Provably Strict Generalisation Benefit for Invariance in Kernel Methods.
Annual Conference on Neural Information Processing Systems(2021)
摘要
It is a commonly held belief that enforcing invariance improves generalisation. Although this approach enjoys widespread popularity, it is only very recently that a rigorous theoretical demonstration of this benefit has been established. In this work we build on the function space perspective of Elesedy and Zaidi [8] to derive a strictly non-zero generalisation benefit of incorporating invariance in kernel ridge regression when the target is invariant to the action of a compact group. We study invariance enforced by feature averaging and find that generalisation is governed by a notion of effective dimension that arises from the interplay between the kernel and the group. In building towards this result, we find that the action of the group induces an orthogonal decomposition of both the reproducing kernel Hilbert space and its kernel, which may be of interest in its own right.
更多查看译文
关键词
strict generalisation benefit,invariance,methods
AI 理解论文
溯源树
样例
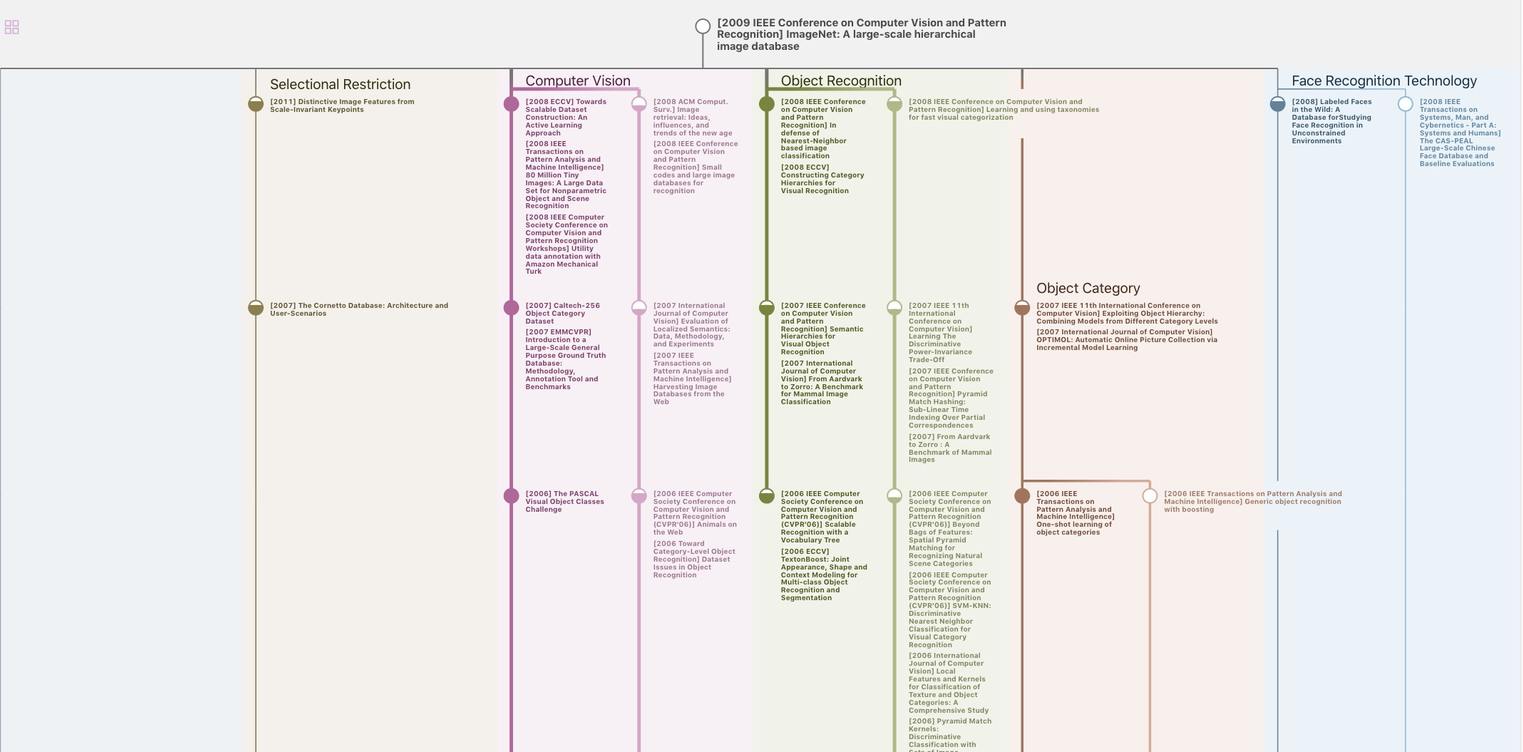
生成溯源树,研究论文发展脉络
Chat Paper
正在生成论文摘要