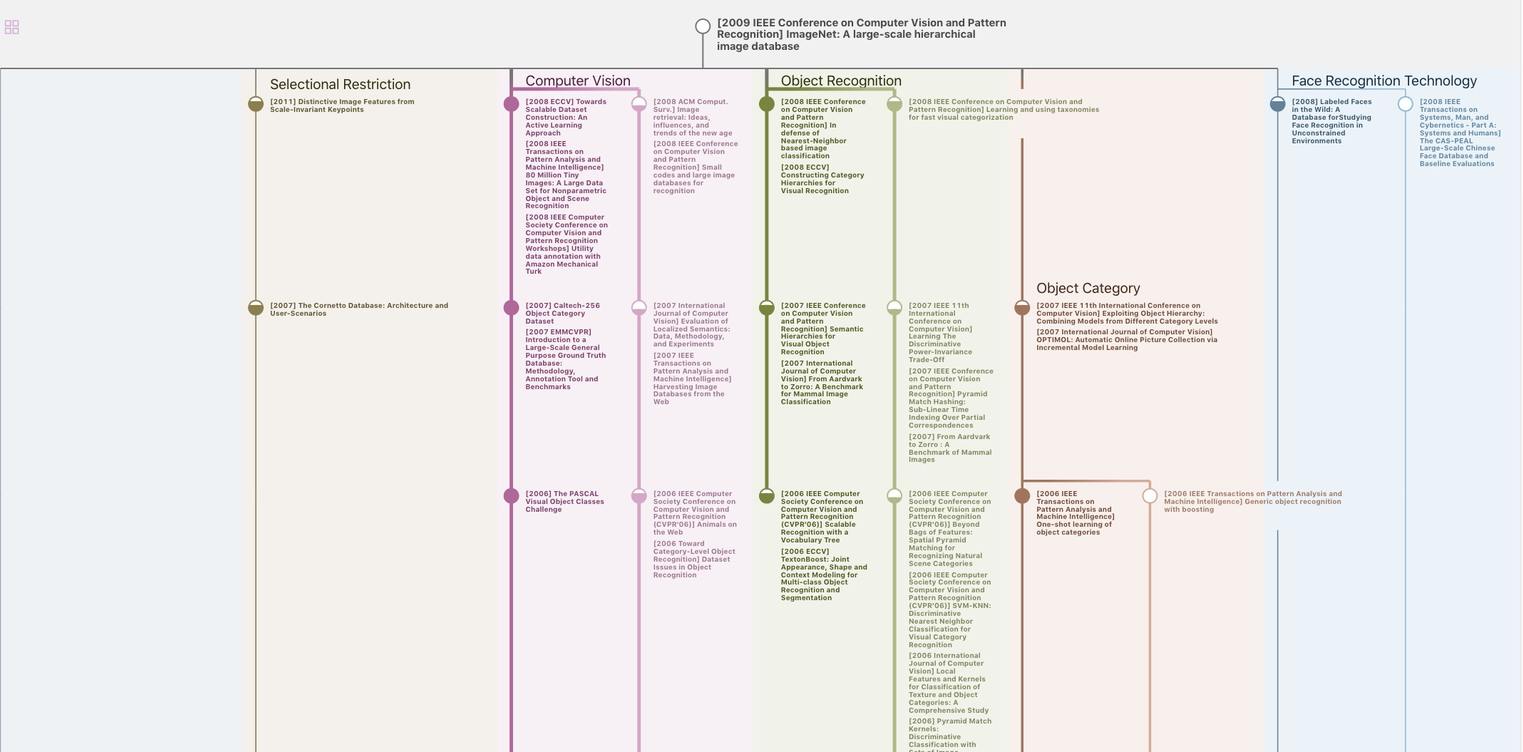
生成溯源树,研究论文发展脉络
Chat Paper
正在生成论文摘要