KGPool - Dynamic Knowledge Graph Context Selection for Relation Extraction.
ACL/IJCNLP(2021)
摘要
We present a novel method for relation extraction (RE) from a single sentence, mapping the sentence and two given entities to a canonical fact in a knowledge graph (KG). Especially in this presumed sentential RE setting, the context of a single sentence is often sparse. This paper introduces the KGPool method to address this sparsity, dynamically expanding the context with additional facts from the KG. It learns the representation of these facts (entity alias, entity descriptions, etc.) using neural methods, supplementing the sentential context. Unlike existing methods that statically use all expanded facts, KGPool conditions this expansion on the sentence. We study the efficacy of KGPool by evaluating it with different neural models and KGs (Wikidata and NYT Freebase). Our experimental evaluation on standard datasets shows that by feeding the KGPool representation into a Graph Neural Network, the overall method is significantly more accurate than state-of-the-art methods.
更多查看译文
关键词
relation,context,knowledge
AI 理解论文
溯源树
样例
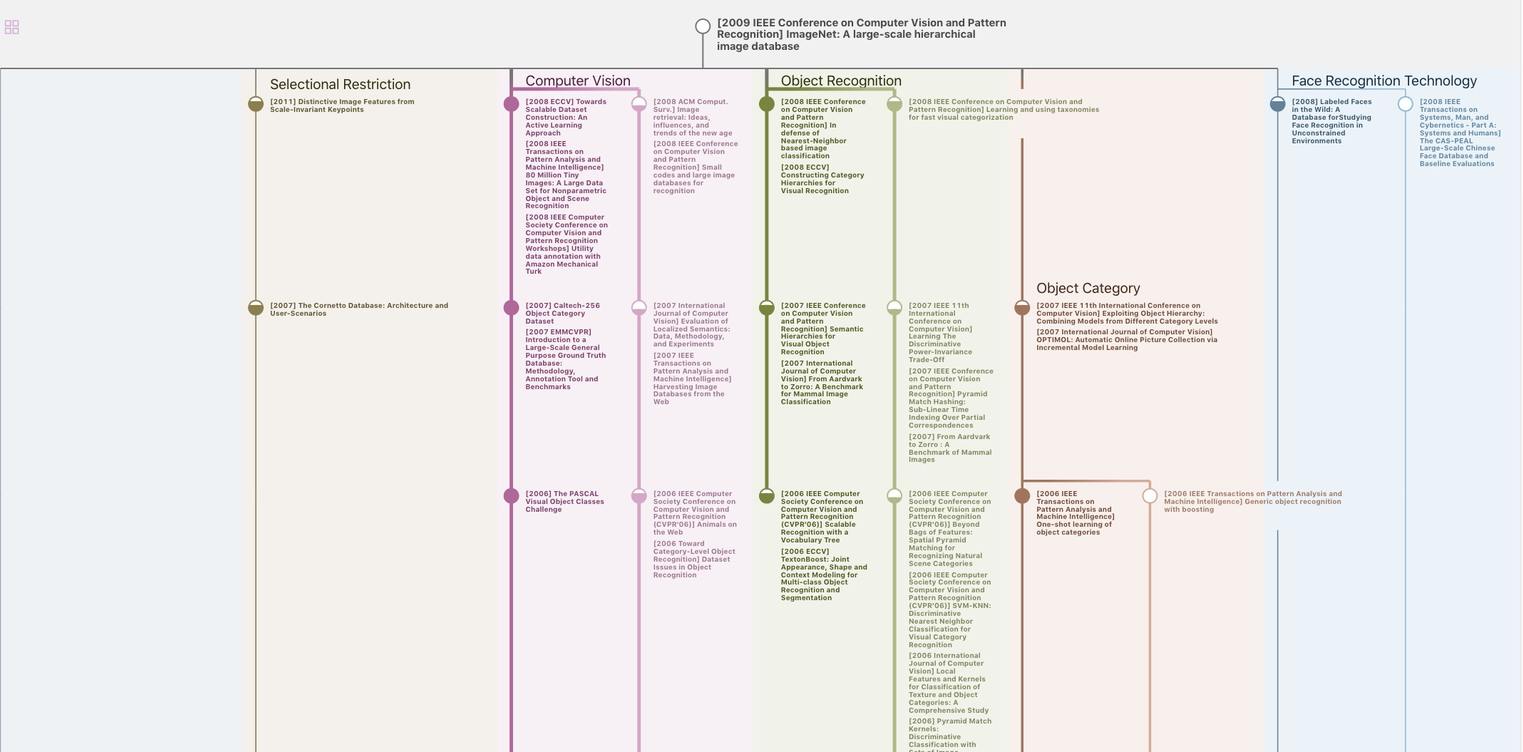
生成溯源树,研究论文发展脉络
Chat Paper
正在生成论文摘要