Robustifying Reinforcement Learning Policies with $\mathcal{L}_1$ Adaptive Control
arXiv (Cornell University)(2021)
Abstract
A reinforcement learning (RL) policy trained in a nominal environment could fail in a new/perturbed environment due to the existence of dynamic variations. Existing robust methods try to obtain a fixed policy for all envisioned dynamic variation scenarios through robust or adversarial training. These methods could lead to conservative performance due to emphasis on the worst case, and often involve tedious modifications to the training environment. We propose an approach to robustifying a pre-trained non-robust RL policy with $\mathcal{L}_1$ adaptive control. Leveraging the capability of an $\mathcal{L}_1$ control law in the fast estimation of and active compensation for dynamic variations, our approach can significantly improve the robustness of an RL policy trained in a standard (i.e., non-robust) way, either in a simulator or in the real world. Numerical experiments are provided to validate the efficacy of the proposed approach.
MoreTranslated text
Key words
reinforcement learning policies,control
AI Read Science
Must-Reading Tree
Example
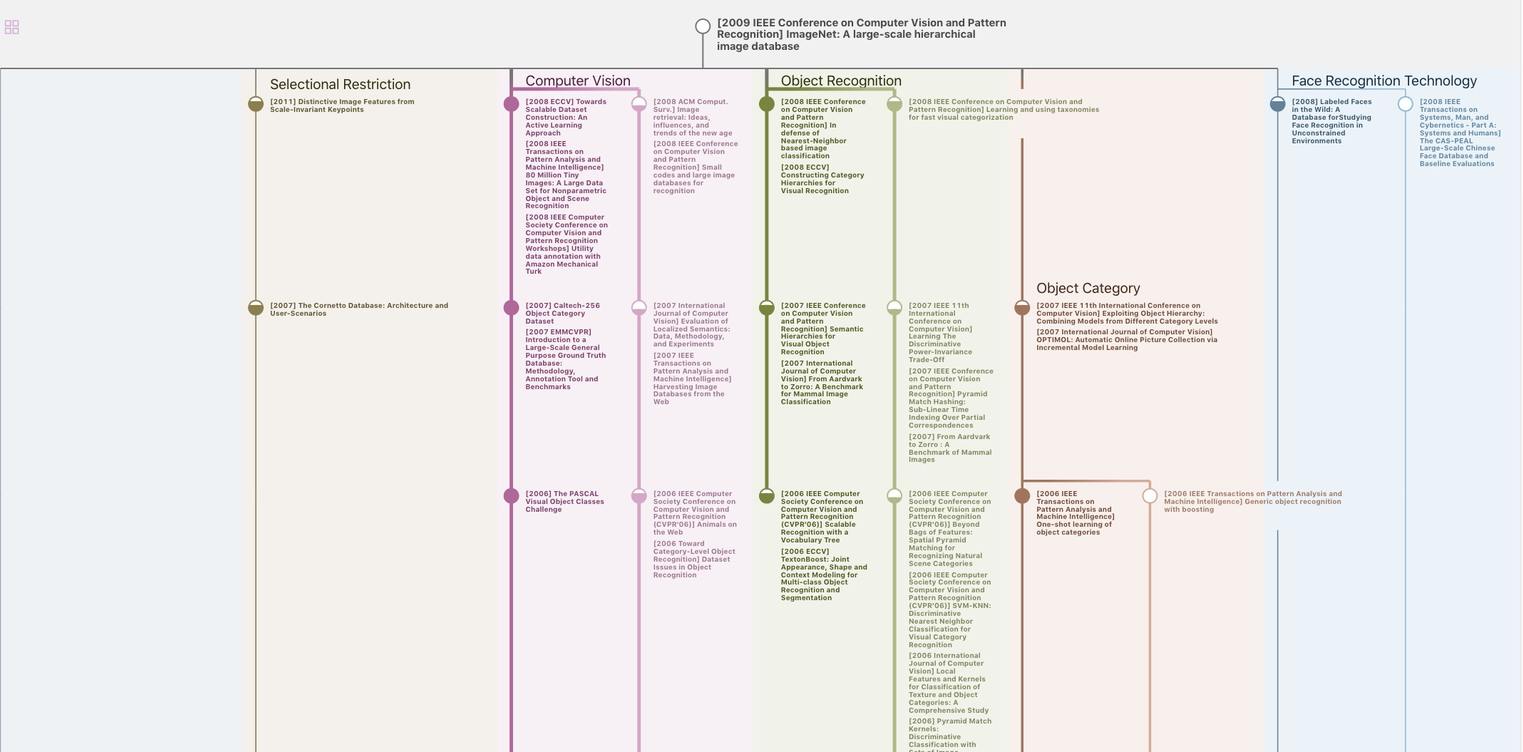
Generate MRT to find the research sequence of this paper
Chat Paper
Summary is being generated by the instructions you defined