Forward Super-Resolution: How Can GANs Learn Hierarchical Generative Models for Real-World Distributions
ICLR 2023(2023)
摘要
Generative adversarial networks (GANs) are among the most successful models for learning high-complexity, real-world distributions. However, in theory, due to the highly non-convex, non-concave landscape of the minmax training objective, GAN remains one of the least understood deep learning models. In this work, we formally study how GANs can efficiently learn certain hierarchically generated distributions that are close to the distribution of real-life images. We prove that when a distribution has a structure that we refer to as Forward Super-Resolution, then simply training generative adversarial networks using stochastic gradient descent ascent (SGDA) can learn this distribution efficiently, both in sample and time complexities. We also provide empirical evidence that our assumption "forward super-resolution" is very natural in practice, and the underlying learning mechanisms that we study in this paper (to allow us efficiently train GAN via SGDA in theory) simulates the actual learning process of GANs on real-world problems.
更多查看译文
关键词
hierarchical generative,gans,models,real-world real-world,super-resolution
AI 理解论文
溯源树
样例
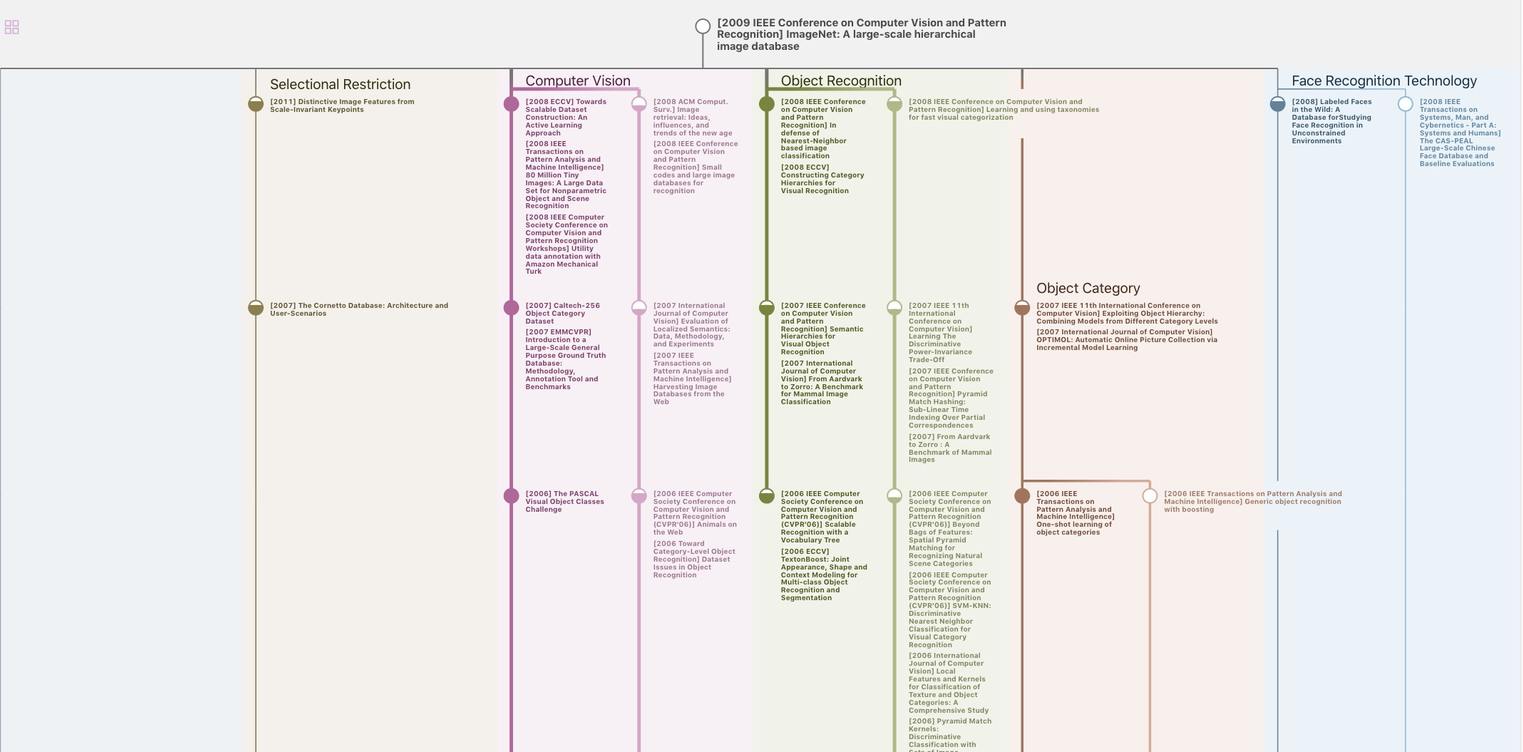
生成溯源树,研究论文发展脉络
Chat Paper
正在生成论文摘要