A Diagnostic Biomarker For Breast Cancer Screening Via Hilbert Embedded Deep Low-Rank Matrix Approximation
IEEE TRANSACTIONS ON INSTRUMENTATION AND MEASUREMENT(2021)
摘要
Thermography has been used extensively as a complementary diagnostic tool in breast cancer detection. Among thermographic methods, matrix factorization (MF) techniques show an unequivocal capability to detect thermal patterns corresponding to vasodilation in the cancer cases. One of the biggest challenges in such techniques is selecting the best representation of the thermal basis. In this study, an embedding method is proposed to address this problem and deep-semi-non-negative MF (Deep-SemiNMF) for thermography is introduced, then tested for 208 breast cancer screening cases. First, we apply Deep-SemiNMF to infrared images to extract low-rank thermal representations for each case. Then, we embed low-rank bases to obtain one basis for each patient. After that, we extract 300 thermal imaging features, called thermomics, to decode imaging information for the automatic diagnostic model. We reduced the dimensionality of thermomics by spanning them on to Hilbert space using radial-based function (RBF) kernel and select the three most efficient features using the block Hilbert-Schmidt Independence Criterion (HSIC) Lasso. The preserved thermal heterogeneity successfully classified asymptomatic versus symptomatic patients applying a random forest model [cross-validated accuracy of 71.36% (69.42%-73.3%)].
更多查看译文
关键词
Breast cancer detection, deep matrix factorization (MF), Hilbert space, thermal heterogeneity, thermomics
AI 理解论文
溯源树
样例
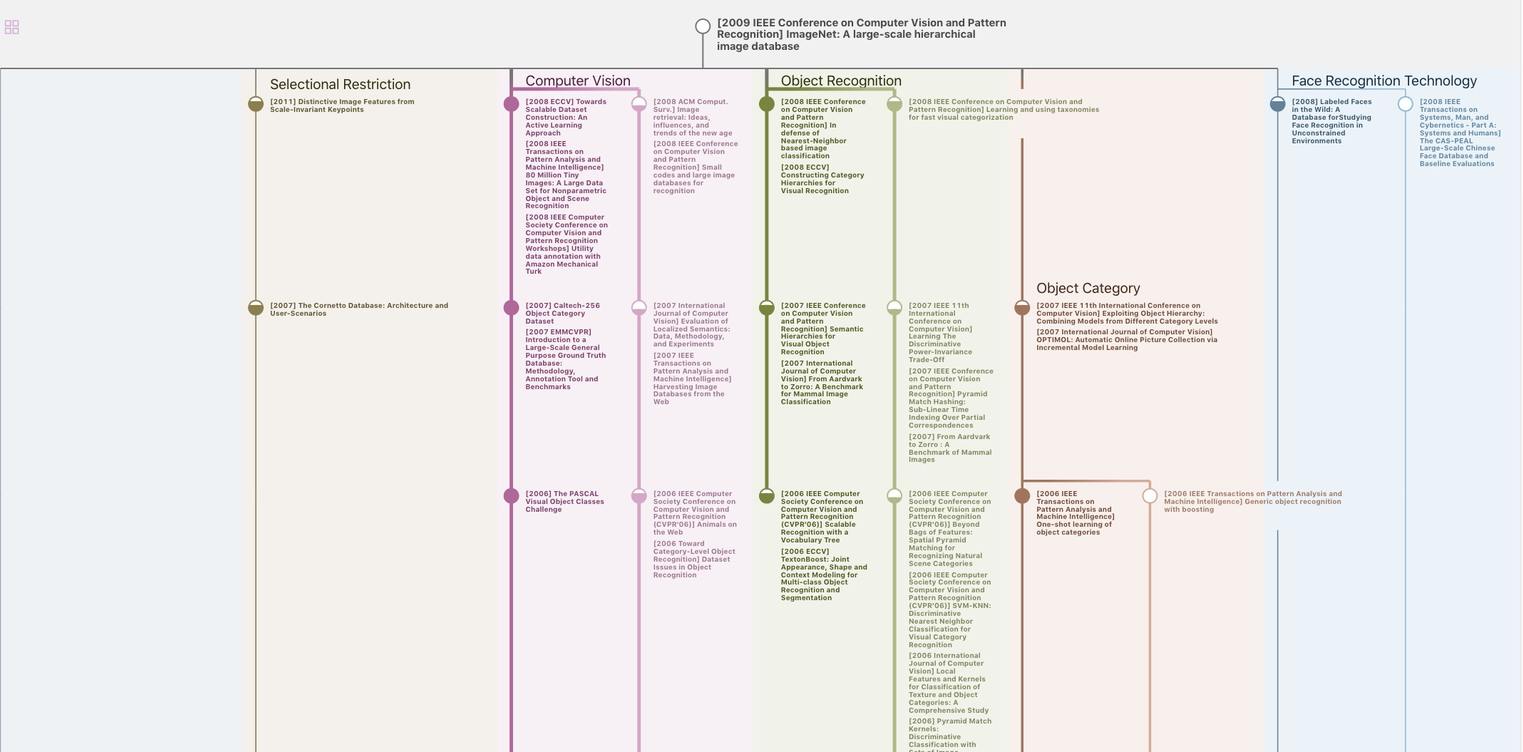
生成溯源树,研究论文发展脉络
Chat Paper
正在生成论文摘要