Price graphs: Utilizing the structural information of financial time series for stock prediction
Information Sciences(2022)
摘要
Great research efforts have been devoted to exploiting deep neural networks in stock prediction. However, long-term dependencies and chaotic properties are still two major issues that lower the performance of state-of-the-art deep learning models in forecasting future price trends. In this study, we propose a novel framework to address both issues. Specifically, in terms of transforming time series into complex networks, we convert market price series into graphs. Then, structural information, referring to temporal point associations and node weights, is extracted from the mapped graphs to resolve the problems regarding long-term dependencies and chaotic properties. We take graph embeddings to represent the associations among temporal points as the prediction model inputs. Node weights are used as a priori knowledge to enhance the learning of temporal attention. The effectiveness of our proposed framework is validated using real-world stock data, and our approach obtains the best performance among several state-of-the-art benchmarks. Moreover, in the conducted trading simulations, our framework further obtains the highest cumulative profits. Our results supplement the existing applications of complex network methods in the financial realm and provide insightful implications for investment applications regarding decision support in financial markets.
更多查看译文
关键词
Stock prediction,Complex network,Time series graph,Graph embedding,Structure information
AI 理解论文
溯源树
样例
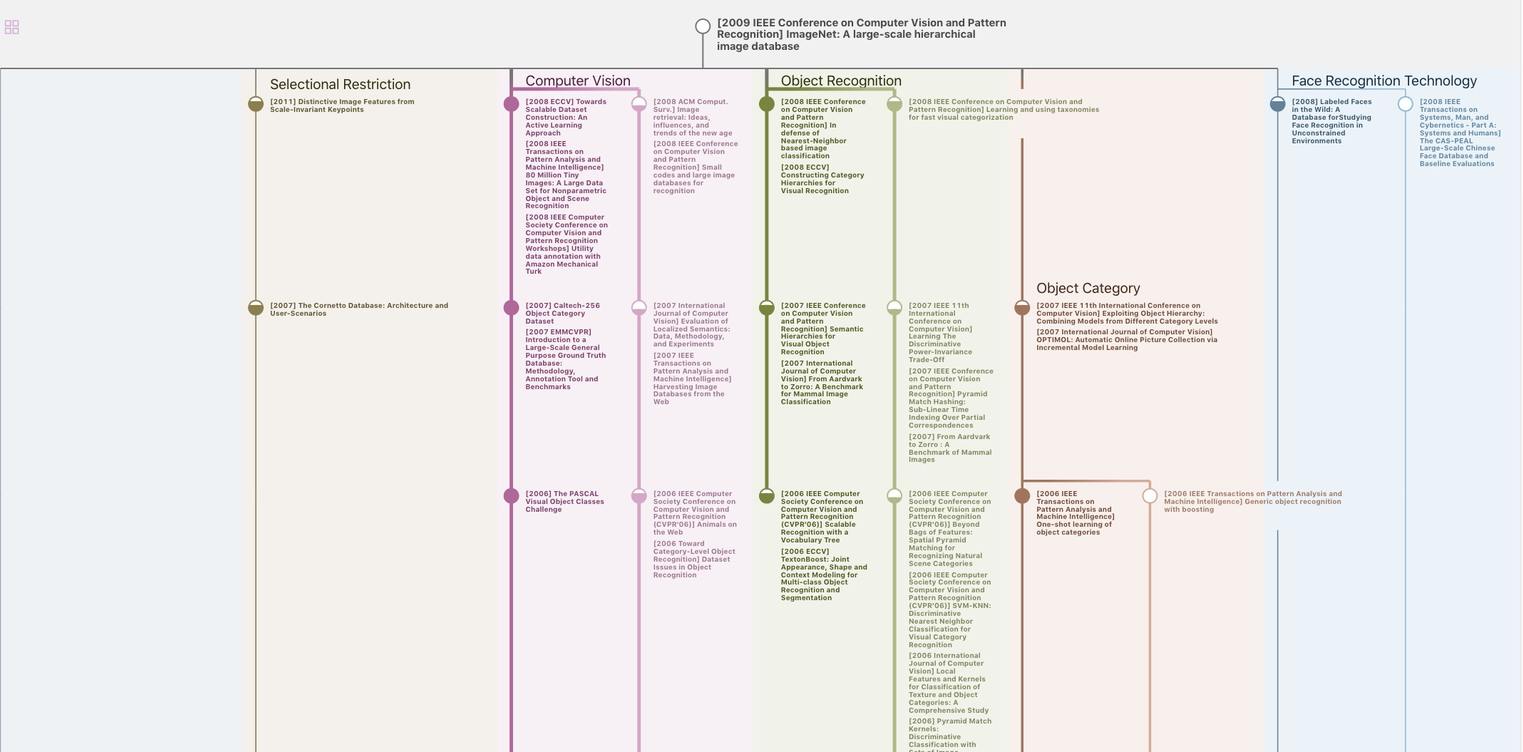
生成溯源树,研究论文发展脉络
Chat Paper
正在生成论文摘要